Gradient Boosting¶
Le notebook explore l’algorithme du Gradient Boosting.
[5]:
%matplotlib inline
Premier exemple¶
On considère les paramètres par défaut de la classe GradientBoostingRegressor.
[6]:
from numpy.random import randn, random
from pandas import DataFrame
from sklearn.model_selection import train_test_split
rnd = randn(1000)
X = random(1000) * 8 - 4
y = X**2 - X + rnd * 2 + 150 # X^2 - X + 150 + epsilon
X = X.reshape((-1, 1))
X_train, X_test, y_train, y_test = train_test_split(X, y)
df = DataFrame({"X": X_train.ravel(), "y": y_train})
ax = df.plot(x="X", y="y", kind="scatter")
ax.set_title("Nuage de points X^2 - X + 150 + epsilon");
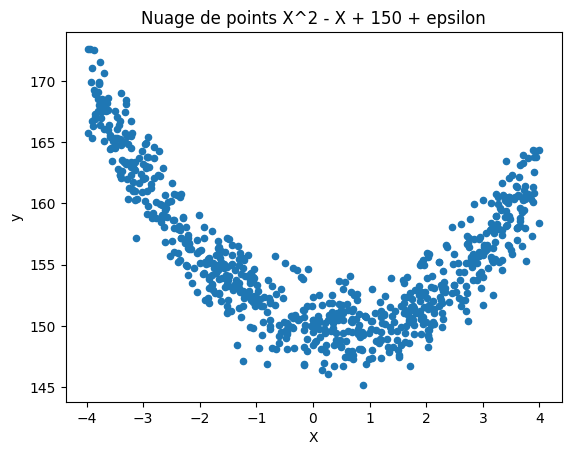
[7]:
from sklearn.ensemble import GradientBoostingRegressor
model = GradientBoostingRegressor(max_depth=1)
model.fit(X_train, y_train)
[7]:
GradientBoostingRegressor(max_depth=1)In a Jupyter environment, please rerun this cell to show the HTML representation or trust the notebook.
On GitHub, the HTML representation is unable to render, please try loading this page with nbviewer.org.
GradientBoostingRegressor(max_depth=1)
[8]:
import numpy
ind = numpy.argsort(X_test, axis=0)
y_ = model.predict(X_test)
df = DataFrame(
{"X": X_test[ind].ravel(), "y": y_test[ind].ravel(), "y^": y_[ind].ravel()}
)
ax = df.plot(x="X", y="y", kind="scatter")
df.plot(x="X", y="y^", kind="line", ax=ax, color="r")
ax.set_title("Prédictions avec GradientBoostingRegressor");
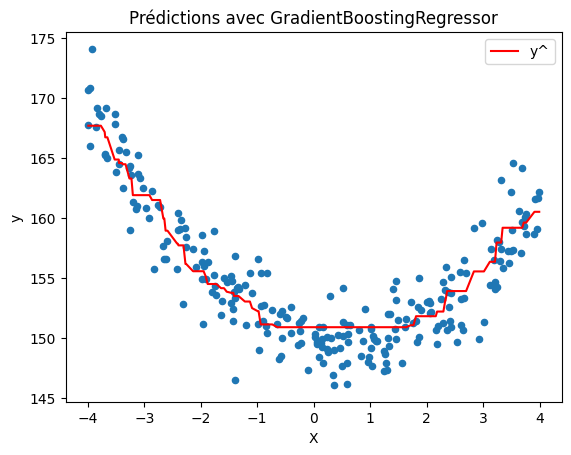
Rien d’imprévu jusque là. Essayons autre chose. On regarde avec une seule itération.
[9]:
model = GradientBoostingRegressor(max_depth=1, n_estimators=1, learning_rate=0.5)
model.fit(X_train, y_train)
[9]:
GradientBoostingRegressor(learning_rate=0.5, max_depth=1, n_estimators=1)In a Jupyter environment, please rerun this cell to show the HTML representation or trust the notebook.
On GitHub, the HTML representation is unable to render, please try loading this page with nbviewer.org.
GradientBoostingRegressor(learning_rate=0.5, max_depth=1, n_estimators=1)
[10]:
y_ = model.predict(X_test)
df = DataFrame(
{"X": X_test[ind].ravel(), "y": y_test[ind].ravel(), "y^": y_[ind].ravel()}
)
ax = df.plot(x="X", y="y", kind="scatter")
df.plot(x="X", y="y^", kind="line", ax=ax, color="r")
ax.set_title("Prédictions avec GradientBoostingRegressor\net une fonction en escalier");
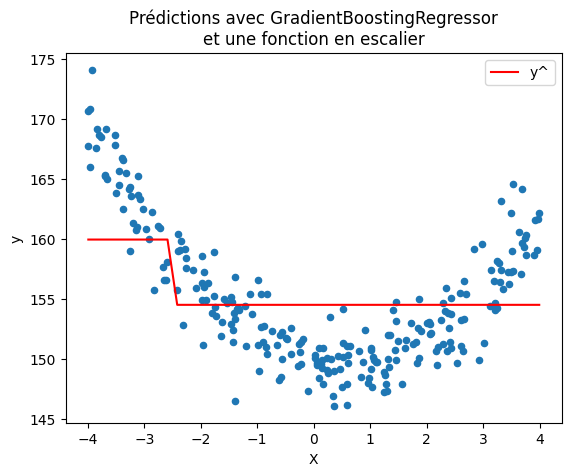
Essayons de montrer l’évolution de la courbe prédite en fonction du nombre de marches et revenons à 100 estimateurs.
[11]:
model = GradientBoostingRegressor(max_depth=1)
model.fit(X_train, y_train)
[11]:
GradientBoostingRegressor(max_depth=1)In a Jupyter environment, please rerun this cell to show the HTML representation or trust the notebook.
On GitHub, the HTML representation is unable to render, please try loading this page with nbviewer.org.
GradientBoostingRegressor(max_depth=1)
[12]:
for i in range(0, model.estimators_.shape[0] + 1, 10):
if i == 0:
df = DataFrame({"X": X_test[ind].ravel(), "y": y_test[ind].ravel()})
ax = df.plot(x="X", y="y", kind="scatter", figsize=(10, 4))
y_ = model.init_.predict(X_test)
color = "b"
else:
y_ = sum(
[
model.init_.predict(X_test),
*[
model.estimators_[k, 0].predict(X_test) * model.learning_rate
for k in range(0, i)
],
]
)
color = "r"
df = DataFrame({"X": X_test[ind].ravel(), "y^": y_[ind].ravel()})
df.plot(x="X", y="y^", kind="line", ax=ax, color=color, label="i=%d" % i)
ax.set_title("Prédictions");
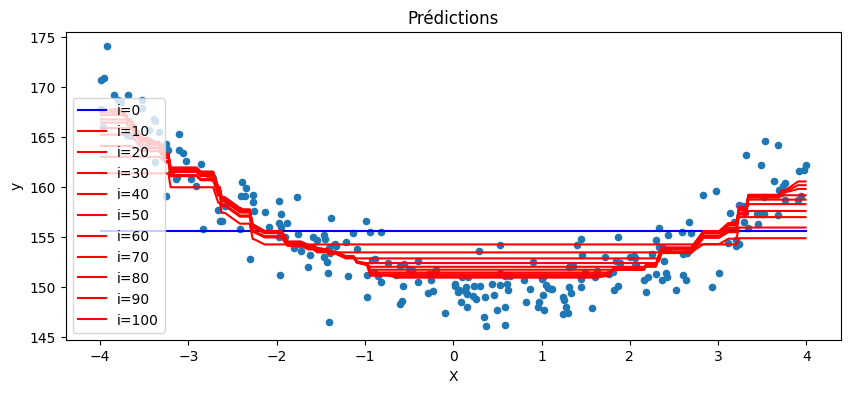
learning rate et itérations¶
Et si on choisissait un learning_rate, plus petit ou plus grand…
[13]:
model01 = GradientBoostingRegressor(max_depth=1, learning_rate=0.01)
model01.fit(X_train, y_train)
modela = GradientBoostingRegressor(max_depth=1, learning_rate=1.2)
modela.fit(X_train, y_train)
modelb = GradientBoostingRegressor(max_depth=1, learning_rate=1.99)
modelb.fit(X_train, y_train)
[13]:
GradientBoostingRegressor(learning_rate=1.99, max_depth=1)In a Jupyter environment, please rerun this cell to show the HTML representation or trust the notebook.
On GitHub, the HTML representation is unable to render, please try loading this page with nbviewer.org.
GradientBoostingRegressor(learning_rate=1.99, max_depth=1)
[14]:
import matplotlib.pyplot as plt
fig, ax = plt.subplots(1, 3, figsize=(12, 4))
ind = numpy.argsort(X_test, axis=0)
for i, mod in enumerate([model01, modela, modelb]):
df = DataFrame(
{
"X": X_test[ind].ravel(),
"y": y_test[ind].ravel(),
"y^": mod.predict(X_test)[ind].ravel(),
}
)
df.plot(x="X", y="y", kind="scatter", ax=ax[i])
df.plot(x="X", y="y^", kind="line", ax=ax[i], color="r")
ax[i].set_title("learning_rate=%f" % mod.learning_rate);
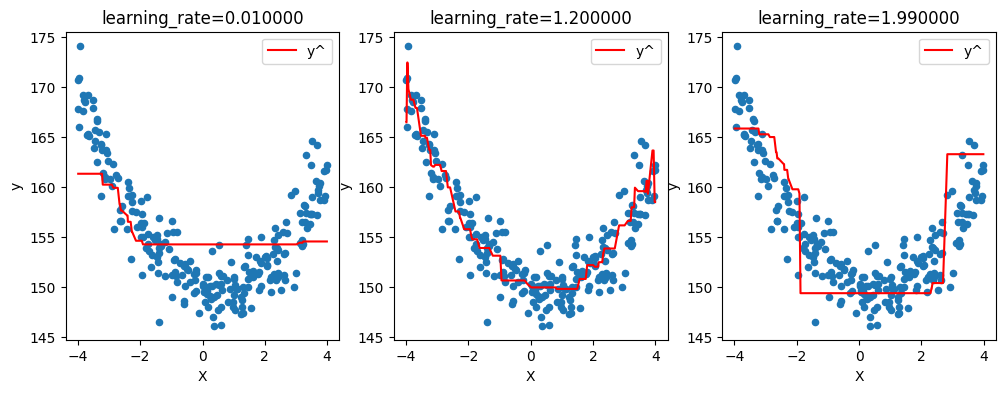
Une trop faible valeur de learning_rate semble retenir le modèle de converger, une grande valeur produit des effets imprévisibles. Pour comprendre pourquoi, il faut détailler l’algorithme…
L’algorithme¶
Inspiration¶
L’algorithme est inspiré de l’algorithme de la descente de gradient. On considère une fonction réelle et on calcule le gradient
pour construire une suite :
La suite converge vers le minimum de la fonction
. On applique cela à une fonction d’erreur issue d’un problème de régression.
Le plus souvent, on applique cette méthode à une fonction qui dépend d’un paramètre
Et c’est la suite qui converge vers le minimum de la fonction
de sorte que la fonction
approxime au mieux les points
. Mais, on pourrait tout-à-fait résoudre ce problème dans un espace de fonctions et non un espace de paramètres :
Le gradient est facile à calculer puisqu’il ne dépend pas de
. On pourrait donc construire la fonction de régression
comme une suite additive de fonctions
.
Et nous pourrions construire la fonction comme solution d’un problème de régression défini par les couples
avec :
Voilà l’idée.
Algorithme¶
Je reprends ici la page wikipedia. On cherche à construire un modèle qui minimise l’erreur . On note
le learning rate.
Etape 1 : on cale un premier modèle de régression, ici, simplement une constante, en optimisant .
est une constante.
On note ensuite où
est la fonction constante construire lors de la première étape.
Etape 2 : on calcule ensuite les erreurs et l’opposé du gradient
Etape 3 : on choisit la fonction de telle sorte qu’elle approxime au mieux les résidus
.
Etape 4 : on choisit le coefficient de telle sorte qu’il minimise l’expression
.
On retourne l’étape 2 autant de fois qu’il y a d’itérations. Lorsque l’erreur est une erreur quadratique , les résidus deviennent
. Par conséquent, la fonction
approxime au mieux ce qu’il manque pour atteindre l’objectif. Un learning rate égal à 1 fait que la somme des prédictions de chaque fonction
oscille autour de la vraie valeur, une faible valeur donne l’impression d’une fonction qui converge à petits
pas, une grande valeur accroît l’amplitude des oscillations au point d’empêcher l’algorithme de converger.
On voit aussi que l’algorithme s’intéresse d’abord aux points où le gradient est le plus fort, donc en principe aux erreurs les plus grandes.
Régression quantile¶
Dans ce cas, l’erreur quadratique est remplacée par une erreur en valeur absolue. Les résidus dans ce cas sont égaux à -1 ou 1.
[15]:
alpha = 0.5
model = GradientBoostingRegressor(
alpha=alpha, loss="quantile", max_depth=1, learning_rate=0.1
)
model.fit(X_train, y_train)
model01 = GradientBoostingRegressor(
alpha=alpha, loss="quantile", max_depth=1, learning_rate=0.01
)
model01.fit(X_train, y_train)
modela = GradientBoostingRegressor(
alpha=alpha, loss="quantile", max_depth=1, learning_rate=1.2
)
modela.fit(X_train, y_train)
modelb = GradientBoostingRegressor(
alpha=alpha, loss="quantile", max_depth=1, learning_rate=1.99
)
modelb.fit(X_train, y_train)
modelc = GradientBoostingRegressor(
alpha=alpha, loss="quantile", max_depth=1, learning_rate=2.01
)
modelc.fit(X_train, y_train)
[15]:
GradientBoostingRegressor(alpha=0.5, learning_rate=2.01, loss='quantile', max_depth=1)In a Jupyter environment, please rerun this cell to show the HTML representation or trust the notebook.
On GitHub, the HTML representation is unable to render, please try loading this page with nbviewer.org.
GradientBoostingRegressor(alpha=0.5, learning_rate=2.01, loss='quantile', max_depth=1)
[16]:
fig, ax = plt.subplots(1, 5, figsize=(12, 4))
ind = numpy.argsort(X_test, axis=0)
for i, mod in enumerate([model, model01, modela, modelb, modelc]):
df = DataFrame(
{
"X": X_test[ind].ravel(),
"y": y_test[ind].ravel(),
"y^": mod.predict(X_test)[ind].ravel(),
}
)
df.plot(x="X", y="y", kind="scatter", ax=ax[i])
df.plot(x="X", y="y^", kind="line", ax=ax[i], color="r")
ax[i].set_title("learning_rate=%1.2f" % mod.learning_rate);
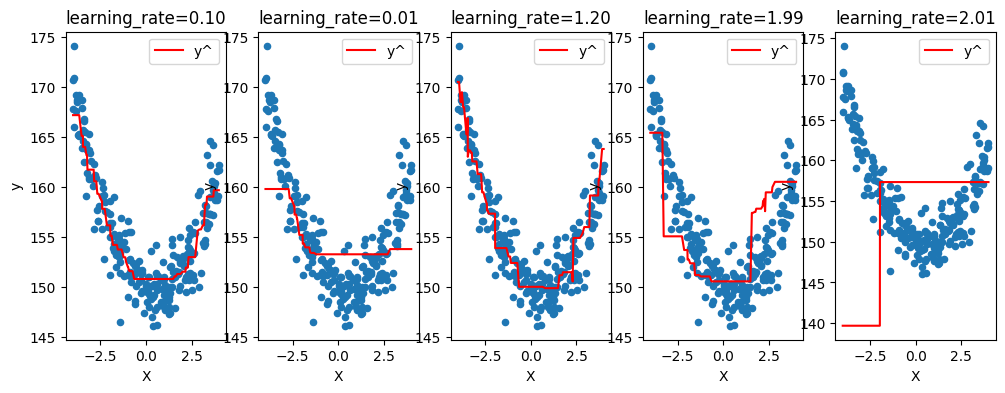
Concrètement, le paramètre max_depth=1 correspond à une simple fonction et le modèle final est une somme pondérée de fonctions indicatrices.
learning_rate et sur-apprentissage¶
[41]:
from sklearn.ensemble import RandomForestRegressor
from tqdm import tqdm
def experiment(models, tries=25):
scores = []
for _ in tqdm(range(tries)):
rnd = randn(1000)
X = random(1000) * 8 - 4
y = X**2 - X + rnd * 2 + 150 # X^2 - X + 150 + epsilon
X = X.reshape((-1, 1))
X_train, X_test, y_train, y_test = train_test_split(X, y)
scs = []
for model in models:
model.fit(X_train, y_train)
sc = model.score(X_test, y_test)
scs.append(sc)
scores.append(scs)
return scores
scores = experiment(
[
GradientBoostingRegressor(max_depth=1, n_estimators=20),
RandomForestRegressor(max_depth=1, n_estimators=20),
]
)
scores[:3]
100%|██████████| 25/25 [00:01<00:00, 15.47it/s]
[41]:
[[0.6061998151266149, 0.5241640887093781],
[0.6865162453017377, 0.5868617679417452],
[0.6643588529036899, 0.5882688129311655]]
[42]:
fig, ax = plt.subplots(1, 1, figsize=(10, 4))
ax.plot([_[0] for _ in scores], label="GradientBoostingRegressor")
ax.plot([_[1] for _ in scores], label="RandomForestRegressor")
ax.set_title("Comparaison pour une somme pondérée de fonctions en escalier")
ax.legend();
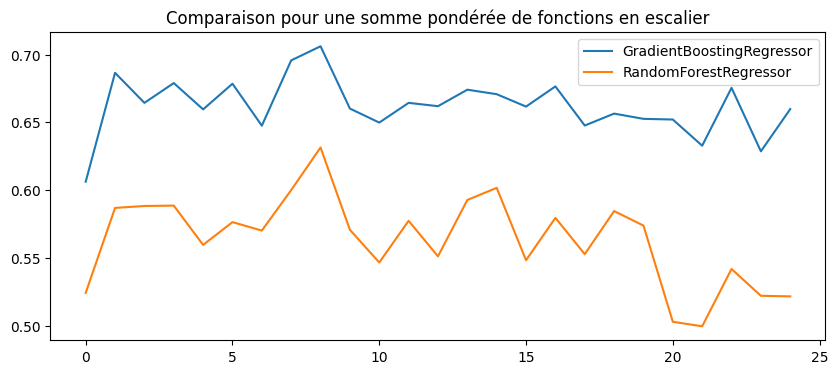
Ce résultat est attendu car la forêt aléatoire est une moyenne de modèle de régression tous appris dans les mêmes conditions alors que le gradient boosting s’intéresse à l’erreur après la somme des premiers régresseurs. Voyons avec des arbres de décision et non plus des fonctions en escaliers.
[43]:
from sklearn.tree import DecisionTreeRegressor
scores = experiment(
[
GradientBoostingRegressor(max_depth=5, n_estimators=20),
RandomForestRegressor(max_depth=5, n_estimators=20),
DecisionTreeRegressor(max_depth=5),
]
)
100%|██████████| 25/25 [00:02<00:00, 11.19it/s]
[44]:
fig, ax = plt.subplots(1, 1, figsize=(10, 4))
ax.plot([_[0] for _ in scores], label="GradientBoostingRegressor")
ax.plot([_[1] for _ in scores], label="RandomForestRegressor")
ax.plot([_[2] for _ in scores], label="DecisionTreeRegressor")
ax.set_title("Comparaison pour une somme pondérée d'arbres de décisions")
ax.legend();
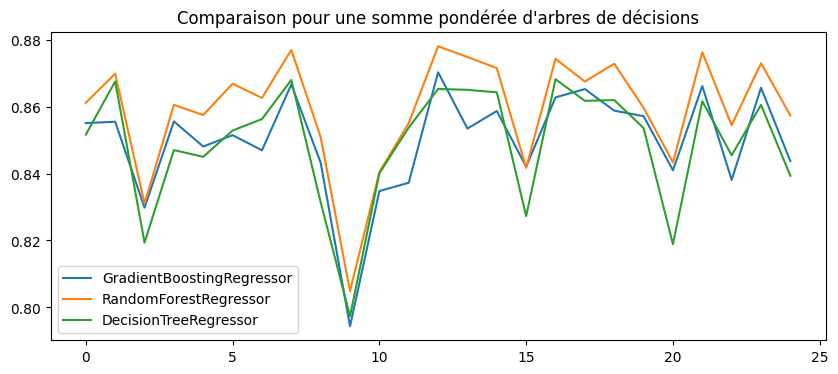
Le modèle GradientBoostingRegressor est clairement moins bon quand le modèle sous-jacent - l’arbre de décision - est performant. On voit que la forêt aléatoire est meilleure qu’un arbre de décision seul. Cela signifie qu’elle généralise mieux et que l’arbre de décision fait du sur apprentissage. De même, le GradientBoostingRegressor est plus exposé au sur-apprentissage.
[47]:
scores = experiment(
[
RandomForestRegressor(max_depth=5, n_estimators=20),
GradientBoostingRegressor(max_depth=5, n_estimators=20, learning_rate=0.05),
GradientBoostingRegressor(max_depth=5, n_estimators=20, learning_rate=0.1),
GradientBoostingRegressor(max_depth=5, n_estimators=20, learning_rate=0.2),
]
)
scores[:2]
100%|██████████| 25/25 [00:03<00:00, 6.40it/s]
[47]:
[[0.8505309865561821,
0.7409584480906592,
0.8372610096704007,
0.8433616064058848],
[0.8610474948662841,
0.7463887159097458,
0.8469931884879516,
0.8553184433763386]]
[48]:
fig, ax = plt.subplots(1, 1, figsize=(10, 4))
ax.plot([_[0] for _ in scores], label="RandomForestRegressor")
ax.plot([_[1] for _ in scores], label="GBR(5, lr=0.05)")
ax.plot([_[2] for _ in scores], label="GBR(5, lr=0.1)")
ax.plot([_[3] for _ in scores], label="GBR(5, lr=0.2)")
ax.set_title("Comparaison pour différents learning_rate")
ax.legend();
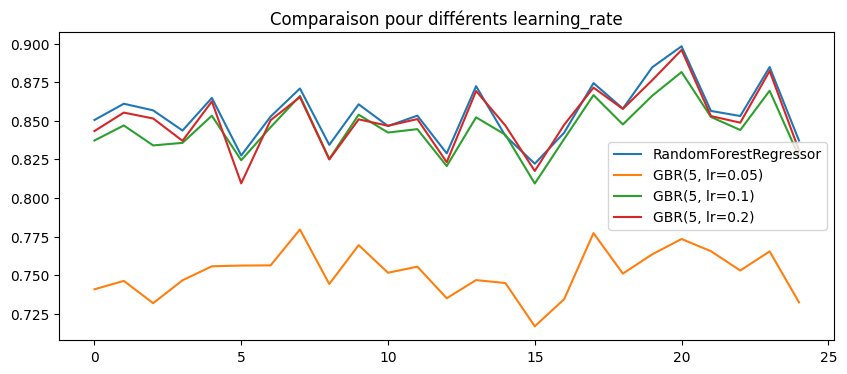
Diminuer learning_rate est clairement une façon d’éviter le sur-apprentissage mais les graphes précédents ont montré qu’il fallait plus d’itérations lorsque le learning rate est petit.
[49]:
scores = experiment(
[
RandomForestRegressor(max_depth=5, n_estimators=20),
GradientBoostingRegressor(max_depth=1, n_estimators=20, learning_rate=0.05),
GradientBoostingRegressor(max_depth=1, n_estimators=20, learning_rate=0.1),
GradientBoostingRegressor(max_depth=1, n_estimators=20, learning_rate=0.2),
]
)
scores[:2]
100%|██████████| 25/25 [00:02<00:00, 8.43it/s]
[49]:
[[0.8595510594071162,
0.47103349192372335,
0.6460977028277105,
0.7953273631823825],
[0.8418307915852581,
0.4894523688410177,
0.6494948729040753,
0.7754015641398699]]
[50]:
fig, ax = plt.subplots(1, 1, figsize=(10, 4))
ax.plot([_[0] for _ in scores], label="RandomForestRegressor")
ax.plot([_[1] for _ in scores], label="GBR(1, lr=0.05)")
ax.plot([_[2] for _ in scores], label="GBR(1, lr=0.1)")
ax.plot([_[3] for _ in scores], label="GBR(1, lr=0.2)")
ax.set_title("Comparaison pour différents learning_rate et des fonctions en escalier")
ax.legend();
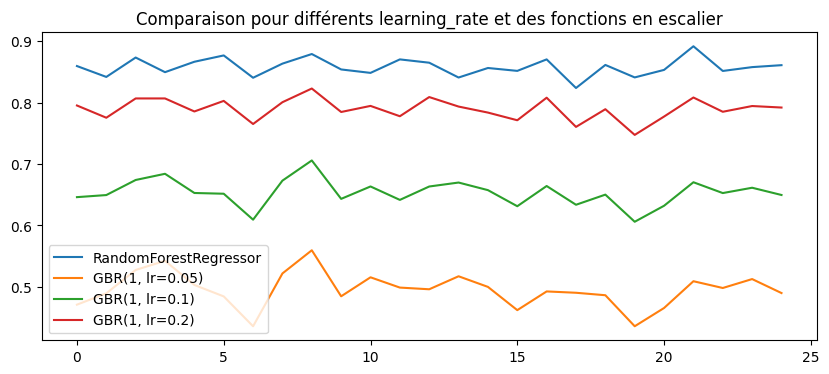
Plus le modèle sous-jacent est simple, plus le learning_rate peut être élevé car les modèles simples ne font pas de sur-apprentissage.
Gradient Boosting avec d’autres librairies¶
Une somme pondérée de régression linéaire reste une regréssion linéaire. Il est impossible de tester ce scénario avec scikit-learn puisque seuls les arbres de décisions sont implémentés. Mais il existe d’autres librairies qui implémente le gradient boosting.
XGBoost¶
[51]:
from xgboost import XGBRegressor
[52]:
scores = experiment(
[
RandomForestRegressor(max_depth=5, n_estimators=20),
XGBRegressor(
max_depth=1,
n_estimators=20,
learning_rate=0.05,
objective="reg:squarederror",
),
XGBRegressor(
max_depth=1,
n_estimators=20,
learning_rate=0.1,
objective="reg:squarederror",
),
XGBRegressor(
max_depth=1,
n_estimators=20,
learning_rate=0.2,
objective="reg:squarederror",
),
]
)
scores[:2]
100%|██████████| 25/25 [00:02<00:00, 9.09it/s]
[52]:
[[0.8362392926836479,
0.5284643193472292,
0.6696397339277518,
0.7786586369744302],
[0.8866993680319293,
0.5135892626305283,
0.6803157400434685,
0.8171169617436257]]
[53]:
fig, ax = plt.subplots(1, 1, figsize=(10, 4))
ax.plot([_[0] for _ in scores], label="RandomForestRegressor(5)")
ax.plot([_[1] for _ in scores], label="XGB(1, lr=0.05)")
ax.plot([_[2] for _ in scores], label="XGB(1, lr=0.1)")
ax.plot([_[3] for _ in scores], label="XGB(1, lr=0.2)")
ax.set_title(
"Comparaison pour différents learning_rate\net des fonctions en escalier "
"avec XGBoost"
)
ax.legend();
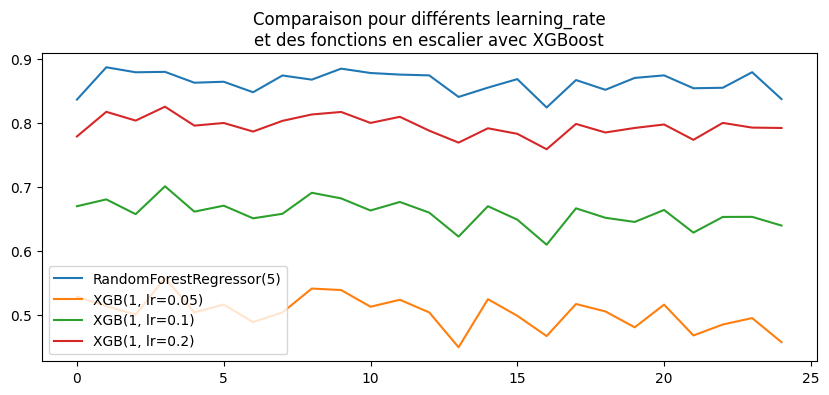
Les résultats sont sensiblement les mêmes.
[54]:
scores = experiment(
[
RandomForestRegressor(max_depth=5, n_estimators=20),
XGBRegressor(
max_depth=5,
n_estimators=20,
learning_rate=0.05,
objective="reg:squarederror",
),
XGBRegressor(
max_depth=5,
n_estimators=20,
learning_rate=0.1,
objective="reg:squarederror",
),
XGBRegressor(
max_depth=5,
n_estimators=20,
learning_rate=0.2,
objective="reg:squarederror",
),
]
)
scores[:2]
0%| | 0/25 [00:00<?, ?it/s]100%|██████████| 25/25 [00:03<00:00, 7.37it/s]
[54]:
[[0.8590132304552919,
0.7402716733771284,
0.8482110599066995,
0.8642576634357626],
[0.8599672573344654,
0.7367047036233907,
0.84659652680362,
0.8606710003826832]]
[55]:
fig, ax = plt.subplots(1, 1, figsize=(10, 4))
ax.plot([_[0] for _ in scores], label="RandomForestRegressor(5)")
ax.plot([_[1] for _ in scores], label="XGB(5, lr=0.05)")
ax.plot([_[2] for _ in scores], label="XGB(5, lr=0.1)")
ax.plot([_[3] for _ in scores], label="XGB(5, lr=0.2)")
ax.set_title(
"Comparaison pour différents learning_rate\net des arbres de décisions "
"avec XGBoost"
)
ax.legend();
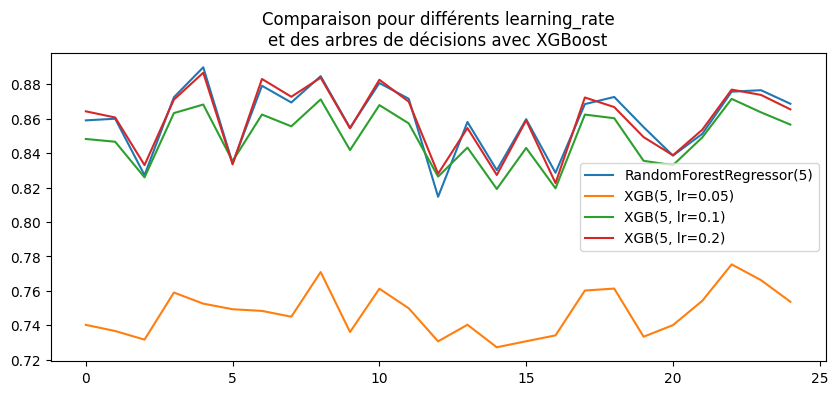
LightGbm¶
[30]:
from lightgbm import LGBMRegressor
[56]:
scores = experiment(
[
RandomForestRegressor(max_depth=5, n_estimators=20),
LGBMRegressor(max_depth=1, n_estimators=20, learning_rate=0.05),
LGBMRegressor(max_depth=1, n_estimators=20, learning_rate=0.1),
LGBMRegressor(max_depth=1, n_estimators=20, learning_rate=0.2),
]
)
scores[:2]
8%|▊ | 2/25 [00:00<00:02, 10.71it/s]
[LightGBM] [Info] Auto-choosing row-wise multi-threading, the overhead of testing was 0.000073 seconds.
You can set `force_row_wise=true` to remove the overhead.
And if memory is not enough, you can set `force_col_wise=true`.
[LightGBM] [Info] Total Bins 250
[LightGBM] [Info] Number of data points in the train set: 750, number of used features: 1
[LightGBM] [Info] Start training from score 155.276912
[LightGBM] [Info] Auto-choosing col-wise multi-threading, the overhead of testing was 0.000094 seconds.
You can set `force_col_wise=true` to remove the overhead.
[LightGBM] [Info] Total Bins 250
[LightGBM] [Info] Number of data points in the train set: 750, number of used features: 1
[LightGBM] [Info] Start training from score 155.276912
[LightGBM] [Info] Auto-choosing col-wise multi-threading, the overhead of testing was 0.000050 seconds.
You can set `force_col_wise=true` to remove the overhead.
[LightGBM] [Info] Total Bins 250
[LightGBM] [Info] Number of data points in the train set: 750, number of used features: 1
[LightGBM] [Info] Start training from score 155.276912
[LightGBM] [Info] Auto-choosing col-wise multi-threading, the overhead of testing was 0.000069 seconds.
You can set `force_col_wise=true` to remove the overhead.
[LightGBM] [Info] Total Bins 251
[LightGBM] [Info] Number of data points in the train set: 750, number of used features: 1
[LightGBM] [Info] Start training from score 155.344856
[LightGBM] [Info] Auto-choosing col-wise multi-threading, the overhead of testing was 0.000056 seconds.
You can set `force_col_wise=true` to remove the overhead.
[LightGBM] [Info] Total Bins 251
[LightGBM] [Info] Number of data points in the train set: 750, number of used features: 1
[LightGBM] [Info] Start training from score 155.344856
[LightGBM] [Info] Auto-choosing col-wise multi-threading, the overhead of testing was 0.000046 seconds.
You can set `force_col_wise=true` to remove the overhead.
[LightGBM] [Info] Total Bins 251
[LightGBM] [Info] Number of data points in the train set: 750, number of used features: 1
[LightGBM] [Info] Start training from score 155.344856
[LightGBM] [Info] Auto-choosing col-wise multi-threading, the overhead of testing was 0.000035 seconds.
You can set `force_col_wise=true` to remove the overhead.
[LightGBM] [Info] Total Bins 250
[LightGBM] [Info] Number of data points in the train set: 750, number of used features: 1
[LightGBM] [Info] Start training from score 155.231196
[LightGBM] [Info] Auto-choosing col-wise multi-threading, the overhead of testing was 0.000032 seconds.
You can set `force_col_wise=true` to remove the overhead.
[LightGBM] [Info] Total Bins 250
[LightGBM] [Info] Number of data points in the train set: 750, number of used features: 1
[LightGBM] [Info] Start training from score 155.231196
[LightGBM] [Info] Auto-choosing col-wise multi-threading, the overhead of testing was 0.000049 seconds.
You can set `force_col_wise=true` to remove the overhead.
[LightGBM] [Info] Total Bins 250
[LightGBM] [Info] Number of data points in the train set: 750, number of used features: 1
[LightGBM] [Info] Start training from score 155.231196
24%|██▍ | 6/25 [00:00<00:01, 12.90it/s]
[LightGBM] [Info] Auto-choosing col-wise multi-threading, the overhead of testing was 0.000035 seconds.
You can set `force_col_wise=true` to remove the overhead.
[LightGBM] [Info] Total Bins 251
[LightGBM] [Info] Number of data points in the train set: 750, number of used features: 1
[LightGBM] [Info] Start training from score 155.434819
[LightGBM] [Info] Auto-choosing col-wise multi-threading, the overhead of testing was 0.000046 seconds.
You can set `force_col_wise=true` to remove the overhead.
[LightGBM] [Info] Total Bins 251
[LightGBM] [Info] Number of data points in the train set: 750, number of used features: 1
[LightGBM] [Info] Start training from score 155.434819
[LightGBM] [Info] Auto-choosing col-wise multi-threading, the overhead of testing was 0.000044 seconds.
You can set `force_col_wise=true` to remove the overhead.
[LightGBM] [Info] Total Bins 251
[LightGBM] [Info] Number of data points in the train set: 750, number of used features: 1
[LightGBM] [Info] Start training from score 155.434819
[LightGBM] [Info] Auto-choosing col-wise multi-threading, the overhead of testing was 0.000035 seconds.
You can set `force_col_wise=true` to remove the overhead.
[LightGBM] [Info] Total Bins 251
[LightGBM] [Info] Number of data points in the train set: 750, number of used features: 1
[LightGBM] [Info] Start training from score 155.363485
[LightGBM] [Info] Auto-choosing col-wise multi-threading, the overhead of testing was 0.000045 seconds.
You can set `force_col_wise=true` to remove the overhead.
[LightGBM] [Info] Total Bins 251
[LightGBM] [Info] Number of data points in the train set: 750, number of used features: 1
[LightGBM] [Info] Start training from score 155.363485
[LightGBM] [Info] Auto-choosing col-wise multi-threading, the overhead of testing was 0.000038 seconds.
You can set `force_col_wise=true` to remove the overhead.
[LightGBM] [Info] Total Bins 251
[LightGBM] [Info] Number of data points in the train set: 750, number of used features: 1
[LightGBM] [Info] Start training from score 155.363485
[LightGBM] [Info] Auto-choosing col-wise multi-threading, the overhead of testing was 0.000042 seconds.
You can set `force_col_wise=true` to remove the overhead.
[LightGBM] [Info] Total Bins 251
[LightGBM] [Info] Number of data points in the train set: 750, number of used features: 1
[LightGBM] [Info] Start training from score 155.646337
[LightGBM] [Info] Auto-choosing col-wise multi-threading, the overhead of testing was 0.000043 seconds.
You can set `force_col_wise=true` to remove the overhead.
[LightGBM] [Info] Total Bins 251
[LightGBM] [Info] Number of data points in the train set: 750, number of used features: 1
[LightGBM] [Info] Start training from score 155.646337
[LightGBM] [Info] Auto-choosing col-wise multi-threading, the overhead of testing was 0.000042 seconds.
You can set `force_col_wise=true` to remove the overhead.
[LightGBM] [Info] Total Bins 251
[LightGBM] [Info] Number of data points in the train set: 750, number of used features: 1
[LightGBM] [Info] Start training from score 155.646337
32%|███▏ | 8/25 [00:00<00:01, 13.40it/s]
[LightGBM] [Info] Auto-choosing col-wise multi-threading, the overhead of testing was 0.000051 seconds.
You can set `force_col_wise=true` to remove the overhead.
[LightGBM] [Info] Total Bins 250
[LightGBM] [Info] Number of data points in the train set: 750, number of used features: 1
[LightGBM] [Info] Start training from score 155.458236
[LightGBM] [Info] Auto-choosing row-wise multi-threading, the overhead of testing was 0.000181 seconds.
You can set `force_row_wise=true` to remove the overhead.
And if memory is not enough, you can set `force_col_wise=true`.
[LightGBM] [Info] Total Bins 250
[LightGBM] [Info] Number of data points in the train set: 750, number of used features: 1
[LightGBM] [Info] Start training from score 155.458236
[LightGBM] [Info] Auto-choosing col-wise multi-threading, the overhead of testing was 0.000068 seconds.
You can set `force_col_wise=true` to remove the overhead.
[LightGBM] [Info] Total Bins 250
[LightGBM] [Info] Number of data points in the train set: 750, number of used features: 1
[LightGBM] [Info] Start training from score 155.458236
[LightGBM] [Info] Auto-choosing col-wise multi-threading, the overhead of testing was 0.000036 seconds.
You can set `force_col_wise=true` to remove the overhead.
[LightGBM] [Info] Total Bins 250
[LightGBM] [Info] Number of data points in the train set: 750, number of used features: 1
[LightGBM] [Info] Start training from score 155.527945
[LightGBM] [Info] Auto-choosing col-wise multi-threading, the overhead of testing was 0.000031 seconds.
You can set `force_col_wise=true` to remove the overhead.
[LightGBM] [Info] Total Bins 250
[LightGBM] [Info] Number of data points in the train set: 750, number of used features: 1
[LightGBM] [Info] Start training from score 155.527945
[LightGBM] [Info] Auto-choosing col-wise multi-threading, the overhead of testing was 0.000034 seconds.
You can set `force_col_wise=true` to remove the overhead.
[LightGBM] [Info] Total Bins 250
[LightGBM] [Info] Number of data points in the train set: 750, number of used features: 1
[LightGBM] [Info] Start training from score 155.527945
[LightGBM] [Info] Auto-choosing col-wise multi-threading, the overhead of testing was 0.000043 seconds.
You can set `force_col_wise=true` to remove the overhead.
[LightGBM] [Info] Total Bins 251
[LightGBM] [Info] Number of data points in the train set: 750, number of used features: 1
[LightGBM] [Info] Start training from score 155.110538
[LightGBM] [Info] Auto-choosing col-wise multi-threading, the overhead of testing was 0.000043 seconds.
You can set `force_col_wise=true` to remove the overhead.
[LightGBM] [Info] Total Bins 251
[LightGBM] [Info] Number of data points in the train set: 750, number of used features: 1
[LightGBM] [Info] Start training from score 155.110538
[LightGBM] [Info] Auto-choosing col-wise multi-threading, the overhead of testing was 0.000041 seconds.
You can set `force_col_wise=true` to remove the overhead.
[LightGBM] [Info] Total Bins 251
[LightGBM] [Info] Number of data points in the train set: 750, number of used features: 1
[LightGBM] [Info] Start training from score 155.110538
48%|████▊ | 12/25 [00:00<00:01, 12.90it/s]
[LightGBM] [Info] Auto-choosing col-wise multi-threading, the overhead of testing was 0.000054 seconds.
You can set `force_col_wise=true` to remove the overhead.
[LightGBM] [Info] Total Bins 251
[LightGBM] [Info] Number of data points in the train set: 750, number of used features: 1
[LightGBM] [Info] Start training from score 155.536138
[LightGBM] [Info] Auto-choosing row-wise multi-threading, the overhead of testing was 0.000080 seconds.
You can set `force_row_wise=true` to remove the overhead.
And if memory is not enough, you can set `force_col_wise=true`.
[LightGBM] [Info] Total Bins 251
[LightGBM] [Info] Number of data points in the train set: 750, number of used features: 1
[LightGBM] [Info] Start training from score 155.536138
[LightGBM] [Info] Auto-choosing col-wise multi-threading, the overhead of testing was 0.000038 seconds.
You can set `force_col_wise=true` to remove the overhead.
[LightGBM] [Info] Total Bins 251
[LightGBM] [Info] Number of data points in the train set: 750, number of used features: 1
[LightGBM] [Info] Start training from score 155.536138
[LightGBM] [Info] Auto-choosing col-wise multi-threading, the overhead of testing was 0.000044 seconds.
You can set `force_col_wise=true` to remove the overhead.
[LightGBM] [Info] Total Bins 251
[LightGBM] [Info] Number of data points in the train set: 750, number of used features: 1
[LightGBM] [Info] Start training from score 155.140867
[LightGBM] [Info] Auto-choosing col-wise multi-threading, the overhead of testing was 0.000060 seconds.
You can set `force_col_wise=true` to remove the overhead.
[LightGBM] [Info] Total Bins 251
[LightGBM] [Info] Number of data points in the train set: 750, number of used features: 1
[LightGBM] [Info] Start training from score 155.140867
[LightGBM] [Info] Auto-choosing row-wise multi-threading, the overhead of testing was 0.000049 seconds.
You can set `force_row_wise=true` to remove the overhead.
And if memory is not enough, you can set `force_col_wise=true`.
[LightGBM] [Info] Total Bins 251
[LightGBM] [Info] Number of data points in the train set: 750, number of used features: 1
[LightGBM] [Info] Start training from score 155.140867
[LightGBM] [Info] Auto-choosing col-wise multi-threading, the overhead of testing was 0.000056 seconds.
You can set `force_col_wise=true` to remove the overhead.
[LightGBM] [Info] Total Bins 250
[LightGBM] [Info] Number of data points in the train set: 750, number of used features: 1
[LightGBM] [Info] Start training from score 155.416133
[LightGBM] [Info] Auto-choosing col-wise multi-threading, the overhead of testing was 0.000053 seconds.
You can set `force_col_wise=true` to remove the overhead.
[LightGBM] [Info] Total Bins 250
[LightGBM] [Info] Number of data points in the train set: 750, number of used features: 1
[LightGBM] [Info] Start training from score 155.416133
[LightGBM] [Info] Auto-choosing col-wise multi-threading, the overhead of testing was 0.000069 seconds.
You can set `force_col_wise=true` to remove the overhead.
[LightGBM] [Info] Total Bins 250
[LightGBM] [Info] Number of data points in the train set: 750, number of used features: 1
[LightGBM] [Info] Start training from score 155.416133
56%|█████▌ | 14/25 [00:01<00:00, 11.40it/s]
[LightGBM] [Info] Auto-choosing col-wise multi-threading, the overhead of testing was 0.000055 seconds.
You can set `force_col_wise=true` to remove the overhead.
[LightGBM] [Info] Total Bins 250
[LightGBM] [Info] Number of data points in the train set: 750, number of used features: 1
[LightGBM] [Info] Start training from score 155.396002
[LightGBM] [Info] Auto-choosing col-wise multi-threading, the overhead of testing was 0.000284 seconds.
You can set `force_col_wise=true` to remove the overhead.
[LightGBM] [Info] Total Bins 250
[LightGBM] [Info] Number of data points in the train set: 750, number of used features: 1
[LightGBM] [Info] Start training from score 155.396002
[LightGBM] [Info] Auto-choosing col-wise multi-threading, the overhead of testing was 0.000067 seconds.
You can set `force_col_wise=true` to remove the overhead.
[LightGBM] [Info] Total Bins 250
[LightGBM] [Info] Number of data points in the train set: 750, number of used features: 1
[LightGBM] [Info] Start training from score 155.396002
[LightGBM] [Info] Auto-choosing col-wise multi-threading, the overhead of testing was 0.000056 seconds.
You can set `force_col_wise=true` to remove the overhead.
[LightGBM] [Info] Total Bins 251
[LightGBM] [Info] Number of data points in the train set: 750, number of used features: 1
[LightGBM] [Info] Start training from score 155.328942
[LightGBM] [Info] Auto-choosing col-wise multi-threading, the overhead of testing was 0.000050 seconds.
You can set `force_col_wise=true` to remove the overhead.
[LightGBM] [Info] Total Bins 251
[LightGBM] [Info] Number of data points in the train set: 750, number of used features: 1
[LightGBM] [Info] Start training from score 155.328942
[LightGBM] [Info] Auto-choosing col-wise multi-threading, the overhead of testing was 0.000064 seconds.
You can set `force_col_wise=true` to remove the overhead.
[LightGBM] [Info] Total Bins 251
[LightGBM] [Info] Number of data points in the train set: 750, number of used features: 1
[LightGBM] [Info] Start training from score 155.328942
[LightGBM] [Info] Auto-choosing col-wise multi-threading, the overhead of testing was 0.000041 seconds.
You can set `force_col_wise=true` to remove the overhead.
[LightGBM] [Info] Total Bins 250
[LightGBM] [Info] Number of data points in the train set: 750, number of used features: 1
[LightGBM] [Info] Start training from score 155.560493
[LightGBM] [Info] Auto-choosing col-wise multi-threading, the overhead of testing was 0.000076 seconds.
You can set `force_col_wise=true` to remove the overhead.
[LightGBM] [Info] Total Bins 250
[LightGBM] [Info] Number of data points in the train set: 750, number of used features: 1
[LightGBM] [Info] Start training from score 155.560493
[LightGBM] [Info] Auto-choosing col-wise multi-threading, the overhead of testing was 0.000034 seconds.
You can set `force_col_wise=true` to remove the overhead.
[LightGBM] [Info] Total Bins 250
[LightGBM] [Info] Number of data points in the train set: 750, number of used features: 1
[LightGBM] [Info] Start training from score 155.560493
72%|███████▏ | 18/25 [00:01<00:00, 12.66it/s]
[LightGBM] [Info] Auto-choosing col-wise multi-threading, the overhead of testing was 0.000047 seconds.
You can set `force_col_wise=true` to remove the overhead.
[LightGBM] [Info] Total Bins 250
[LightGBM] [Info] Number of data points in the train set: 750, number of used features: 1
[LightGBM] [Info] Start training from score 154.999049
[LightGBM] [Info] Auto-choosing col-wise multi-threading, the overhead of testing was 0.000036 seconds.
You can set `force_col_wise=true` to remove the overhead.
[LightGBM] [Info] Total Bins 250
[LightGBM] [Info] Number of data points in the train set: 750, number of used features: 1
[LightGBM] [Info] Start training from score 154.999049
[LightGBM] [Info] Auto-choosing col-wise multi-threading, the overhead of testing was 0.000035 seconds.
You can set `force_col_wise=true` to remove the overhead.
[LightGBM] [Info] Total Bins 250
[LightGBM] [Info] Number of data points in the train set: 750, number of used features: 1
[LightGBM] [Info] Start training from score 154.999049
[LightGBM] [Info] Auto-choosing col-wise multi-threading, the overhead of testing was 0.000042 seconds.
You can set `force_col_wise=true` to remove the overhead.
[LightGBM] [Info] Total Bins 251
[LightGBM] [Info] Number of data points in the train set: 750, number of used features: 1
[LightGBM] [Info] Start training from score 154.935543
[LightGBM] [Info] Auto-choosing col-wise multi-threading, the overhead of testing was 0.000037 seconds.
You can set `force_col_wise=true` to remove the overhead.
[LightGBM] [Info] Total Bins 251
[LightGBM] [Info] Number of data points in the train set: 750, number of used features: 1
[LightGBM] [Info] Start training from score 154.935543
[LightGBM] [Info] Auto-choosing col-wise multi-threading, the overhead of testing was 0.000042 seconds.
You can set `force_col_wise=true` to remove the overhead.
[LightGBM] [Info] Total Bins 251
[LightGBM] [Info] Number of data points in the train set: 750, number of used features: 1
[LightGBM] [Info] Start training from score 154.935543
[LightGBM] [Info] Auto-choosing col-wise multi-threading, the overhead of testing was 0.000136 seconds.
You can set `force_col_wise=true` to remove the overhead.
[LightGBM] [Info] Total Bins 250
[LightGBM] [Info] Number of data points in the train set: 750, number of used features: 1
[LightGBM] [Info] Start training from score 155.395022
[LightGBM] [Info] Auto-choosing col-wise multi-threading, the overhead of testing was 0.000035 seconds.
You can set `force_col_wise=true` to remove the overhead.
[LightGBM] [Info] Total Bins 250
[LightGBM] [Info] Number of data points in the train set: 750, number of used features: 1
[LightGBM] [Info] Start training from score 155.395022
[LightGBM] [Info] Auto-choosing col-wise multi-threading, the overhead of testing was 0.000031 seconds.
You can set `force_col_wise=true` to remove the overhead.
[LightGBM] [Info] Total Bins 250
[LightGBM] [Info] Number of data points in the train set: 750, number of used features: 1
[LightGBM] [Info] Start training from score 155.395022
[LightGBM] [Info] Auto-choosing col-wise multi-threading, the overhead of testing was 0.000034 seconds.
You can set `force_col_wise=true` to remove the overhead.
[LightGBM] [Info] Total Bins 250
[LightGBM] [Info] Number of data points in the train set: 750, number of used features: 1
[LightGBM] [Info] Start training from score 155.189666
80%|████████ | 20/25 [00:01<00:00, 12.98it/s]
[LightGBM] [Info] Auto-choosing row-wise multi-threading, the overhead of testing was 0.000305 seconds.
You can set `force_row_wise=true` to remove the overhead.
And if memory is not enough, you can set `force_col_wise=true`.
[LightGBM] [Info] Total Bins 250
[LightGBM] [Info] Number of data points in the train set: 750, number of used features: 1
[LightGBM] [Info] Start training from score 155.189666
[LightGBM] [Info] Auto-choosing col-wise multi-threading, the overhead of testing was 0.000033 seconds.
You can set `force_col_wise=true` to remove the overhead.
[LightGBM] [Info] Total Bins 250
[LightGBM] [Info] Number of data points in the train set: 750, number of used features: 1
[LightGBM] [Info] Start training from score 155.189666
[LightGBM] [Info] Auto-choosing col-wise multi-threading, the overhead of testing was 0.000040 seconds.
You can set `force_col_wise=true` to remove the overhead.
[LightGBM] [Info] Total Bins 250
[LightGBM] [Info] Number of data points in the train set: 750, number of used features: 1
[LightGBM] [Info] Start training from score 155.055646
[LightGBM] [Info] Auto-choosing col-wise multi-threading, the overhead of testing was 0.000039 seconds.
You can set `force_col_wise=true` to remove the overhead.
[LightGBM] [Info] Total Bins 250
[LightGBM] [Info] Number of data points in the train set: 750, number of used features: 1
[LightGBM] [Info] Start training from score 155.055646
[LightGBM] [Info] Auto-choosing col-wise multi-threading, the overhead of testing was 0.000048 seconds.
You can set `force_col_wise=true` to remove the overhead.
[LightGBM] [Info] Total Bins 250
[LightGBM] [Info] Number of data points in the train set: 750, number of used features: 1
[LightGBM] [Info] Start training from score 155.055646
[LightGBM] [Info] Auto-choosing col-wise multi-threading, the overhead of testing was 0.000041 seconds.
You can set `force_col_wise=true` to remove the overhead.
[LightGBM] [Info] Total Bins 250
[LightGBM] [Info] Number of data points in the train set: 750, number of used features: 1
[LightGBM] [Info] Start training from score 155.380804
[LightGBM] [Info] Auto-choosing col-wise multi-threading, the overhead of testing was 0.000046 seconds.
You can set `force_col_wise=true` to remove the overhead.
[LightGBM] [Info] Total Bins 250
[LightGBM] [Info] Number of data points in the train set: 750, number of used features: 1
[LightGBM] [Info] Start training from score 155.380804
[LightGBM] [Info] Auto-choosing col-wise multi-threading, the overhead of testing was 0.000046 seconds.
You can set `force_col_wise=true` to remove the overhead.
[LightGBM] [Info] Total Bins 250
[LightGBM] [Info] Number of data points in the train set: 750, number of used features: 1
[LightGBM] [Info] Start training from score 155.380804
[LightGBM] [Info] Auto-choosing col-wise multi-threading, the overhead of testing was 0.000042 seconds.
You can set `force_col_wise=true` to remove the overhead.
[LightGBM] [Info] Total Bins 250
[LightGBM] [Info] Number of data points in the train set: 750, number of used features: 1
[LightGBM] [Info] Start training from score 155.240350
96%|█████████▌| 24/25 [00:01<00:00, 13.63it/s]
[LightGBM] [Info] Auto-choosing col-wise multi-threading, the overhead of testing was 0.000042 seconds.
You can set `force_col_wise=true` to remove the overhead.
[LightGBM] [Info] Total Bins 250
[LightGBM] [Info] Number of data points in the train set: 750, number of used features: 1
[LightGBM] [Info] Start training from score 155.240350
[LightGBM] [Info] Auto-choosing row-wise multi-threading, the overhead of testing was 0.000577 seconds.
You can set `force_row_wise=true` to remove the overhead.
And if memory is not enough, you can set `force_col_wise=true`.
[LightGBM] [Info] Total Bins 250
[LightGBM] [Info] Number of data points in the train set: 750, number of used features: 1
[LightGBM] [Info] Start training from score 155.240350
[LightGBM] [Info] Auto-choosing col-wise multi-threading, the overhead of testing was 0.000042 seconds.
You can set `force_col_wise=true` to remove the overhead.
[LightGBM] [Info] Total Bins 250
[LightGBM] [Info] Number of data points in the train set: 750, number of used features: 1
[LightGBM] [Info] Start training from score 155.005265
[LightGBM] [Info] Auto-choosing col-wise multi-threading, the overhead of testing was 0.000034 seconds.
You can set `force_col_wise=true` to remove the overhead.
[LightGBM] [Info] Total Bins 250
[LightGBM] [Info] Number of data points in the train set: 750, number of used features: 1
[LightGBM] [Info] Start training from score 155.005265
[LightGBM] [Info] Auto-choosing col-wise multi-threading, the overhead of testing was 0.000049 seconds.
You can set `force_col_wise=true` to remove the overhead.
[LightGBM] [Info] Total Bins 250
[LightGBM] [Info] Number of data points in the train set: 750, number of used features: 1
[LightGBM] [Info] Start training from score 155.005265
[LightGBM] [Info] Auto-choosing col-wise multi-threading, the overhead of testing was 0.000042 seconds.
You can set `force_col_wise=true` to remove the overhead.
[LightGBM] [Info] Total Bins 250
[LightGBM] [Info] Number of data points in the train set: 750, number of used features: 1
[LightGBM] [Info] Start training from score 155.258938
[LightGBM] [Info] Auto-choosing col-wise multi-threading, the overhead of testing was 0.000037 seconds.
You can set `force_col_wise=true` to remove the overhead.
[LightGBM] [Info] Total Bins 250
[LightGBM] [Info] Number of data points in the train set: 750, number of used features: 1
[LightGBM] [Info] Start training from score 155.258938
[LightGBM] [Info] Auto-choosing col-wise multi-threading, the overhead of testing was 0.000039 seconds.
You can set `force_col_wise=true` to remove the overhead.
[LightGBM] [Info] Total Bins 250
[LightGBM] [Info] Number of data points in the train set: 750, number of used features: 1
[LightGBM] [Info] Start training from score 155.258938
100%|██████████| 25/25 [00:01<00:00, 12.91it/s]
[LightGBM] [Info] Auto-choosing col-wise multi-threading, the overhead of testing was 0.000035 seconds.
You can set `force_col_wise=true` to remove the overhead.
[LightGBM] [Info] Total Bins 250
[LightGBM] [Info] Number of data points in the train set: 750, number of used features: 1
[LightGBM] [Info] Start training from score 155.386138
[LightGBM] [Info] Auto-choosing col-wise multi-threading, the overhead of testing was 0.000049 seconds.
You can set `force_col_wise=true` to remove the overhead.
[LightGBM] [Info] Total Bins 250
[LightGBM] [Info] Number of data points in the train set: 750, number of used features: 1
[LightGBM] [Info] Start training from score 155.386138
[LightGBM] [Info] Auto-choosing col-wise multi-threading, the overhead of testing was 0.000035 seconds.
You can set `force_col_wise=true` to remove the overhead.
[LightGBM] [Info] Total Bins 250
[LightGBM] [Info] Number of data points in the train set: 750, number of used features: 1
[LightGBM] [Info] Start training from score 155.386138
[56]:
[[0.8773032128441518,
0.48214820701023675,
0.6539572474605444,
0.7984627882777451],
[0.8555575315832163,
0.4977691173485087,
0.6367319006906368,
0.7683900978469463]]
[57]:
fig, ax = plt.subplots(1, 1, figsize=(10, 4))
ax.plot([_[0] for _ in scores], label="RandomForestRegressor(5)")
ax.plot([_[1] for _ in scores], label="LGB(1, lr=0.05)")
ax.plot([_[2] for _ in scores], label="LGB(1, lr=0.1)")
ax.plot([_[3] for _ in scores], label="LGB(1, lr=0.2)")
ax.set_title(
"Comparaison pour différents learning_rate\net des fonctions en escalier "
"avec LightGBM"
)
ax.legend();
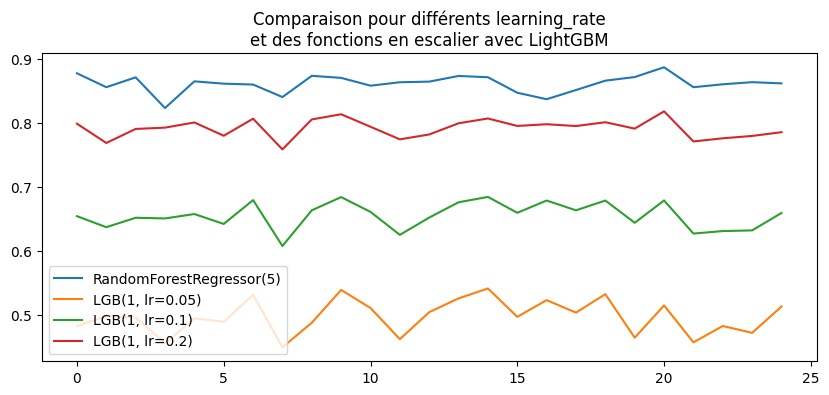
[58]:
scores = experiment(
[
RandomForestRegressor(max_depth=5, n_estimators=20),
LGBMRegressor(max_depth=5, n_estimators=20, learning_rate=0.05),
LGBMRegressor(max_depth=5, n_estimators=20, learning_rate=0.1),
LGBMRegressor(max_depth=5, n_estimators=20, learning_rate=0.2),
]
)
scores[:2]
8%|▊ | 2/25 [00:00<00:02, 8.95it/s]
[LightGBM] [Warning] Accuracy may be bad since you didn't explicitly set num_leaves OR 2^max_depth > num_leaves. (num_leaves=31).
[LightGBM] [Warning] Accuracy may be bad since you didn't explicitly set num_leaves OR 2^max_depth > num_leaves. (num_leaves=31).
[LightGBM] [Info] Auto-choosing col-wise multi-threading, the overhead of testing was 0.000063 seconds.
You can set `force_col_wise=true` to remove the overhead.
[LightGBM] [Info] Total Bins 250
[LightGBM] [Info] Number of data points in the train set: 750, number of used features: 1
[LightGBM] [Info] Start training from score 155.156120
[LightGBM] [Warning] No further splits with positive gain, best gain: -inf
[LightGBM] [Warning] No further splits with positive gain, best gain: -inf
[LightGBM] [Warning] No further splits with positive gain, best gain: -inf
[LightGBM] [Warning] No further splits with positive gain, best gain: -inf
[LightGBM] [Warning] No further splits with positive gain, best gain: -inf
[LightGBM] [Warning] No further splits with positive gain, best gain: -inf
[LightGBM] [Warning] No further splits with positive gain, best gain: -inf
[LightGBM] [Warning] No further splits with positive gain, best gain: -inf
[LightGBM] [Warning] No further splits with positive gain, best gain: -inf
[LightGBM] [Warning] No further splits with positive gain, best gain: -inf
[LightGBM] [Warning] No further splits with positive gain, best gain: -inf
[LightGBM] [Warning] No further splits with positive gain, best gain: -inf
[LightGBM] [Warning] No further splits with positive gain, best gain: -inf
[LightGBM] [Warning] No further splits with positive gain, best gain: -inf
[LightGBM] [Warning] No further splits with positive gain, best gain: -inf
[LightGBM] [Warning] No further splits with positive gain, best gain: -inf
[LightGBM] [Warning] No further splits with positive gain, best gain: -inf
[LightGBM] [Warning] No further splits with positive gain, best gain: -inf
[LightGBM] [Warning] No further splits with positive gain, best gain: -inf
[LightGBM] [Warning] No further splits with positive gain, best gain: -inf
[LightGBM] [Warning] Accuracy may be bad since you didn't explicitly set num_leaves OR 2^max_depth > num_leaves. (num_leaves=31).
[LightGBM] [Warning] Accuracy may be bad since you didn't explicitly set num_leaves OR 2^max_depth > num_leaves. (num_leaves=31).
[LightGBM] [Warning] Accuracy may be bad since you didn't explicitly set num_leaves OR 2^max_depth > num_leaves. (num_leaves=31).
[LightGBM] [Info] Auto-choosing col-wise multi-threading, the overhead of testing was 0.000039 seconds.
You can set `force_col_wise=true` to remove the overhead.
[LightGBM] [Info] Total Bins 250
[LightGBM] [Info] Number of data points in the train set: 750, number of used features: 1
[LightGBM] [Info] Start training from score 155.156120
[LightGBM] [Warning] No further splits with positive gain, best gain: -inf
[LightGBM] [Warning] No further splits with positive gain, best gain: -inf
[LightGBM] [Warning] No further splits with positive gain, best gain: -inf
[LightGBM] [Warning] No further splits with positive gain, best gain: -inf
[LightGBM] [Warning] No further splits with positive gain, best gain: -inf
[LightGBM] [Warning] No further splits with positive gain, best gain: -inf
[LightGBM] [Warning] No further splits with positive gain, best gain: -inf
[LightGBM] [Warning] No further splits with positive gain, best gain: -inf
[LightGBM] [Warning] No further splits with positive gain, best gain: -inf
[LightGBM] [Warning] No further splits with positive gain, best gain: -inf
[LightGBM] [Warning] No further splits with positive gain, best gain: -inf
[LightGBM] [Warning] No further splits with positive gain, best gain: -inf
[LightGBM] [Warning] No further splits with positive gain, best gain: -inf
[LightGBM] [Warning] No further splits with positive gain, best gain: -inf
[LightGBM] [Warning] No further splits with positive gain, best gain: -inf
[LightGBM] [Warning] No further splits with positive gain, best gain: -inf
[LightGBM] [Warning] No further splits with positive gain, best gain: -inf
[LightGBM] [Warning] No further splits with positive gain, best gain: -inf
[LightGBM] [Warning] No further splits with positive gain, best gain: -inf
[LightGBM] [Warning] No further splits with positive gain, best gain: -inf
[LightGBM] [Warning] Accuracy may be bad since you didn't explicitly set num_leaves OR 2^max_depth > num_leaves. (num_leaves=31).
[LightGBM] [Warning] Accuracy may be bad since you didn't explicitly set num_leaves OR 2^max_depth > num_leaves. (num_leaves=31).
[LightGBM] [Warning] Accuracy may be bad since you didn't explicitly set num_leaves OR 2^max_depth > num_leaves. (num_leaves=31).
[LightGBM] [Info] Auto-choosing col-wise multi-threading, the overhead of testing was 0.000067 seconds.
You can set `force_col_wise=true` to remove the overhead.
[LightGBM] [Info] Total Bins 250
[LightGBM] [Info] Number of data points in the train set: 750, number of used features: 1
[LightGBM] [Info] Start training from score 155.156120
[LightGBM] [Warning] No further splits with positive gain, best gain: -inf
[LightGBM] [Warning] No further splits with positive gain, best gain: -inf
[LightGBM] [Warning] No further splits with positive gain, best gain: -inf
[LightGBM] [Warning] No further splits with positive gain, best gain: -inf
[LightGBM] [Warning] No further splits with positive gain, best gain: -inf
[LightGBM] [Warning] No further splits with positive gain, best gain: -inf
[LightGBM] [Warning] No further splits with positive gain, best gain: -inf
[LightGBM] [Warning] No further splits with positive gain, best gain: -inf
[LightGBM] [Warning] No further splits with positive gain, best gain: -inf
[LightGBM] [Warning] No further splits with positive gain, best gain: -inf
[LightGBM] [Warning] No further splits with positive gain, best gain: -inf
[LightGBM] [Warning] No further splits with positive gain, best gain: -inf
[LightGBM] [Warning] No further splits with positive gain, best gain: -inf
[LightGBM] [Warning] No further splits with positive gain, best gain: -inf
[LightGBM] [Warning] No further splits with positive gain, best gain: -inf
[LightGBM] [Warning] No further splits with positive gain, best gain: -inf
[LightGBM] [Warning] No further splits with positive gain, best gain: -inf
[LightGBM] [Warning] No further splits with positive gain, best gain: -inf
[LightGBM] [Warning] No further splits with positive gain, best gain: -inf
[LightGBM] [Warning] No further splits with positive gain, best gain: -inf
[LightGBM] [Warning] Accuracy may be bad since you didn't explicitly set num_leaves OR 2^max_depth > num_leaves. (num_leaves=31).
[LightGBM] [Warning] Accuracy may be bad since you didn't explicitly set num_leaves OR 2^max_depth > num_leaves. (num_leaves=31).
[LightGBM] [Warning] Accuracy may be bad since you didn't explicitly set num_leaves OR 2^max_depth > num_leaves. (num_leaves=31).
[LightGBM] [Info] Auto-choosing col-wise multi-threading, the overhead of testing was 0.000049 seconds.
You can set `force_col_wise=true` to remove the overhead.
[LightGBM] [Info] Total Bins 250
[LightGBM] [Info] Number of data points in the train set: 750, number of used features: 1
[LightGBM] [Info] Start training from score 155.182360
[LightGBM] [Warning] No further splits with positive gain, best gain: -inf
[LightGBM] [Warning] No further splits with positive gain, best gain: -inf
[LightGBM] [Warning] No further splits with positive gain, best gain: -inf
[LightGBM] [Warning] No further splits with positive gain, best gain: -inf
[LightGBM] [Warning] No further splits with positive gain, best gain: -inf
[LightGBM] [Warning] No further splits with positive gain, best gain: -inf
[LightGBM] [Warning] No further splits with positive gain, best gain: -inf
[LightGBM] [Warning] No further splits with positive gain, best gain: -inf
[LightGBM] [Warning] No further splits with positive gain, best gain: -inf
[LightGBM] [Warning] No further splits with positive gain, best gain: -inf
[LightGBM] [Warning] No further splits with positive gain, best gain: -inf
[LightGBM] [Warning] No further splits with positive gain, best gain: -inf
[LightGBM] [Warning] No further splits with positive gain, best gain: -inf
[LightGBM] [Warning] No further splits with positive gain, best gain: -inf
[LightGBM] [Warning] No further splits with positive gain, best gain: -inf
[LightGBM] [Warning] No further splits with positive gain, best gain: -inf
[LightGBM] [Warning] No further splits with positive gain, best gain: -inf
[LightGBM] [Warning] No further splits with positive gain, best gain: -inf
[LightGBM] [Warning] No further splits with positive gain, best gain: -inf
[LightGBM] [Warning] No further splits with positive gain, best gain: -inf
[LightGBM] [Warning] Accuracy may be bad since you didn't explicitly set num_leaves OR 2^max_depth > num_leaves. (num_leaves=31).
[LightGBM] [Warning] Accuracy may be bad since you didn't explicitly set num_leaves OR 2^max_depth > num_leaves. (num_leaves=31).
[LightGBM] [Warning] Accuracy may be bad since you didn't explicitly set num_leaves OR 2^max_depth > num_leaves. (num_leaves=31).
[LightGBM] [Info] Auto-choosing col-wise multi-threading, the overhead of testing was 0.000048 seconds.
You can set `force_col_wise=true` to remove the overhead.
[LightGBM] [Info] Total Bins 250
[LightGBM] [Info] Number of data points in the train set: 750, number of used features: 1
[LightGBM] [Info] Start training from score 155.182360
[LightGBM] [Warning] No further splits with positive gain, best gain: -inf
[LightGBM] [Warning] No further splits with positive gain, best gain: -inf
[LightGBM] [Warning] No further splits with positive gain, best gain: -inf
[LightGBM] [Warning] No further splits with positive gain, best gain: -inf
[LightGBM] [Warning] No further splits with positive gain, best gain: -inf
[LightGBM] [Warning] No further splits with positive gain, best gain: -inf
[LightGBM] [Warning] No further splits with positive gain, best gain: -inf
[LightGBM] [Warning] No further splits with positive gain, best gain: -inf
[LightGBM] [Warning] No further splits with positive gain, best gain: -inf
[LightGBM] [Warning] No further splits with positive gain, best gain: -inf
[LightGBM] [Warning] No further splits with positive gain, best gain: -inf
[LightGBM] [Warning] No further splits with positive gain, best gain: -inf
[LightGBM] [Warning] No further splits with positive gain, best gain: -inf
[LightGBM] [Warning] No further splits with positive gain, best gain: -inf
[LightGBM] [Warning] No further splits with positive gain, best gain: -inf
[LightGBM] [Warning] No further splits with positive gain, best gain: -inf
[LightGBM] [Warning] No further splits with positive gain, best gain: -inf
[LightGBM] [Warning] No further splits with positive gain, best gain: -inf
[LightGBM] [Warning] No further splits with positive gain, best gain: -inf
[LightGBM] [Warning] No further splits with positive gain, best gain: -inf
[LightGBM] [Warning] Accuracy may be bad since you didn't explicitly set num_leaves OR 2^max_depth > num_leaves. (num_leaves=31).
[LightGBM] [Warning] Accuracy may be bad since you didn't explicitly set num_leaves OR 2^max_depth > num_leaves. (num_leaves=31).
[LightGBM] [Warning] Accuracy may be bad since you didn't explicitly set num_leaves OR 2^max_depth > num_leaves. (num_leaves=31).
[LightGBM] [Info] Auto-choosing col-wise multi-threading, the overhead of testing was 0.000039 seconds.
You can set `force_col_wise=true` to remove the overhead.
[LightGBM] [Info] Total Bins 250
[LightGBM] [Info] Number of data points in the train set: 750, number of used features: 1
[LightGBM] [Info] Start training from score 155.182360
[LightGBM] [Warning] No further splits with positive gain, best gain: -inf
[LightGBM] [Warning] No further splits with positive gain, best gain: -inf
[LightGBM] [Warning] No further splits with positive gain, best gain: -inf
[LightGBM] [Warning] No further splits with positive gain, best gain: -inf
[LightGBM] [Warning] No further splits with positive gain, best gain: -inf
[LightGBM] [Warning] No further splits with positive gain, best gain: -inf
[LightGBM] [Warning] No further splits with positive gain, best gain: -inf
[LightGBM] [Warning] No further splits with positive gain, best gain: -inf
[LightGBM] [Warning] No further splits with positive gain, best gain: -inf
[LightGBM] [Warning] No further splits with positive gain, best gain: -inf
[LightGBM] [Warning] No further splits with positive gain, best gain: -inf
[LightGBM] [Warning] No further splits with positive gain, best gain: -inf
[LightGBM] [Warning] No further splits with positive gain, best gain: -inf
[LightGBM] [Warning] No further splits with positive gain, best gain: -inf
[LightGBM] [Warning] No further splits with positive gain, best gain: -inf
[LightGBM] [Warning] No further splits with positive gain, best gain: -inf
[LightGBM] [Warning] No further splits with positive gain, best gain: -inf
[LightGBM] [Warning] No further splits with positive gain, best gain: -inf
[LightGBM] [Warning] No further splits with positive gain, best gain: -inf
[LightGBM] [Warning] No further splits with positive gain, best gain: -inf
[LightGBM] [Warning] Accuracy may be bad since you didn't explicitly set num_leaves OR 2^max_depth > num_leaves. (num_leaves=31).
[LightGBM] [Warning] Accuracy may be bad since you didn't explicitly set num_leaves OR 2^max_depth > num_leaves. (num_leaves=31).
[LightGBM] [Warning] Accuracy may be bad since you didn't explicitly set num_leaves OR 2^max_depth > num_leaves. (num_leaves=31).
[LightGBM] [Info] Auto-choosing col-wise multi-threading, the overhead of testing was 0.000036 seconds.
You can set `force_col_wise=true` to remove the overhead.
[LightGBM] [Info] Total Bins 250
[LightGBM] [Info] Number of data points in the train set: 750, number of used features: 1
[LightGBM] [Info] Start training from score 155.345161
[LightGBM] [Warning] No further splits with positive gain, best gain: -inf
[LightGBM] [Warning] No further splits with positive gain, best gain: -inf
[LightGBM] [Warning] No further splits with positive gain, best gain: -inf
[LightGBM] [Warning] No further splits with positive gain, best gain: -inf
[LightGBM] [Warning] No further splits with positive gain, best gain: -inf
[LightGBM] [Warning] No further splits with positive gain, best gain: -inf
[LightGBM] [Warning] No further splits with positive gain, best gain: -inf
[LightGBM] [Warning] No further splits with positive gain, best gain: -inf
[LightGBM] [Warning] No further splits with positive gain, best gain: -inf
[LightGBM] [Warning] No further splits with positive gain, best gain: -inf
[LightGBM] [Warning] No further splits with positive gain, best gain: -inf
[LightGBM] [Warning] No further splits with positive gain, best gain: -inf
[LightGBM] [Warning] No further splits with positive gain, best gain: -inf
[LightGBM] [Warning] No further splits with positive gain, best gain: -inf
[LightGBM] [Warning] No further splits with positive gain, best gain: -inf
[LightGBM] [Warning] No further splits with positive gain, best gain: -inf
[LightGBM] [Warning] No further splits with positive gain, best gain: -inf
[LightGBM] [Warning] No further splits with positive gain, best gain: -inf
[LightGBM] [Warning] No further splits with positive gain, best gain: -inf
[LightGBM] [Warning] No further splits with positive gain, best gain: -inf
16%|█▌ | 4/25 [00:00<00:02, 10.20it/s]
[LightGBM] [Warning] Accuracy may be bad since you didn't explicitly set num_leaves OR 2^max_depth > num_leaves. (num_leaves=31).
[LightGBM] [Warning] Accuracy may be bad since you didn't explicitly set num_leaves OR 2^max_depth > num_leaves. (num_leaves=31).
[LightGBM] [Warning] Accuracy may be bad since you didn't explicitly set num_leaves OR 2^max_depth > num_leaves. (num_leaves=31).
[LightGBM] [Info] Auto-choosing col-wise multi-threading, the overhead of testing was 0.000038 seconds.
You can set `force_col_wise=true` to remove the overhead.
[LightGBM] [Info] Total Bins 250
[LightGBM] [Info] Number of data points in the train set: 750, number of used features: 1
[LightGBM] [Info] Start training from score 155.345161
[LightGBM] [Warning] No further splits with positive gain, best gain: -inf
[LightGBM] [Warning] No further splits with positive gain, best gain: -inf
[LightGBM] [Warning] No further splits with positive gain, best gain: -inf
[LightGBM] [Warning] No further splits with positive gain, best gain: -inf
[LightGBM] [Warning] No further splits with positive gain, best gain: -inf
[LightGBM] [Warning] No further splits with positive gain, best gain: -inf
[LightGBM] [Warning] No further splits with positive gain, best gain: -inf
[LightGBM] [Warning] No further splits with positive gain, best gain: -inf
[LightGBM] [Warning] No further splits with positive gain, best gain: -inf
[LightGBM] [Warning] No further splits with positive gain, best gain: -inf
[LightGBM] [Warning] No further splits with positive gain, best gain: -inf
[LightGBM] [Warning] No further splits with positive gain, best gain: -inf
[LightGBM] [Warning] No further splits with positive gain, best gain: -inf
[LightGBM] [Warning] No further splits with positive gain, best gain: -inf
[LightGBM] [Warning] No further splits with positive gain, best gain: -inf
[LightGBM] [Warning] No further splits with positive gain, best gain: -inf
[LightGBM] [Warning] No further splits with positive gain, best gain: -inf
[LightGBM] [Warning] No further splits with positive gain, best gain: -inf
[LightGBM] [Warning] No further splits with positive gain, best gain: -inf
[LightGBM] [Warning] No further splits with positive gain, best gain: -inf
[LightGBM] [Warning] Accuracy may be bad since you didn't explicitly set num_leaves OR 2^max_depth > num_leaves. (num_leaves=31).
[LightGBM] [Warning] Accuracy may be bad since you didn't explicitly set num_leaves OR 2^max_depth > num_leaves. (num_leaves=31).
[LightGBM] [Warning] Accuracy may be bad since you didn't explicitly set num_leaves OR 2^max_depth > num_leaves. (num_leaves=31).
[LightGBM] [Info] Auto-choosing col-wise multi-threading, the overhead of testing was 0.000037 seconds.
You can set `force_col_wise=true` to remove the overhead.
[LightGBM] [Info] Total Bins 250
[LightGBM] [Info] Number of data points in the train set: 750, number of used features: 1
[LightGBM] [Info] Start training from score 155.345161
[LightGBM] [Warning] No further splits with positive gain, best gain: -inf
[LightGBM] [Warning] No further splits with positive gain, best gain: -inf
[LightGBM] [Warning] No further splits with positive gain, best gain: -inf
[LightGBM] [Warning] No further splits with positive gain, best gain: -inf
[LightGBM] [Warning] No further splits with positive gain, best gain: -inf
[LightGBM] [Warning] No further splits with positive gain, best gain: -inf
[LightGBM] [Warning] No further splits with positive gain, best gain: -inf
[LightGBM] [Warning] No further splits with positive gain, best gain: -inf
[LightGBM] [Warning] No further splits with positive gain, best gain: -inf
[LightGBM] [Warning] No further splits with positive gain, best gain: -inf
[LightGBM] [Warning] No further splits with positive gain, best gain: -inf
[LightGBM] [Warning] No further splits with positive gain, best gain: -inf
[LightGBM] [Warning] No further splits with positive gain, best gain: -inf
[LightGBM] [Warning] No further splits with positive gain, best gain: -inf
[LightGBM] [Warning] No further splits with positive gain, best gain: -inf
[LightGBM] [Warning] No further splits with positive gain, best gain: -inf
[LightGBM] [Warning] No further splits with positive gain, best gain: -inf
[LightGBM] [Warning] No further splits with positive gain, best gain: -inf
[LightGBM] [Warning] No further splits with positive gain, best gain: -inf
[LightGBM] [Warning] No further splits with positive gain, best gain: -inf
[LightGBM] [Warning] Accuracy may be bad since you didn't explicitly set num_leaves OR 2^max_depth > num_leaves. (num_leaves=31).
[LightGBM] [Warning] Accuracy may be bad since you didn't explicitly set num_leaves OR 2^max_depth > num_leaves. (num_leaves=31).
[LightGBM] [Warning] Accuracy may be bad since you didn't explicitly set num_leaves OR 2^max_depth > num_leaves. (num_leaves=31).
[LightGBM] [Info] Auto-choosing col-wise multi-threading, the overhead of testing was 0.000035 seconds.
You can set `force_col_wise=true` to remove the overhead.
[LightGBM] [Info] Total Bins 250
[LightGBM] [Info] Number of data points in the train set: 750, number of used features: 1
[LightGBM] [Info] Start training from score 155.125674
[LightGBM] [Warning] No further splits with positive gain, best gain: -inf
[LightGBM] [Warning] No further splits with positive gain, best gain: -inf
[LightGBM] [Warning] No further splits with positive gain, best gain: -inf
[LightGBM] [Warning] No further splits with positive gain, best gain: -inf
[LightGBM] [Warning] No further splits with positive gain, best gain: -inf
[LightGBM] [Warning] No further splits with positive gain, best gain: -inf
[LightGBM] [Warning] No further splits with positive gain, best gain: -inf
[LightGBM] [Warning] No further splits with positive gain, best gain: -inf
[LightGBM] [Warning] No further splits with positive gain, best gain: -inf
[LightGBM] [Warning] No further splits with positive gain, best gain: -inf
[LightGBM] [Warning] No further splits with positive gain, best gain: -inf
[LightGBM] [Warning] No further splits with positive gain, best gain: -inf
[LightGBM] [Warning] No further splits with positive gain, best gain: -inf
[LightGBM] [Warning] No further splits with positive gain, best gain: -inf
[LightGBM] [Warning] No further splits with positive gain, best gain: -inf
[LightGBM] [Warning] No further splits with positive gain, best gain: -inf
[LightGBM] [Warning] No further splits with positive gain, best gain: -inf
[LightGBM] [Warning] No further splits with positive gain, best gain: -inf
[LightGBM] [Warning] No further splits with positive gain, best gain: -inf
[LightGBM] [Warning] No further splits with positive gain, best gain: -inf
[LightGBM] [Warning] Accuracy may be bad since you didn't explicitly set num_leaves OR 2^max_depth > num_leaves. (num_leaves=31).
[LightGBM] [Warning] Accuracy may be bad since you didn't explicitly set num_leaves OR 2^max_depth > num_leaves. (num_leaves=31).
[LightGBM] [Warning] Accuracy may be bad since you didn't explicitly set num_leaves OR 2^max_depth > num_leaves. (num_leaves=31).
[LightGBM] [Info] Auto-choosing col-wise multi-threading, the overhead of testing was 0.000042 seconds.
You can set `force_col_wise=true` to remove the overhead.
[LightGBM] [Info] Total Bins 250
[LightGBM] [Info] Number of data points in the train set: 750, number of used features: 1
[LightGBM] [Info] Start training from score 155.125674
[LightGBM] [Warning] No further splits with positive gain, best gain: -inf
[LightGBM] [Warning] No further splits with positive gain, best gain: -inf
[LightGBM] [Warning] No further splits with positive gain, best gain: -inf
[LightGBM] [Warning] No further splits with positive gain, best gain: -inf
[LightGBM] [Warning] No further splits with positive gain, best gain: -inf
[LightGBM] [Warning] No further splits with positive gain, best gain: -inf
[LightGBM] [Warning] No further splits with positive gain, best gain: -inf
[LightGBM] [Warning] No further splits with positive gain, best gain: -inf
[LightGBM] [Warning] No further splits with positive gain, best gain: -inf
[LightGBM] [Warning] No further splits with positive gain, best gain: -inf
[LightGBM] [Warning] No further splits with positive gain, best gain: -inf
[LightGBM] [Warning] No further splits with positive gain, best gain: -inf
[LightGBM] [Warning] No further splits with positive gain, best gain: -inf
[LightGBM] [Warning] No further splits with positive gain, best gain: -inf
[LightGBM] [Warning] No further splits with positive gain, best gain: -inf
[LightGBM] [Warning] No further splits with positive gain, best gain: -inf
[LightGBM] [Warning] No further splits with positive gain, best gain: -inf
[LightGBM] [Warning] No further splits with positive gain, best gain: -inf
[LightGBM] [Warning] No further splits with positive gain, best gain: -inf
[LightGBM] [Warning] No further splits with positive gain, best gain: -inf
[LightGBM] [Warning] Accuracy may be bad since you didn't explicitly set num_leaves OR 2^max_depth > num_leaves. (num_leaves=31).
[LightGBM] [Warning] Accuracy may be bad since you didn't explicitly set num_leaves OR 2^max_depth > num_leaves. (num_leaves=31).
[LightGBM] [Warning] Accuracy may be bad since you didn't explicitly set num_leaves OR 2^max_depth > num_leaves. (num_leaves=31).
[LightGBM] [Info] Auto-choosing col-wise multi-threading, the overhead of testing was 0.000041 seconds.
You can set `force_col_wise=true` to remove the overhead.
[LightGBM] [Info] Total Bins 250
[LightGBM] [Info] Number of data points in the train set: 750, number of used features: 1
[LightGBM] [Info] Start training from score 155.125674
[LightGBM] [Warning] No further splits with positive gain, best gain: -inf
[LightGBM] [Warning] No further splits with positive gain, best gain: -inf
[LightGBM] [Warning] No further splits with positive gain, best gain: -inf
[LightGBM] [Warning] No further splits with positive gain, best gain: -inf
[LightGBM] [Warning] No further splits with positive gain, best gain: -inf
[LightGBM] [Warning] No further splits with positive gain, best gain: -inf
[LightGBM] [Warning] No further splits with positive gain, best gain: -inf
[LightGBM] [Warning] No further splits with positive gain, best gain: -inf
[LightGBM] [Warning] No further splits with positive gain, best gain: -inf
[LightGBM] [Warning] No further splits with positive gain, best gain: -inf
[LightGBM] [Warning] No further splits with positive gain, best gain: -inf
[LightGBM] [Warning] No further splits with positive gain, best gain: -inf
[LightGBM] [Warning] No further splits with positive gain, best gain: -inf
[LightGBM] [Warning] No further splits with positive gain, best gain: -inf
[LightGBM] [Warning] No further splits with positive gain, best gain: -inf
[LightGBM] [Warning] No further splits with positive gain, best gain: -inf
[LightGBM] [Warning] No further splits with positive gain, best gain: -inf
[LightGBM] [Warning] No further splits with positive gain, best gain: -inf
[LightGBM] [Warning] No further splits with positive gain, best gain: -inf
[LightGBM] [Warning] No further splits with positive gain, best gain: -inf
[LightGBM] [Warning] Accuracy may be bad since you didn't explicitly set num_leaves OR 2^max_depth > num_leaves. (num_leaves=31).
[LightGBM] [Warning] Accuracy may be bad since you didn't explicitly set num_leaves OR 2^max_depth > num_leaves. (num_leaves=31).
[LightGBM] [Warning] Accuracy may be bad since you didn't explicitly set num_leaves OR 2^max_depth > num_leaves. (num_leaves=31).
[LightGBM] [Info] Auto-choosing col-wise multi-threading, the overhead of testing was 0.000038 seconds.
You can set `force_col_wise=true` to remove the overhead.
[LightGBM] [Info] Total Bins 250
[LightGBM] [Info] Number of data points in the train set: 750, number of used features: 1
[LightGBM] [Info] Start training from score 155.173663
[LightGBM] [Warning] No further splits with positive gain, best gain: -inf
[LightGBM] [Warning] No further splits with positive gain, best gain: -inf
[LightGBM] [Warning] No further splits with positive gain, best gain: -inf
[LightGBM] [Warning] No further splits with positive gain, best gain: -inf
[LightGBM] [Warning] No further splits with positive gain, best gain: -inf
[LightGBM] [Warning] No further splits with positive gain, best gain: -inf
[LightGBM] [Warning] No further splits with positive gain, best gain: -inf
[LightGBM] [Warning] No further splits with positive gain, best gain: -inf
[LightGBM] [Warning] No further splits with positive gain, best gain: -inf
[LightGBM] [Warning] No further splits with positive gain, best gain: -inf
[LightGBM] [Warning] No further splits with positive gain, best gain: -inf
[LightGBM] [Warning] No further splits with positive gain, best gain: -inf
[LightGBM] [Warning] No further splits with positive gain, best gain: -inf
[LightGBM] [Warning] No further splits with positive gain, best gain: -inf
[LightGBM] [Warning] No further splits with positive gain, best gain: -inf
[LightGBM] [Warning] No further splits with positive gain, best gain: -inf
[LightGBM] [Warning] No further splits with positive gain, best gain: -inf
[LightGBM] [Warning] No further splits with positive gain, best gain: -inf
[LightGBM] [Warning] No further splits with positive gain, best gain: -inf
[LightGBM] [Warning] No further splits with positive gain, best gain: -inf
[LightGBM] [Warning] Accuracy may be bad since you didn't explicitly set num_leaves OR 2^max_depth > num_leaves. (num_leaves=31).
[LightGBM] [Warning] Accuracy may be bad since you didn't explicitly set num_leaves OR 2^max_depth > num_leaves. (num_leaves=31).
[LightGBM] [Warning] Accuracy may be bad since you didn't explicitly set num_leaves OR 2^max_depth > num_leaves. (num_leaves=31).
[LightGBM] [Info] Auto-choosing col-wise multi-threading, the overhead of testing was 0.000035 seconds.
You can set `force_col_wise=true` to remove the overhead.
[LightGBM] [Info] Total Bins 250
[LightGBM] [Info] Number of data points in the train set: 750, number of used features: 1
[LightGBM] [Info] Start training from score 155.173663
[LightGBM] [Warning] No further splits with positive gain, best gain: -inf
[LightGBM] [Warning] No further splits with positive gain, best gain: -inf
[LightGBM] [Warning] No further splits with positive gain, best gain: -inf
[LightGBM] [Warning] No further splits with positive gain, best gain: -inf
[LightGBM] [Warning] No further splits with positive gain, best gain: -inf
[LightGBM] [Warning] No further splits with positive gain, best gain: -inf
[LightGBM] [Warning] No further splits with positive gain, best gain: -inf
[LightGBM] [Warning] No further splits with positive gain, best gain: -inf
[LightGBM] [Warning] No further splits with positive gain, best gain: -inf
[LightGBM] [Warning] No further splits with positive gain, best gain: -inf
[LightGBM] [Warning] No further splits with positive gain, best gain: -inf
[LightGBM] [Warning] No further splits with positive gain, best gain: -inf
[LightGBM] [Warning] No further splits with positive gain, best gain: -inf
[LightGBM] [Warning] No further splits with positive gain, best gain: -inf
[LightGBM] [Warning] No further splits with positive gain, best gain: -inf
[LightGBM] [Warning] No further splits with positive gain, best gain: -inf
[LightGBM] [Warning] No further splits with positive gain, best gain: -inf
[LightGBM] [Warning] No further splits with positive gain, best gain: -inf
[LightGBM] [Warning] No further splits with positive gain, best gain: -inf
[LightGBM] [Warning] No further splits with positive gain, best gain: -inf
[LightGBM] [Warning] Accuracy may be bad since you didn't explicitly set num_leaves OR 2^max_depth > num_leaves. (num_leaves=31).
[LightGBM] [Warning] Accuracy may be bad since you didn't explicitly set num_leaves OR 2^max_depth > num_leaves. (num_leaves=31).
[LightGBM] [Warning] Accuracy may be bad since you didn't explicitly set num_leaves OR 2^max_depth > num_leaves. (num_leaves=31).
[LightGBM] [Info] Auto-choosing col-wise multi-threading, the overhead of testing was 0.000040 seconds.
You can set `force_col_wise=true` to remove the overhead.
[LightGBM] [Info] Total Bins 250
[LightGBM] [Info] Number of data points in the train set: 750, number of used features: 1
[LightGBM] [Info] Start training from score 155.173663
[LightGBM] [Warning] No further splits with positive gain, best gain: -inf
[LightGBM] [Warning] No further splits with positive gain, best gain: -inf
[LightGBM] [Warning] No further splits with positive gain, best gain: -inf
[LightGBM] [Warning] No further splits with positive gain, best gain: -inf
[LightGBM] [Warning] No further splits with positive gain, best gain: -inf
[LightGBM] [Warning] No further splits with positive gain, best gain: -inf
[LightGBM] [Warning] No further splits with positive gain, best gain: -inf
[LightGBM] [Warning] No further splits with positive gain, best gain: -inf
[LightGBM] [Warning] No further splits with positive gain, best gain: -inf
[LightGBM] [Warning] No further splits with positive gain, best gain: -inf
[LightGBM] [Warning] No further splits with positive gain, best gain: -inf
[LightGBM] [Warning] No further splits with positive gain, best gain: -inf
[LightGBM] [Warning] No further splits with positive gain, best gain: -inf
[LightGBM] [Warning] No further splits with positive gain, best gain: -inf
[LightGBM] [Warning] No further splits with positive gain, best gain: -inf
[LightGBM] [Warning] No further splits with positive gain, best gain: -inf
[LightGBM] [Warning] No further splits with positive gain, best gain: -inf
[LightGBM] [Warning] No further splits with positive gain, best gain: -inf
[LightGBM] [Warning] No further splits with positive gain, best gain: -inf
[LightGBM] [Warning] No further splits with positive gain, best gain: -inf
[LightGBM] [Warning] Accuracy may be bad since you didn't explicitly set num_leaves OR 2^max_depth > num_leaves. (num_leaves=31).
24%|██▍ | 6/25 [00:00<00:01, 10.88it/s]
[LightGBM] [Warning] Accuracy may be bad since you didn't explicitly set num_leaves OR 2^max_depth > num_leaves. (num_leaves=31).
[LightGBM] [Warning] Accuracy may be bad since you didn't explicitly set num_leaves OR 2^max_depth > num_leaves. (num_leaves=31).
[LightGBM] [Info] Auto-choosing col-wise multi-threading, the overhead of testing was 0.000057 seconds.
You can set `force_col_wise=true` to remove the overhead.
[LightGBM] [Info] Total Bins 250
[LightGBM] [Info] Number of data points in the train set: 750, number of used features: 1
[LightGBM] [Info] Start training from score 155.600185
[LightGBM] [Warning] No further splits with positive gain, best gain: -inf
[LightGBM] [Warning] No further splits with positive gain, best gain: -inf
[LightGBM] [Warning] No further splits with positive gain, best gain: -inf
[LightGBM] [Warning] No further splits with positive gain, best gain: -inf
[LightGBM] [Warning] No further splits with positive gain, best gain: -inf
[LightGBM] [Warning] No further splits with positive gain, best gain: -inf
[LightGBM] [Warning] No further splits with positive gain, best gain: -inf
[LightGBM] [Warning] No further splits with positive gain, best gain: -inf
[LightGBM] [Warning] No further splits with positive gain, best gain: -inf
[LightGBM] [Warning] No further splits with positive gain, best gain: -inf
[LightGBM] [Warning] No further splits with positive gain, best gain: -inf
[LightGBM] [Warning] No further splits with positive gain, best gain: -inf
[LightGBM] [Warning] No further splits with positive gain, best gain: -inf
[LightGBM] [Warning] No further splits with positive gain, best gain: -inf
[LightGBM] [Warning] No further splits with positive gain, best gain: -inf
[LightGBM] [Warning] No further splits with positive gain, best gain: -inf
[LightGBM] [Warning] No further splits with positive gain, best gain: -inf
[LightGBM] [Warning] No further splits with positive gain, best gain: -inf
[LightGBM] [Warning] No further splits with positive gain, best gain: -inf
[LightGBM] [Warning] No further splits with positive gain, best gain: -inf
[LightGBM] [Warning] Accuracy may be bad since you didn't explicitly set num_leaves OR 2^max_depth > num_leaves. (num_leaves=31).
[LightGBM] [Warning] Accuracy may be bad since you didn't explicitly set num_leaves OR 2^max_depth > num_leaves. (num_leaves=31).
[LightGBM] [Warning] Accuracy may be bad since you didn't explicitly set num_leaves OR 2^max_depth > num_leaves. (num_leaves=31).
[LightGBM] [Info] Auto-choosing col-wise multi-threading, the overhead of testing was 0.000048 seconds.
You can set `force_col_wise=true` to remove the overhead.
[LightGBM] [Info] Total Bins 250
[LightGBM] [Info] Number of data points in the train set: 750, number of used features: 1
[LightGBM] [Info] Start training from score 155.600185
[LightGBM] [Warning] No further splits with positive gain, best gain: -inf
[LightGBM] [Warning] No further splits with positive gain, best gain: -inf
[LightGBM] [Warning] No further splits with positive gain, best gain: -inf
[LightGBM] [Warning] No further splits with positive gain, best gain: -inf
[LightGBM] [Warning] No further splits with positive gain, best gain: -inf
[LightGBM] [Warning] No further splits with positive gain, best gain: -inf
[LightGBM] [Warning] No further splits with positive gain, best gain: -inf
[LightGBM] [Warning] No further splits with positive gain, best gain: -inf
[LightGBM] [Warning] No further splits with positive gain, best gain: -inf
[LightGBM] [Warning] No further splits with positive gain, best gain: -inf
[LightGBM] [Warning] No further splits with positive gain, best gain: -inf
[LightGBM] [Warning] No further splits with positive gain, best gain: -inf
[LightGBM] [Warning] No further splits with positive gain, best gain: -inf
[LightGBM] [Warning] No further splits with positive gain, best gain: -inf
[LightGBM] [Warning] No further splits with positive gain, best gain: -inf
[LightGBM] [Warning] No further splits with positive gain, best gain: -inf
[LightGBM] [Warning] No further splits with positive gain, best gain: -inf
[LightGBM] [Warning] No further splits with positive gain, best gain: -inf
[LightGBM] [Warning] No further splits with positive gain, best gain: -inf
[LightGBM] [Warning] No further splits with positive gain, best gain: -inf
[LightGBM] [Warning] Accuracy may be bad since you didn't explicitly set num_leaves OR 2^max_depth > num_leaves. (num_leaves=31).
[LightGBM] [Warning] Accuracy may be bad since you didn't explicitly set num_leaves OR 2^max_depth > num_leaves. (num_leaves=31).
[LightGBM] [Warning] Accuracy may be bad since you didn't explicitly set num_leaves OR 2^max_depth > num_leaves. (num_leaves=31).
[LightGBM] [Info] Auto-choosing col-wise multi-threading, the overhead of testing was 0.000047 seconds.
You can set `force_col_wise=true` to remove the overhead.
[LightGBM] [Info] Total Bins 250
[LightGBM] [Info] Number of data points in the train set: 750, number of used features: 1
[LightGBM] [Info] Start training from score 155.600185
[LightGBM] [Warning] No further splits with positive gain, best gain: -inf
[LightGBM] [Warning] No further splits with positive gain, best gain: -inf
[LightGBM] [Warning] No further splits with positive gain, best gain: -inf
[LightGBM] [Warning] No further splits with positive gain, best gain: -inf
[LightGBM] [Warning] No further splits with positive gain, best gain: -inf
[LightGBM] [Warning] No further splits with positive gain, best gain: -inf
[LightGBM] [Warning] No further splits with positive gain, best gain: -inf
[LightGBM] [Warning] No further splits with positive gain, best gain: -inf
[LightGBM] [Warning] No further splits with positive gain, best gain: -inf
[LightGBM] [Warning] No further splits with positive gain, best gain: -inf
[LightGBM] [Warning] No further splits with positive gain, best gain: -inf
[LightGBM] [Warning] No further splits with positive gain, best gain: -inf
[LightGBM] [Warning] No further splits with positive gain, best gain: -inf
[LightGBM] [Warning] No further splits with positive gain, best gain: -inf
[LightGBM] [Warning] No further splits with positive gain, best gain: -inf
[LightGBM] [Warning] No further splits with positive gain, best gain: -inf
[LightGBM] [Warning] No further splits with positive gain, best gain: -inf
[LightGBM] [Warning] No further splits with positive gain, best gain: -inf
[LightGBM] [Warning] No further splits with positive gain, best gain: -inf
[LightGBM] [Warning] No further splits with positive gain, best gain: -inf
[LightGBM] [Warning] Accuracy may be bad since you didn't explicitly set num_leaves OR 2^max_depth > num_leaves. (num_leaves=31).
[LightGBM] [Warning] Accuracy may be bad since you didn't explicitly set num_leaves OR 2^max_depth > num_leaves. (num_leaves=31).
[LightGBM] [Warning] Accuracy may be bad since you didn't explicitly set num_leaves OR 2^max_depth > num_leaves. (num_leaves=31).
[LightGBM] [Info] Auto-choosing col-wise multi-threading, the overhead of testing was 0.000039 seconds.
You can set `force_col_wise=true` to remove the overhead.
[LightGBM] [Info] Total Bins 250
[LightGBM] [Info] Number of data points in the train set: 750, number of used features: 1
[LightGBM] [Info] Start training from score 155.499798
[LightGBM] [Warning] No further splits with positive gain, best gain: -inf
[LightGBM] [Warning] No further splits with positive gain, best gain: -inf
[LightGBM] [Warning] No further splits with positive gain, best gain: -inf
[LightGBM] [Warning] No further splits with positive gain, best gain: -inf
[LightGBM] [Warning] No further splits with positive gain, best gain: -inf
[LightGBM] [Warning] No further splits with positive gain, best gain: -inf
[LightGBM] [Warning] No further splits with positive gain, best gain: -inf
[LightGBM] [Warning] No further splits with positive gain, best gain: -inf
[LightGBM] [Warning] No further splits with positive gain, best gain: -inf
[LightGBM] [Warning] No further splits with positive gain, best gain: -inf
[LightGBM] [Warning] No further splits with positive gain, best gain: -inf
[LightGBM] [Warning] No further splits with positive gain, best gain: -inf
[LightGBM] [Warning] No further splits with positive gain, best gain: -inf
[LightGBM] [Warning] No further splits with positive gain, best gain: -inf
[LightGBM] [Warning] No further splits with positive gain, best gain: -inf
[LightGBM] [Warning] No further splits with positive gain, best gain: -inf
[LightGBM] [Warning] No further splits with positive gain, best gain: -inf
[LightGBM] [Warning] No further splits with positive gain, best gain: -inf
[LightGBM] [Warning] No further splits with positive gain, best gain: -inf
[LightGBM] [Warning] No further splits with positive gain, best gain: -inf
[LightGBM] [Warning] Accuracy may be bad since you didn't explicitly set num_leaves OR 2^max_depth > num_leaves. (num_leaves=31).
[LightGBM] [Warning] Accuracy may be bad since you didn't explicitly set num_leaves OR 2^max_depth > num_leaves. (num_leaves=31).
[LightGBM] [Warning] Accuracy may be bad since you didn't explicitly set num_leaves OR 2^max_depth > num_leaves. (num_leaves=31).
[LightGBM] [Info] Auto-choosing col-wise multi-threading, the overhead of testing was 0.000052 seconds.
You can set `force_col_wise=true` to remove the overhead.
[LightGBM] [Info] Total Bins 250
[LightGBM] [Info] Number of data points in the train set: 750, number of used features: 1
[LightGBM] [Info] Start training from score 155.499798
[LightGBM] [Warning] No further splits with positive gain, best gain: -inf
[LightGBM] [Warning] No further splits with positive gain, best gain: -inf
[LightGBM] [Warning] No further splits with positive gain, best gain: -inf
[LightGBM] [Warning] No further splits with positive gain, best gain: -inf
[LightGBM] [Warning] No further splits with positive gain, best gain: -inf
[LightGBM] [Warning] No further splits with positive gain, best gain: -inf
[LightGBM] [Warning] No further splits with positive gain, best gain: -inf
[LightGBM] [Warning] No further splits with positive gain, best gain: -inf
[LightGBM] [Warning] No further splits with positive gain, best gain: -inf
[LightGBM] [Warning] No further splits with positive gain, best gain: -inf
[LightGBM] [Warning] No further splits with positive gain, best gain: -inf
[LightGBM] [Warning] No further splits with positive gain, best gain: -inf
[LightGBM] [Warning] No further splits with positive gain, best gain: -inf
[LightGBM] [Warning] No further splits with positive gain, best gain: -inf
[LightGBM] [Warning] No further splits with positive gain, best gain: -inf
[LightGBM] [Warning] No further splits with positive gain, best gain: -inf
[LightGBM] [Warning] No further splits with positive gain, best gain: -inf
[LightGBM] [Warning] No further splits with positive gain, best gain: -inf
[LightGBM] [Warning] No further splits with positive gain, best gain: -inf
[LightGBM] [Warning] No further splits with positive gain, best gain: -inf
[LightGBM] [Warning] Accuracy may be bad since you didn't explicitly set num_leaves OR 2^max_depth > num_leaves. (num_leaves=31).
[LightGBM] [Warning] Accuracy may be bad since you didn't explicitly set num_leaves OR 2^max_depth > num_leaves. (num_leaves=31).
[LightGBM] [Warning] Accuracy may be bad since you didn't explicitly set num_leaves OR 2^max_depth > num_leaves. (num_leaves=31).
[LightGBM] [Info] Auto-choosing col-wise multi-threading, the overhead of testing was 0.000045 seconds.
You can set `force_col_wise=true` to remove the overhead.
[LightGBM] [Info] Total Bins 250
[LightGBM] [Info] Number of data points in the train set: 750, number of used features: 1
[LightGBM] [Info] Start training from score 155.499798
[LightGBM] [Warning] No further splits with positive gain, best gain: -inf
[LightGBM] [Warning] No further splits with positive gain, best gain: -inf
[LightGBM] [Warning] No further splits with positive gain, best gain: -inf
[LightGBM] [Warning] No further splits with positive gain, best gain: -inf
[LightGBM] [Warning] No further splits with positive gain, best gain: -inf
[LightGBM] [Warning] No further splits with positive gain, best gain: -inf
[LightGBM] [Warning] No further splits with positive gain, best gain: -inf
[LightGBM] [Warning] No further splits with positive gain, best gain: -inf
[LightGBM] [Warning] No further splits with positive gain, best gain: -inf
[LightGBM] [Warning] No further splits with positive gain, best gain: -inf
[LightGBM] [Warning] No further splits with positive gain, best gain: -inf
[LightGBM] [Warning] No further splits with positive gain, best gain: -inf
[LightGBM] [Warning] No further splits with positive gain, best gain: -inf
[LightGBM] [Warning] No further splits with positive gain, best gain: -inf
[LightGBM] [Warning] No further splits with positive gain, best gain: -inf
[LightGBM] [Warning] No further splits with positive gain, best gain: -inf
[LightGBM] [Warning] No further splits with positive gain, best gain: -inf
[LightGBM] [Warning] No further splits with positive gain, best gain: -inf
[LightGBM] [Warning] No further splits with positive gain, best gain: -inf
[LightGBM] [Warning] No further splits with positive gain, best gain: -inf
[LightGBM] [Warning] Accuracy may be bad since you didn't explicitly set num_leaves OR 2^max_depth > num_leaves. (num_leaves=31).
[LightGBM] [Warning] Accuracy may be bad since you didn't explicitly set num_leaves OR 2^max_depth > num_leaves. (num_leaves=31).
[LightGBM] [Warning] Accuracy may be bad since you didn't explicitly set num_leaves OR 2^max_depth > num_leaves. (num_leaves=31).
[LightGBM] [Info] Auto-choosing col-wise multi-threading, the overhead of testing was 0.000046 seconds.
You can set `force_col_wise=true` to remove the overhead.
[LightGBM] [Info] Total Bins 251
[LightGBM] [Info] Number of data points in the train set: 750, number of used features: 1
[LightGBM] [Info] Start training from score 155.414976
[LightGBM] [Warning] No further splits with positive gain, best gain: -inf
[LightGBM] [Warning] No further splits with positive gain, best gain: -inf
[LightGBM] [Warning] No further splits with positive gain, best gain: -inf
[LightGBM] [Warning] No further splits with positive gain, best gain: -inf
[LightGBM] [Warning] No further splits with positive gain, best gain: -inf
[LightGBM] [Warning] No further splits with positive gain, best gain: -inf
[LightGBM] [Warning] No further splits with positive gain, best gain: -inf
[LightGBM] [Warning] No further splits with positive gain, best gain: -inf
[LightGBM] [Warning] No further splits with positive gain, best gain: -inf
[LightGBM] [Warning] No further splits with positive gain, best gain: -inf
[LightGBM] [Warning] No further splits with positive gain, best gain: -inf
[LightGBM] [Warning] No further splits with positive gain, best gain: -inf
[LightGBM] [Warning] No further splits with positive gain, best gain: -inf
[LightGBM] [Warning] No further splits with positive gain, best gain: -inf
[LightGBM] [Warning] No further splits with positive gain, best gain: -inf
[LightGBM] [Warning] No further splits with positive gain, best gain: -inf
[LightGBM] [Warning] No further splits with positive gain, best gain: -inf
[LightGBM] [Warning] No further splits with positive gain, best gain: -inf
[LightGBM] [Warning] No further splits with positive gain, best gain: -inf
[LightGBM] [Warning] No further splits with positive gain, best gain: -inf
[LightGBM] [Warning] Accuracy may be bad since you didn't explicitly set num_leaves OR 2^max_depth > num_leaves. (num_leaves=31).
[LightGBM] [Warning] Accuracy may be bad since you didn't explicitly set num_leaves OR 2^max_depth > num_leaves. (num_leaves=31).
32%|███▏ | 8/25 [00:00<00:01, 10.56it/s]
[LightGBM] [Warning] Accuracy may be bad since you didn't explicitly set num_leaves OR 2^max_depth > num_leaves. (num_leaves=31).
[LightGBM] [Info] Auto-choosing col-wise multi-threading, the overhead of testing was 0.000054 seconds.
You can set `force_col_wise=true` to remove the overhead.
[LightGBM] [Info] Total Bins 251
[LightGBM] [Info] Number of data points in the train set: 750, number of used features: 1
[LightGBM] [Info] Start training from score 155.414976
[LightGBM] [Warning] No further splits with positive gain, best gain: -inf
[LightGBM] [Warning] No further splits with positive gain, best gain: -inf
[LightGBM] [Warning] No further splits with positive gain, best gain: -inf
[LightGBM] [Warning] No further splits with positive gain, best gain: -inf
[LightGBM] [Warning] No further splits with positive gain, best gain: -inf
[LightGBM] [Warning] No further splits with positive gain, best gain: -inf
[LightGBM] [Warning] No further splits with positive gain, best gain: -inf
[LightGBM] [Warning] No further splits with positive gain, best gain: -inf
[LightGBM] [Warning] No further splits with positive gain, best gain: -inf
[LightGBM] [Warning] No further splits with positive gain, best gain: -inf
[LightGBM] [Warning] No further splits with positive gain, best gain: -inf
[LightGBM] [Warning] No further splits with positive gain, best gain: -inf
[LightGBM] [Warning] No further splits with positive gain, best gain: -inf
[LightGBM] [Warning] No further splits with positive gain, best gain: -inf
[LightGBM] [Warning] No further splits with positive gain, best gain: -inf
[LightGBM] [Warning] No further splits with positive gain, best gain: -inf
[LightGBM] [Warning] No further splits with positive gain, best gain: -inf
[LightGBM] [Warning] No further splits with positive gain, best gain: -inf
[LightGBM] [Warning] No further splits with positive gain, best gain: -inf
[LightGBM] [Warning] No further splits with positive gain, best gain: -inf
[LightGBM] [Warning] Accuracy may be bad since you didn't explicitly set num_leaves OR 2^max_depth > num_leaves. (num_leaves=31).
[LightGBM] [Warning] Accuracy may be bad since you didn't explicitly set num_leaves OR 2^max_depth > num_leaves. (num_leaves=31).
[LightGBM] [Warning] Accuracy may be bad since you didn't explicitly set num_leaves OR 2^max_depth > num_leaves. (num_leaves=31).
[LightGBM] [Info] Auto-choosing col-wise multi-threading, the overhead of testing was 0.000245 seconds.
You can set `force_col_wise=true` to remove the overhead.
[LightGBM] [Info] Total Bins 251
[LightGBM] [Info] Number of data points in the train set: 750, number of used features: 1
[LightGBM] [Info] Start training from score 155.414976
[LightGBM] [Warning] No further splits with positive gain, best gain: -inf
[LightGBM] [Warning] No further splits with positive gain, best gain: -inf
[LightGBM] [Warning] No further splits with positive gain, best gain: -inf
[LightGBM] [Warning] No further splits with positive gain, best gain: -inf
[LightGBM] [Warning] No further splits with positive gain, best gain: -inf
[LightGBM] [Warning] No further splits with positive gain, best gain: -inf
[LightGBM] [Warning] No further splits with positive gain, best gain: -inf
[LightGBM] [Warning] No further splits with positive gain, best gain: -inf
[LightGBM] [Warning] No further splits with positive gain, best gain: -inf
[LightGBM] [Warning] No further splits with positive gain, best gain: -inf
[LightGBM] [Warning] No further splits with positive gain, best gain: -inf
[LightGBM] [Warning] No further splits with positive gain, best gain: -inf
[LightGBM] [Warning] No further splits with positive gain, best gain: -inf
[LightGBM] [Warning] No further splits with positive gain, best gain: -inf
[LightGBM] [Warning] No further splits with positive gain, best gain: -inf
[LightGBM] [Warning] No further splits with positive gain, best gain: -inf
[LightGBM] [Warning] No further splits with positive gain, best gain: -inf
[LightGBM] [Warning] No further splits with positive gain, best gain: -inf
[LightGBM] [Warning] No further splits with positive gain, best gain: -inf
[LightGBM] [Warning] No further splits with positive gain, best gain: -inf
[LightGBM] [Warning] Accuracy may be bad since you didn't explicitly set num_leaves OR 2^max_depth > num_leaves. (num_leaves=31).
[LightGBM] [Warning] Accuracy may be bad since you didn't explicitly set num_leaves OR 2^max_depth > num_leaves. (num_leaves=31).
[LightGBM] [Warning] Accuracy may be bad since you didn't explicitly set num_leaves OR 2^max_depth > num_leaves. (num_leaves=31).
[LightGBM] [Info] Auto-choosing col-wise multi-threading, the overhead of testing was 0.000071 seconds.
You can set `force_col_wise=true` to remove the overhead.
[LightGBM] [Info] Total Bins 250
[LightGBM] [Info] Number of data points in the train set: 750, number of used features: 1
[LightGBM] [Info] Start training from score 155.525282
[LightGBM] [Warning] No further splits with positive gain, best gain: -inf
[LightGBM] [Warning] No further splits with positive gain, best gain: -inf
[LightGBM] [Warning] No further splits with positive gain, best gain: -inf
[LightGBM] [Warning] No further splits with positive gain, best gain: -inf
[LightGBM] [Warning] No further splits with positive gain, best gain: -inf
[LightGBM] [Warning] No further splits with positive gain, best gain: -inf
[LightGBM] [Warning] No further splits with positive gain, best gain: -inf
[LightGBM] [Warning] No further splits with positive gain, best gain: -inf
[LightGBM] [Warning] No further splits with positive gain, best gain: -inf
[LightGBM] [Warning] No further splits with positive gain, best gain: -inf
[LightGBM] [Warning] No further splits with positive gain, best gain: -inf
[LightGBM] [Warning] No further splits with positive gain, best gain: -inf
[LightGBM] [Warning] No further splits with positive gain, best gain: -inf
[LightGBM] [Warning] No further splits with positive gain, best gain: -inf
[LightGBM] [Warning] No further splits with positive gain, best gain: -inf
[LightGBM] [Warning] No further splits with positive gain, best gain: -inf
[LightGBM] [Warning] No further splits with positive gain, best gain: -inf
[LightGBM] [Warning] No further splits with positive gain, best gain: -inf
[LightGBM] [Warning] No further splits with positive gain, best gain: -inf
[LightGBM] [Warning] No further splits with positive gain, best gain: -inf
[LightGBM] [Warning] Accuracy may be bad since you didn't explicitly set num_leaves OR 2^max_depth > num_leaves. (num_leaves=31).
[LightGBM] [Warning] Accuracy may be bad since you didn't explicitly set num_leaves OR 2^max_depth > num_leaves. (num_leaves=31).
[LightGBM] [Warning] Accuracy may be bad since you didn't explicitly set num_leaves OR 2^max_depth > num_leaves. (num_leaves=31).
[LightGBM] [Info] Auto-choosing col-wise multi-threading, the overhead of testing was 0.000068 seconds.
You can set `force_col_wise=true` to remove the overhead.
[LightGBM] [Info] Total Bins 250
[LightGBM] [Info] Number of data points in the train set: 750, number of used features: 1
[LightGBM] [Info] Start training from score 155.525282
[LightGBM] [Warning] No further splits with positive gain, best gain: -inf
[LightGBM] [Warning] No further splits with positive gain, best gain: -inf
[LightGBM] [Warning] No further splits with positive gain, best gain: -inf
[LightGBM] [Warning] No further splits with positive gain, best gain: -inf
[LightGBM] [Warning] No further splits with positive gain, best gain: -inf
[LightGBM] [Warning] No further splits with positive gain, best gain: -inf
[LightGBM] [Warning] No further splits with positive gain, best gain: -inf
[LightGBM] [Warning] No further splits with positive gain, best gain: -inf
[LightGBM] [Warning] No further splits with positive gain, best gain: -inf
[LightGBM] [Warning] No further splits with positive gain, best gain: -inf
[LightGBM] [Warning] No further splits with positive gain, best gain: -inf
[LightGBM] [Warning] No further splits with positive gain, best gain: -inf
[LightGBM] [Warning] No further splits with positive gain, best gain: -inf
[LightGBM] [Warning] No further splits with positive gain, best gain: -inf
[LightGBM] [Warning] No further splits with positive gain, best gain: -inf
[LightGBM] [Warning] No further splits with positive gain, best gain: -inf
[LightGBM] [Warning] No further splits with positive gain, best gain: -inf
[LightGBM] [Warning] No further splits with positive gain, best gain: -inf
[LightGBM] [Warning] No further splits with positive gain, best gain: -inf
[LightGBM] [Warning] No further splits with positive gain, best gain: -inf
[LightGBM] [Warning] Accuracy may be bad since you didn't explicitly set num_leaves OR 2^max_depth > num_leaves. (num_leaves=31).
[LightGBM] [Warning] Accuracy may be bad since you didn't explicitly set num_leaves OR 2^max_depth > num_leaves. (num_leaves=31).
[LightGBM] [Warning] Accuracy may be bad since you didn't explicitly set num_leaves OR 2^max_depth > num_leaves. (num_leaves=31).
[LightGBM] [Info] Auto-choosing col-wise multi-threading, the overhead of testing was 0.000072 seconds.
You can set `force_col_wise=true` to remove the overhead.
[LightGBM] [Info] Total Bins 250
[LightGBM] [Info] Number of data points in the train set: 750, number of used features: 1
[LightGBM] [Info] Start training from score 155.525282
[LightGBM] [Warning] No further splits with positive gain, best gain: -inf
[LightGBM] [Warning] No further splits with positive gain, best gain: -inf
[LightGBM] [Warning] No further splits with positive gain, best gain: -inf
[LightGBM] [Warning] No further splits with positive gain, best gain: -inf
[LightGBM] [Warning] No further splits with positive gain, best gain: -inf
[LightGBM] [Warning] No further splits with positive gain, best gain: -inf
[LightGBM] [Warning] No further splits with positive gain, best gain: -inf
[LightGBM] [Warning] No further splits with positive gain, best gain: -inf
[LightGBM] [Warning] No further splits with positive gain, best gain: -inf
[LightGBM] [Warning] No further splits with positive gain, best gain: -inf
[LightGBM] [Warning] No further splits with positive gain, best gain: -inf
[LightGBM] [Warning] No further splits with positive gain, best gain: -inf
[LightGBM] [Warning] No further splits with positive gain, best gain: -inf
[LightGBM] [Warning] No further splits with positive gain, best gain: -inf
[LightGBM] [Warning] No further splits with positive gain, best gain: -inf
[LightGBM] [Warning] No further splits with positive gain, best gain: -inf
[LightGBM] [Warning] No further splits with positive gain, best gain: -inf
[LightGBM] [Warning] No further splits with positive gain, best gain: -inf
[LightGBM] [Warning] No further splits with positive gain, best gain: -inf
[LightGBM] [Warning] No further splits with positive gain, best gain: -inf
[LightGBM] [Warning] Accuracy may be bad since you didn't explicitly set num_leaves OR 2^max_depth > num_leaves. (num_leaves=31).
40%|████ | 10/25 [00:01<00:01, 9.77it/s]
[LightGBM] [Warning] Accuracy may be bad since you didn't explicitly set num_leaves OR 2^max_depth > num_leaves. (num_leaves=31).
[LightGBM] [Warning] Accuracy may be bad since you didn't explicitly set num_leaves OR 2^max_depth > num_leaves. (num_leaves=31).
[LightGBM] [Info] Auto-choosing col-wise multi-threading, the overhead of testing was 0.000042 seconds.
You can set `force_col_wise=true` to remove the overhead.
[LightGBM] [Info] Total Bins 251
[LightGBM] [Info] Number of data points in the train set: 750, number of used features: 1
[LightGBM] [Info] Start training from score 155.312694
[LightGBM] [Warning] No further splits with positive gain, best gain: -inf
[LightGBM] [Warning] No further splits with positive gain, best gain: -inf
[LightGBM] [Warning] No further splits with positive gain, best gain: -inf
[LightGBM] [Warning] No further splits with positive gain, best gain: -inf
[LightGBM] [Warning] No further splits with positive gain, best gain: -inf
[LightGBM] [Warning] No further splits with positive gain, best gain: -inf
[LightGBM] [Warning] No further splits with positive gain, best gain: -inf
[LightGBM] [Warning] No further splits with positive gain, best gain: -inf
[LightGBM] [Warning] No further splits with positive gain, best gain: -inf
[LightGBM] [Warning] No further splits with positive gain, best gain: -inf
[LightGBM] [Warning] No further splits with positive gain, best gain: -inf
[LightGBM] [Warning] No further splits with positive gain, best gain: -inf
[LightGBM] [Warning] No further splits with positive gain, best gain: -inf
[LightGBM] [Warning] No further splits with positive gain, best gain: -inf
[LightGBM] [Warning] No further splits with positive gain, best gain: -inf
[LightGBM] [Warning] No further splits with positive gain, best gain: -inf
[LightGBM] [Warning] No further splits with positive gain, best gain: -inf
[LightGBM] [Warning] No further splits with positive gain, best gain: -inf
[LightGBM] [Warning] No further splits with positive gain, best gain: -inf
[LightGBM] [Warning] No further splits with positive gain, best gain: -inf
[LightGBM] [Warning] Accuracy may be bad since you didn't explicitly set num_leaves OR 2^max_depth > num_leaves. (num_leaves=31).
[LightGBM] [Warning] Accuracy may be bad since you didn't explicitly set num_leaves OR 2^max_depth > num_leaves. (num_leaves=31).
[LightGBM] [Warning] Accuracy may be bad since you didn't explicitly set num_leaves OR 2^max_depth > num_leaves. (num_leaves=31).
[LightGBM] [Info] Auto-choosing col-wise multi-threading, the overhead of testing was 0.000042 seconds.
You can set `force_col_wise=true` to remove the overhead.
[LightGBM] [Info] Total Bins 251
[LightGBM] [Info] Number of data points in the train set: 750, number of used features: 1
[LightGBM] [Info] Start training from score 155.312694
[LightGBM] [Warning] No further splits with positive gain, best gain: -inf
[LightGBM] [Warning] No further splits with positive gain, best gain: -inf
[LightGBM] [Warning] No further splits with positive gain, best gain: -inf
[LightGBM] [Warning] No further splits with positive gain, best gain: -inf
[LightGBM] [Warning] No further splits with positive gain, best gain: -inf
[LightGBM] [Warning] No further splits with positive gain, best gain: -inf
[LightGBM] [Warning] No further splits with positive gain, best gain: -inf
[LightGBM] [Warning] No further splits with positive gain, best gain: -inf
[LightGBM] [Warning] No further splits with positive gain, best gain: -inf
[LightGBM] [Warning] No further splits with positive gain, best gain: -inf
[LightGBM] [Warning] No further splits with positive gain, best gain: -inf
[LightGBM] [Warning] No further splits with positive gain, best gain: -inf
[LightGBM] [Warning] No further splits with positive gain, best gain: -inf
[LightGBM] [Warning] No further splits with positive gain, best gain: -inf
[LightGBM] [Warning] No further splits with positive gain, best gain: -inf
[LightGBM] [Warning] No further splits with positive gain, best gain: -inf
[LightGBM] [Warning] No further splits with positive gain, best gain: -inf
[LightGBM] [Warning] No further splits with positive gain, best gain: -inf
[LightGBM] [Warning] No further splits with positive gain, best gain: -inf
[LightGBM] [Warning] No further splits with positive gain, best gain: -inf
[LightGBM] [Warning] Accuracy may be bad since you didn't explicitly set num_leaves OR 2^max_depth > num_leaves. (num_leaves=31).
[LightGBM] [Warning] Accuracy may be bad since you didn't explicitly set num_leaves OR 2^max_depth > num_leaves. (num_leaves=31).
[LightGBM] [Warning] Accuracy may be bad since you didn't explicitly set num_leaves OR 2^max_depth > num_leaves. (num_leaves=31).
[LightGBM] [Info] Auto-choosing col-wise multi-threading, the overhead of testing was 0.000042 seconds.
You can set `force_col_wise=true` to remove the overhead.
[LightGBM] [Info] Total Bins 251
[LightGBM] [Info] Number of data points in the train set: 750, number of used features: 1
[LightGBM] [Info] Start training from score 155.312694
[LightGBM] [Warning] No further splits with positive gain, best gain: -inf
[LightGBM] [Warning] No further splits with positive gain, best gain: -inf
[LightGBM] [Warning] No further splits with positive gain, best gain: -inf
[LightGBM] [Warning] No further splits with positive gain, best gain: -inf
[LightGBM] [Warning] No further splits with positive gain, best gain: -inf
[LightGBM] [Warning] No further splits with positive gain, best gain: -inf
[LightGBM] [Warning] No further splits with positive gain, best gain: -inf
[LightGBM] [Warning] No further splits with positive gain, best gain: -inf
[LightGBM] [Warning] No further splits with positive gain, best gain: -inf
[LightGBM] [Warning] No further splits with positive gain, best gain: -inf
[LightGBM] [Warning] No further splits with positive gain, best gain: -inf
[LightGBM] [Warning] No further splits with positive gain, best gain: -inf
[LightGBM] [Warning] No further splits with positive gain, best gain: -inf
[LightGBM] [Warning] No further splits with positive gain, best gain: -inf
[LightGBM] [Warning] No further splits with positive gain, best gain: -inf
[LightGBM] [Warning] No further splits with positive gain, best gain: -inf
[LightGBM] [Warning] No further splits with positive gain, best gain: -inf
[LightGBM] [Warning] No further splits with positive gain, best gain: -inf
[LightGBM] [Warning] No further splits with positive gain, best gain: -inf
[LightGBM] [Warning] No further splits with positive gain, best gain: -inf
[LightGBM] [Warning] Accuracy may be bad since you didn't explicitly set num_leaves OR 2^max_depth > num_leaves. (num_leaves=31).
[LightGBM] [Warning] Accuracy may be bad since you didn't explicitly set num_leaves OR 2^max_depth > num_leaves. (num_leaves=31).
[LightGBM] [Warning] Accuracy may be bad since you didn't explicitly set num_leaves OR 2^max_depth > num_leaves. (num_leaves=31).
[LightGBM] [Info] Auto-choosing col-wise multi-threading, the overhead of testing was 0.000047 seconds.
You can set `force_col_wise=true` to remove the overhead.
[LightGBM] [Info] Total Bins 251
[LightGBM] [Info] Number of data points in the train set: 750, number of used features: 1
[LightGBM] [Info] Start training from score 155.210617
[LightGBM] [Warning] No further splits with positive gain, best gain: -inf
[LightGBM] [Warning] No further splits with positive gain, best gain: -inf
[LightGBM] [Warning] No further splits with positive gain, best gain: -inf
[LightGBM] [Warning] No further splits with positive gain, best gain: -inf
[LightGBM] [Warning] No further splits with positive gain, best gain: -inf
[LightGBM] [Warning] No further splits with positive gain, best gain: -inf
[LightGBM] [Warning] No further splits with positive gain, best gain: -inf
[LightGBM] [Warning] No further splits with positive gain, best gain: -inf
[LightGBM] [Warning] No further splits with positive gain, best gain: -inf
[LightGBM] [Warning] No further splits with positive gain, best gain: -inf
[LightGBM] [Warning] No further splits with positive gain, best gain: -inf
[LightGBM] [Warning] No further splits with positive gain, best gain: -inf
[LightGBM] [Warning] No further splits with positive gain, best gain: -inf
[LightGBM] [Warning] No further splits with positive gain, best gain: -inf
[LightGBM] [Warning] No further splits with positive gain, best gain: -inf
[LightGBM] [Warning] No further splits with positive gain, best gain: -inf
[LightGBM] [Warning] No further splits with positive gain, best gain: -inf
[LightGBM] [Warning] No further splits with positive gain, best gain: -inf
[LightGBM] [Warning] No further splits with positive gain, best gain: -inf
[LightGBM] [Warning] No further splits with positive gain, best gain: -inf
[LightGBM] [Warning] Accuracy may be bad since you didn't explicitly set num_leaves OR 2^max_depth > num_leaves. (num_leaves=31).
[LightGBM] [Warning] Accuracy may be bad since you didn't explicitly set num_leaves OR 2^max_depth > num_leaves. (num_leaves=31).
[LightGBM] [Warning] Accuracy may be bad since you didn't explicitly set num_leaves OR 2^max_depth > num_leaves. (num_leaves=31).
[LightGBM] [Info] Auto-choosing col-wise multi-threading, the overhead of testing was 0.000045 seconds.
You can set `force_col_wise=true` to remove the overhead.
[LightGBM] [Info] Total Bins 251
[LightGBM] [Info] Number of data points in the train set: 750, number of used features: 1
[LightGBM] [Info] Start training from score 155.210617
[LightGBM] [Warning] No further splits with positive gain, best gain: -inf
[LightGBM] [Warning] No further splits with positive gain, best gain: -inf
[LightGBM] [Warning] No further splits with positive gain, best gain: -inf
[LightGBM] [Warning] No further splits with positive gain, best gain: -inf
[LightGBM] [Warning] No further splits with positive gain, best gain: -inf
[LightGBM] [Warning] No further splits with positive gain, best gain: -inf
[LightGBM] [Warning] No further splits with positive gain, best gain: -inf
[LightGBM] [Warning] No further splits with positive gain, best gain: -inf
[LightGBM] [Warning] No further splits with positive gain, best gain: -inf
[LightGBM] [Warning] No further splits with positive gain, best gain: -inf
[LightGBM] [Warning] No further splits with positive gain, best gain: -inf
[LightGBM] [Warning] No further splits with positive gain, best gain: -inf
[LightGBM] [Warning] No further splits with positive gain, best gain: -inf
[LightGBM] [Warning] No further splits with positive gain, best gain: -inf
[LightGBM] [Warning] No further splits with positive gain, best gain: -inf
[LightGBM] [Warning] No further splits with positive gain, best gain: -inf
[LightGBM] [Warning] No further splits with positive gain, best gain: -inf
[LightGBM] [Warning] No further splits with positive gain, best gain: -inf
[LightGBM] [Warning] No further splits with positive gain, best gain: -inf
[LightGBM] [Warning] No further splits with positive gain, best gain: -inf
[LightGBM] [Warning] Accuracy may be bad since you didn't explicitly set num_leaves OR 2^max_depth > num_leaves. (num_leaves=31).
[LightGBM] [Warning] Accuracy may be bad since you didn't explicitly set num_leaves OR 2^max_depth > num_leaves. (num_leaves=31).
[LightGBM] [Warning] Accuracy may be bad since you didn't explicitly set num_leaves OR 2^max_depth > num_leaves. (num_leaves=31).
[LightGBM] [Info] Auto-choosing col-wise multi-threading, the overhead of testing was 0.000039 seconds.
You can set `force_col_wise=true` to remove the overhead.
[LightGBM] [Info] Total Bins 251
[LightGBM] [Info] Number of data points in the train set: 750, number of used features: 1
[LightGBM] [Info] Start training from score 155.210617
[LightGBM] [Warning] No further splits with positive gain, best gain: -inf
[LightGBM] [Warning] No further splits with positive gain, best gain: -inf
[LightGBM] [Warning] No further splits with positive gain, best gain: -inf
[LightGBM] [Warning] No further splits with positive gain, best gain: -inf
[LightGBM] [Warning] No further splits with positive gain, best gain: -inf
[LightGBM] [Warning] No further splits with positive gain, best gain: -inf
[LightGBM] [Warning] No further splits with positive gain, best gain: -inf
[LightGBM] [Warning] No further splits with positive gain, best gain: -inf
[LightGBM] [Warning] No further splits with positive gain, best gain: -inf
[LightGBM] [Warning] No further splits with positive gain, best gain: -inf
[LightGBM] [Warning] No further splits with positive gain, best gain: -inf
[LightGBM] [Warning] No further splits with positive gain, best gain: -inf
[LightGBM] [Warning] No further splits with positive gain, best gain: -inf
[LightGBM] [Warning] No further splits with positive gain, best gain: -inf
[LightGBM] [Warning] No further splits with positive gain, best gain: -inf
[LightGBM] [Warning] No further splits with positive gain, best gain: -inf
[LightGBM] [Warning] No further splits with positive gain, best gain: -inf
[LightGBM] [Warning] No further splits with positive gain, best gain: -inf
[LightGBM] [Warning] No further splits with positive gain, best gain: -inf
[LightGBM] [Warning] No further splits with positive gain, best gain: -inf
[LightGBM] [Warning] Accuracy may be bad since you didn't explicitly set num_leaves OR 2^max_depth > num_leaves. (num_leaves=31).
[LightGBM] [Warning] Accuracy may be bad since you didn't explicitly set num_leaves OR 2^max_depth > num_leaves. (num_leaves=31).
[LightGBM] [Warning] Accuracy may be bad since you didn't explicitly set num_leaves OR 2^max_depth > num_leaves. (num_leaves=31).
[LightGBM] [Info] Auto-choosing col-wise multi-threading, the overhead of testing was 0.000044 seconds.
You can set `force_col_wise=true` to remove the overhead.
[LightGBM] [Info] Total Bins 250
[LightGBM] [Info] Number of data points in the train set: 750, number of used features: 1
[LightGBM] [Info] Start training from score 154.757290
[LightGBM] [Warning] No further splits with positive gain, best gain: -inf
[LightGBM] [Warning] No further splits with positive gain, best gain: -inf
[LightGBM] [Warning] No further splits with positive gain, best gain: -inf
[LightGBM] [Warning] No further splits with positive gain, best gain: -inf
[LightGBM] [Warning] No further splits with positive gain, best gain: -inf
[LightGBM] [Warning] No further splits with positive gain, best gain: -inf
[LightGBM] [Warning] No further splits with positive gain, best gain: -inf
[LightGBM] [Warning] No further splits with positive gain, best gain: -inf
[LightGBM] [Warning] No further splits with positive gain, best gain: -inf
[LightGBM] [Warning] No further splits with positive gain, best gain: -inf
[LightGBM] [Warning] No further splits with positive gain, best gain: -inf
[LightGBM] [Warning] No further splits with positive gain, best gain: -inf
[LightGBM] [Warning] No further splits with positive gain, best gain: -inf
[LightGBM] [Warning] No further splits with positive gain, best gain: -inf
[LightGBM] [Warning] No further splits with positive gain, best gain: -inf
[LightGBM] [Warning] No further splits with positive gain, best gain: -inf
[LightGBM] [Warning] No further splits with positive gain, best gain: -inf
[LightGBM] [Warning] No further splits with positive gain, best gain: -inf
[LightGBM] [Warning] No further splits with positive gain, best gain: -inf
[LightGBM] [Warning] No further splits with positive gain, best gain: -inf
[LightGBM] [Warning] Accuracy may be bad since you didn't explicitly set num_leaves OR 2^max_depth > num_leaves. (num_leaves=31).
[LightGBM] [Warning] Accuracy may be bad since you didn't explicitly set num_leaves OR 2^max_depth > num_leaves. (num_leaves=31).
[LightGBM] [Warning] Accuracy may be bad since you didn't explicitly set num_leaves OR 2^max_depth > num_leaves. (num_leaves=31).
[LightGBM] [Info] Auto-choosing col-wise multi-threading, the overhead of testing was 0.000042 seconds.
You can set `force_col_wise=true` to remove the overhead.
[LightGBM] [Info] Total Bins 250
[LightGBM] [Info] Number of data points in the train set: 750, number of used features: 1
[LightGBM] [Info] Start training from score 154.757290
[LightGBM] [Warning] No further splits with positive gain, best gain: -inf
[LightGBM] [Warning] No further splits with positive gain, best gain: -inf
[LightGBM] [Warning] No further splits with positive gain, best gain: -inf
[LightGBM] [Warning] No further splits with positive gain, best gain: -inf
[LightGBM] [Warning] No further splits with positive gain, best gain: -inf
[LightGBM] [Warning] No further splits with positive gain, best gain: -inf
[LightGBM] [Warning] No further splits with positive gain, best gain: -inf
[LightGBM] [Warning] No further splits with positive gain, best gain: -inf
[LightGBM] [Warning] No further splits with positive gain, best gain: -inf
[LightGBM] [Warning] No further splits with positive gain, best gain: -inf
[LightGBM] [Warning] No further splits with positive gain, best gain: -inf
[LightGBM] [Warning] No further splits with positive gain, best gain: -inf
[LightGBM] [Warning] No further splits with positive gain, best gain: -inf
[LightGBM] [Warning] No further splits with positive gain, best gain: -inf
[LightGBM] [Warning] No further splits with positive gain, best gain: -inf
[LightGBM] [Warning] No further splits with positive gain, best gain: -inf
[LightGBM] [Warning] No further splits with positive gain, best gain: -inf
[LightGBM] [Warning] No further splits with positive gain, best gain: -inf
[LightGBM] [Warning] No further splits with positive gain, best gain: -inf
[LightGBM] [Warning] No further splits with positive gain, best gain: -inf
[LightGBM] [Warning] Accuracy may be bad since you didn't explicitly set num_leaves OR 2^max_depth > num_leaves. (num_leaves=31).
[LightGBM] [Warning] Accuracy may be bad since you didn't explicitly set num_leaves OR 2^max_depth > num_leaves. (num_leaves=31).
[LightGBM] [Warning] Accuracy may be bad since you didn't explicitly set num_leaves OR 2^max_depth > num_leaves. (num_leaves=31).
[LightGBM] [Info] Auto-choosing col-wise multi-threading, the overhead of testing was 0.000051 seconds.
You can set `force_col_wise=true` to remove the overhead.
[LightGBM] [Info] Total Bins 250
[LightGBM] [Info] Number of data points in the train set: 750, number of used features: 1
[LightGBM] [Info] Start training from score 154.757290
[LightGBM] [Warning] No further splits with positive gain, best gain: -inf
48%|████▊ | 12/25 [00:01<00:01, 10.15it/s]
[LightGBM] [Warning] No further splits with positive gain, best gain: -inf
[LightGBM] [Warning] No further splits with positive gain, best gain: -inf
[LightGBM] [Warning] No further splits with positive gain, best gain: -inf
[LightGBM] [Warning] No further splits with positive gain, best gain: -inf
[LightGBM] [Warning] No further splits with positive gain, best gain: -inf
[LightGBM] [Warning] No further splits with positive gain, best gain: -inf
[LightGBM] [Warning] No further splits with positive gain, best gain: -inf
[LightGBM] [Warning] No further splits with positive gain, best gain: -inf
[LightGBM] [Warning] No further splits with positive gain, best gain: -inf
[LightGBM] [Warning] No further splits with positive gain, best gain: -inf
[LightGBM] [Warning] No further splits with positive gain, best gain: -inf
[LightGBM] [Warning] No further splits with positive gain, best gain: -inf
[LightGBM] [Warning] No further splits with positive gain, best gain: -inf
[LightGBM] [Warning] No further splits with positive gain, best gain: -inf
[LightGBM] [Warning] No further splits with positive gain, best gain: -inf
[LightGBM] [Warning] No further splits with positive gain, best gain: -inf
[LightGBM] [Warning] No further splits with positive gain, best gain: -inf
[LightGBM] [Warning] No further splits with positive gain, best gain: -inf
[LightGBM] [Warning] No further splits with positive gain, best gain: -inf
[LightGBM] [Warning] Accuracy may be bad since you didn't explicitly set num_leaves OR 2^max_depth > num_leaves. (num_leaves=31).
[LightGBM] [Warning] Accuracy may be bad since you didn't explicitly set num_leaves OR 2^max_depth > num_leaves. (num_leaves=31).
[LightGBM] [Warning] Accuracy may be bad since you didn't explicitly set num_leaves OR 2^max_depth > num_leaves. (num_leaves=31).
[LightGBM] [Info] Auto-choosing col-wise multi-threading, the overhead of testing was 0.000043 seconds.
You can set `force_col_wise=true` to remove the overhead.
[LightGBM] [Info] Total Bins 250
[LightGBM] [Info] Number of data points in the train set: 750, number of used features: 1
[LightGBM] [Info] Start training from score 155.371614
[LightGBM] [Warning] No further splits with positive gain, best gain: -inf
[LightGBM] [Warning] No further splits with positive gain, best gain: -inf
[LightGBM] [Warning] No further splits with positive gain, best gain: -inf
[LightGBM] [Warning] No further splits with positive gain, best gain: -inf
[LightGBM] [Warning] No further splits with positive gain, best gain: -inf
[LightGBM] [Warning] No further splits with positive gain, best gain: -inf
[LightGBM] [Warning] No further splits with positive gain, best gain: -inf
[LightGBM] [Warning] No further splits with positive gain, best gain: -inf
[LightGBM] [Warning] No further splits with positive gain, best gain: -inf
[LightGBM] [Warning] No further splits with positive gain, best gain: -inf
[LightGBM] [Warning] No further splits with positive gain, best gain: -inf
[LightGBM] [Warning] No further splits with positive gain, best gain: -inf
[LightGBM] [Warning] No further splits with positive gain, best gain: -inf
[LightGBM] [Warning] No further splits with positive gain, best gain: -inf
[LightGBM] [Warning] No further splits with positive gain, best gain: -inf
[LightGBM] [Warning] No further splits with positive gain, best gain: -inf
[LightGBM] [Warning] No further splits with positive gain, best gain: -inf
[LightGBM] [Warning] No further splits with positive gain, best gain: -inf
[LightGBM] [Warning] No further splits with positive gain, best gain: -inf
[LightGBM] [Warning] No further splits with positive gain, best gain: -inf
[LightGBM] [Warning] Accuracy may be bad since you didn't explicitly set num_leaves OR 2^max_depth > num_leaves. (num_leaves=31).
[LightGBM] [Warning] Accuracy may be bad since you didn't explicitly set num_leaves OR 2^max_depth > num_leaves. (num_leaves=31).
[LightGBM] [Warning] Accuracy may be bad since you didn't explicitly set num_leaves OR 2^max_depth > num_leaves. (num_leaves=31).
[LightGBM] [Info] Auto-choosing col-wise multi-threading, the overhead of testing was 0.000045 seconds.
You can set `force_col_wise=true` to remove the overhead.
[LightGBM] [Info] Total Bins 250
[LightGBM] [Info] Number of data points in the train set: 750, number of used features: 1
[LightGBM] [Info] Start training from score 155.371614
[LightGBM] [Warning] No further splits with positive gain, best gain: -inf
[LightGBM] [Warning] No further splits with positive gain, best gain: -inf
[LightGBM] [Warning] No further splits with positive gain, best gain: -inf
[LightGBM] [Warning] No further splits with positive gain, best gain: -inf
[LightGBM] [Warning] No further splits with positive gain, best gain: -inf
[LightGBM] [Warning] No further splits with positive gain, best gain: -inf
[LightGBM] [Warning] No further splits with positive gain, best gain: -inf
[LightGBM] [Warning] No further splits with positive gain, best gain: -inf
[LightGBM] [Warning] No further splits with positive gain, best gain: -inf
[LightGBM] [Warning] No further splits with positive gain, best gain: -inf
[LightGBM] [Warning] No further splits with positive gain, best gain: -inf
[LightGBM] [Warning] No further splits with positive gain, best gain: -inf
[LightGBM] [Warning] No further splits with positive gain, best gain: -inf
[LightGBM] [Warning] No further splits with positive gain, best gain: -inf
[LightGBM] [Warning] No further splits with positive gain, best gain: -inf
[LightGBM] [Warning] No further splits with positive gain, best gain: -inf
[LightGBM] [Warning] No further splits with positive gain, best gain: -inf
[LightGBM] [Warning] No further splits with positive gain, best gain: -inf
[LightGBM] [Warning] No further splits with positive gain, best gain: -inf
[LightGBM] [Warning] No further splits with positive gain, best gain: -inf
[LightGBM] [Warning] Accuracy may be bad since you didn't explicitly set num_leaves OR 2^max_depth > num_leaves. (num_leaves=31).
[LightGBM] [Warning] Accuracy may be bad since you didn't explicitly set num_leaves OR 2^max_depth > num_leaves. (num_leaves=31).
[LightGBM] [Warning] Accuracy may be bad since you didn't explicitly set num_leaves OR 2^max_depth > num_leaves. (num_leaves=31).
[LightGBM] [Info] Auto-choosing col-wise multi-threading, the overhead of testing was 0.000059 seconds.
You can set `force_col_wise=true` to remove the overhead.
[LightGBM] [Info] Total Bins 250
[LightGBM] [Info] Number of data points in the train set: 750, number of used features: 1
[LightGBM] [Info] Start training from score 155.371614
[LightGBM] [Warning] No further splits with positive gain, best gain: -inf
[LightGBM] [Warning] No further splits with positive gain, best gain: -inf
[LightGBM] [Warning] No further splits with positive gain, best gain: -inf
[LightGBM] [Warning] No further splits with positive gain, best gain: -inf
[LightGBM] [Warning] No further splits with positive gain, best gain: -inf
[LightGBM] [Warning] No further splits with positive gain, best gain: -inf
[LightGBM] [Warning] No further splits with positive gain, best gain: -inf
[LightGBM] [Warning] No further splits with positive gain, best gain: -inf
[LightGBM] [Warning] No further splits with positive gain, best gain: -inf
[LightGBM] [Warning] No further splits with positive gain, best gain: -inf
[LightGBM] [Warning] No further splits with positive gain, best gain: -inf
[LightGBM] [Warning] No further splits with positive gain, best gain: -inf
[LightGBM] [Warning] No further splits with positive gain, best gain: -inf
[LightGBM] [Warning] No further splits with positive gain, best gain: -inf
[LightGBM] [Warning] No further splits with positive gain, best gain: -inf
[LightGBM] [Warning] No further splits with positive gain, best gain: -inf
[LightGBM] [Warning] No further splits with positive gain, best gain: -inf
[LightGBM] [Warning] No further splits with positive gain, best gain: -inf
[LightGBM] [Warning] No further splits with positive gain, best gain: -inf
[LightGBM] [Warning] No further splits with positive gain, best gain: -inf
[LightGBM] [Warning] Accuracy may be bad since you didn't explicitly set num_leaves OR 2^max_depth > num_leaves. (num_leaves=31).
[LightGBM] [Warning] Accuracy may be bad since you didn't explicitly set num_leaves OR 2^max_depth > num_leaves. (num_leaves=31).
[LightGBM] [Warning] Accuracy may be bad since you didn't explicitly set num_leaves OR 2^max_depth > num_leaves. (num_leaves=31).
[LightGBM] [Info] Auto-choosing col-wise multi-threading, the overhead of testing was 0.000056 seconds.
You can set `force_col_wise=true` to remove the overhead.
[LightGBM] [Info] Total Bins 250
[LightGBM] [Info] Number of data points in the train set: 750, number of used features: 1
[LightGBM] [Info] Start training from score 155.379656
[LightGBM] [Warning] No further splits with positive gain, best gain: -inf
[LightGBM] [Warning] No further splits with positive gain, best gain: -inf
[LightGBM] [Warning] No further splits with positive gain, best gain: -inf
[LightGBM] [Warning] No further splits with positive gain, best gain: -inf
[LightGBM] [Warning] No further splits with positive gain, best gain: -inf
[LightGBM] [Warning] No further splits with positive gain, best gain: -inf
[LightGBM] [Warning] No further splits with positive gain, best gain: -inf
[LightGBM] [Warning] No further splits with positive gain, best gain: -inf
56%|█████▌ | 14/25 [00:01<00:01, 9.10it/s]
[LightGBM] [Warning] No further splits with positive gain, best gain: -inf
[LightGBM] [Warning] No further splits with positive gain, best gain: -inf
[LightGBM] [Warning] No further splits with positive gain, best gain: -inf
[LightGBM] [Warning] No further splits with positive gain, best gain: -inf
[LightGBM] [Warning] No further splits with positive gain, best gain: -inf
[LightGBM] [Warning] No further splits with positive gain, best gain: -inf
[LightGBM] [Warning] No further splits with positive gain, best gain: -inf
[LightGBM] [Warning] No further splits with positive gain, best gain: -inf
[LightGBM] [Warning] No further splits with positive gain, best gain: -inf
[LightGBM] [Warning] No further splits with positive gain, best gain: -inf
[LightGBM] [Warning] No further splits with positive gain, best gain: -inf
[LightGBM] [Warning] No further splits with positive gain, best gain: -inf
[LightGBM] [Warning] Accuracy may be bad since you didn't explicitly set num_leaves OR 2^max_depth > num_leaves. (num_leaves=31).
[LightGBM] [Warning] Accuracy may be bad since you didn't explicitly set num_leaves OR 2^max_depth > num_leaves. (num_leaves=31).
[LightGBM] [Warning] Accuracy may be bad since you didn't explicitly set num_leaves OR 2^max_depth > num_leaves. (num_leaves=31).
[LightGBM] [Info] Auto-choosing col-wise multi-threading, the overhead of testing was 0.000856 seconds.
You can set `force_col_wise=true` to remove the overhead.
[LightGBM] [Info] Total Bins 250
[LightGBM] [Info] Number of data points in the train set: 750, number of used features: 1
[LightGBM] [Info] Start training from score 155.379656
[LightGBM] [Warning] No further splits with positive gain, best gain: -inf
[LightGBM] [Warning] No further splits with positive gain, best gain: -inf
[LightGBM] [Warning] No further splits with positive gain, best gain: -inf
[LightGBM] [Warning] No further splits with positive gain, best gain: -inf
[LightGBM] [Warning] No further splits with positive gain, best gain: -inf
[LightGBM] [Warning] No further splits with positive gain, best gain: -inf
[LightGBM] [Warning] No further splits with positive gain, best gain: -inf
[LightGBM] [Warning] No further splits with positive gain, best gain: -inf
[LightGBM] [Warning] No further splits with positive gain, best gain: -inf
[LightGBM] [Warning] No further splits with positive gain, best gain: -inf
[LightGBM] [Warning] No further splits with positive gain, best gain: -inf
[LightGBM] [Warning] No further splits with positive gain, best gain: -inf
[LightGBM] [Warning] No further splits with positive gain, best gain: -inf
[LightGBM] [Warning] No further splits with positive gain, best gain: -inf
[LightGBM] [Warning] No further splits with positive gain, best gain: -inf
[LightGBM] [Warning] No further splits with positive gain, best gain: -inf
[LightGBM] [Warning] No further splits with positive gain, best gain: -inf
[LightGBM] [Warning] No further splits with positive gain, best gain: -inf
[LightGBM] [Warning] No further splits with positive gain, best gain: -inf
[LightGBM] [Warning] No further splits with positive gain, best gain: -inf
[LightGBM] [Warning] Accuracy may be bad since you didn't explicitly set num_leaves OR 2^max_depth > num_leaves. (num_leaves=31).
[LightGBM] [Warning] Accuracy may be bad since you didn't explicitly set num_leaves OR 2^max_depth > num_leaves. (num_leaves=31).
[LightGBM] [Warning] Accuracy may be bad since you didn't explicitly set num_leaves OR 2^max_depth > num_leaves. (num_leaves=31).
[LightGBM] [Info] Auto-choosing col-wise multi-threading, the overhead of testing was 0.000071 seconds.
You can set `force_col_wise=true` to remove the overhead.
[LightGBM] [Info] Total Bins 250
[LightGBM] [Info] Number of data points in the train set: 750, number of used features: 1
[LightGBM] [Info] Start training from score 155.379656
[LightGBM] [Warning] No further splits with positive gain, best gain: -inf
[LightGBM] [Warning] No further splits with positive gain, best gain: -inf
[LightGBM] [Warning] No further splits with positive gain, best gain: -inf
[LightGBM] [Warning] No further splits with positive gain, best gain: -inf
[LightGBM] [Warning] No further splits with positive gain, best gain: -inf
[LightGBM] [Warning] No further splits with positive gain, best gain: -inf
[LightGBM] [Warning] No further splits with positive gain, best gain: -inf
[LightGBM] [Warning] No further splits with positive gain, best gain: -inf
[LightGBM] [Warning] No further splits with positive gain, best gain: -inf
[LightGBM] [Warning] No further splits with positive gain, best gain: -inf
[LightGBM] [Warning] No further splits with positive gain, best gain: -inf
[LightGBM] [Warning] No further splits with positive gain, best gain: -inf
[LightGBM] [Warning] No further splits with positive gain, best gain: -inf
[LightGBM] [Warning] No further splits with positive gain, best gain: -inf
[LightGBM] [Warning] No further splits with positive gain, best gain: -inf
[LightGBM] [Warning] No further splits with positive gain, best gain: -inf
[LightGBM] [Warning] No further splits with positive gain, best gain: -inf
[LightGBM] [Warning] No further splits with positive gain, best gain: -inf
[LightGBM] [Warning] No further splits with positive gain, best gain: -inf
[LightGBM] [Warning] No further splits with positive gain, best gain: -inf
[LightGBM] [Warning] Accuracy may be bad since you didn't explicitly set num_leaves OR 2^max_depth > num_leaves. (num_leaves=31).
[LightGBM] [Warning] Accuracy may be bad since you didn't explicitly set num_leaves OR 2^max_depth > num_leaves. (num_leaves=31).
[LightGBM] [Warning] Accuracy may be bad since you didn't explicitly set num_leaves OR 2^max_depth > num_leaves. (num_leaves=31).
[LightGBM] [Info] Auto-choosing col-wise multi-threading, the overhead of testing was 0.000043 seconds.
You can set `force_col_wise=true` to remove the overhead.
[LightGBM] [Info] Total Bins 250
[LightGBM] [Info] Number of data points in the train set: 750, number of used features: 1
[LightGBM] [Info] Start training from score 155.051009
[LightGBM] [Warning] No further splits with positive gain, best gain: -inf
[LightGBM] [Warning] No further splits with positive gain, best gain: -inf
[LightGBM] [Warning] No further splits with positive gain, best gain: -inf
[LightGBM] [Warning] No further splits with positive gain, best gain: -inf
[LightGBM] [Warning] No further splits with positive gain, best gain: -inf
[LightGBM] [Warning] No further splits with positive gain, best gain: -inf
[LightGBM] [Warning] No further splits with positive gain, best gain: -inf
[LightGBM] [Warning] No further splits with positive gain, best gain: -inf
[LightGBM] [Warning] No further splits with positive gain, best gain: -inf
[LightGBM] [Warning] No further splits with positive gain, best gain: -inf
[LightGBM] [Warning] No further splits with positive gain, best gain: -inf
[LightGBM] [Warning] No further splits with positive gain, best gain: -inf
[LightGBM] [Warning] No further splits with positive gain, best gain: -inf
[LightGBM] [Warning] No further splits with positive gain, best gain: -inf
[LightGBM] [Warning] No further splits with positive gain, best gain: -inf
[LightGBM] [Warning] No further splits with positive gain, best gain: -inf
[LightGBM] [Warning] No further splits with positive gain, best gain: -inf
[LightGBM] [Warning] No further splits with positive gain, best gain: -inf
[LightGBM] [Warning] No further splits with positive gain, best gain: -inf
[LightGBM] [Warning] No further splits with positive gain, best gain: -inf
[LightGBM] [Warning] Accuracy may be bad since you didn't explicitly set num_leaves OR 2^max_depth > num_leaves. (num_leaves=31).
[LightGBM] [Warning] Accuracy may be bad since you didn't explicitly set num_leaves OR 2^max_depth > num_leaves. (num_leaves=31).
[LightGBM] [Warning] Accuracy may be bad since you didn't explicitly set num_leaves OR 2^max_depth > num_leaves. (num_leaves=31).
[LightGBM] [Info] Auto-choosing col-wise multi-threading, the overhead of testing was 0.000043 seconds.
You can set `force_col_wise=true` to remove the overhead.
[LightGBM] [Info] Total Bins 250
[LightGBM] [Info] Number of data points in the train set: 750, number of used features: 1
[LightGBM] [Info] Start training from score 155.051009
[LightGBM] [Warning] No further splits with positive gain, best gain: -inf
[LightGBM] [Warning] No further splits with positive gain, best gain: -inf
[LightGBM] [Warning] No further splits with positive gain, best gain: -inf
[LightGBM] [Warning] No further splits with positive gain, best gain: -inf
[LightGBM] [Warning] No further splits with positive gain, best gain: -inf
[LightGBM] [Warning] No further splits with positive gain, best gain: -inf
[LightGBM] [Warning] No further splits with positive gain, best gain: -inf
[LightGBM] [Warning] No further splits with positive gain, best gain: -inf
[LightGBM] [Warning] No further splits with positive gain, best gain: -inf
[LightGBM] [Warning] No further splits with positive gain, best gain: -inf
[LightGBM] [Warning] No further splits with positive gain, best gain: -inf
[LightGBM] [Warning] No further splits with positive gain, best gain: -inf
[LightGBM] [Warning] No further splits with positive gain, best gain: -inf
[LightGBM] [Warning] No further splits with positive gain, best gain: -inf
[LightGBM] [Warning] No further splits with positive gain, best gain: -inf
[LightGBM] [Warning] No further splits with positive gain, best gain: -inf
[LightGBM] [Warning] No further splits with positive gain, best gain: -inf
[LightGBM] [Warning] No further splits with positive gain, best gain: -inf
[LightGBM] [Warning] No further splits with positive gain, best gain: -inf
[LightGBM] [Warning] No further splits with positive gain, best gain: -inf
[LightGBM] [Warning] Accuracy may be bad since you didn't explicitly set num_leaves OR 2^max_depth > num_leaves. (num_leaves=31).
[LightGBM] [Warning] Accuracy may be bad since you didn't explicitly set num_leaves OR 2^max_depth > num_leaves. (num_leaves=31).
[LightGBM] [Warning] Accuracy may be bad since you didn't explicitly set num_leaves OR 2^max_depth > num_leaves. (num_leaves=31).
[LightGBM] [Info] Auto-choosing col-wise multi-threading, the overhead of testing was 0.000033 seconds.
You can set `force_col_wise=true` to remove the overhead.
[LightGBM] [Info] Total Bins 250
[LightGBM] [Info] Number of data points in the train set: 750, number of used features: 1
[LightGBM] [Info] Start training from score 155.051009
[LightGBM] [Warning] No further splits with positive gain, best gain: -inf
[LightGBM] [Warning] No further splits with positive gain, best gain: -inf
[LightGBM] [Warning] No further splits with positive gain, best gain: -inf
[LightGBM] [Warning] No further splits with positive gain, best gain: -inf
[LightGBM] [Warning] No further splits with positive gain, best gain: -inf
[LightGBM] [Warning] No further splits with positive gain, best gain: -inf
[LightGBM] [Warning] No further splits with positive gain, best gain: -inf
[LightGBM] [Warning] No further splits with positive gain, best gain: -inf
[LightGBM] [Warning] No further splits with positive gain, best gain: -inf
[LightGBM] [Warning] No further splits with positive gain, best gain: -inf
[LightGBM] [Warning] No further splits with positive gain, best gain: -inf
[LightGBM] [Warning] No further splits with positive gain, best gain: -inf
[LightGBM] [Warning] No further splits with positive gain, best gain: -inf
[LightGBM] [Warning] No further splits with positive gain, best gain: -inf
[LightGBM] [Warning] No further splits with positive gain, best gain: -inf
[LightGBM] [Warning] No further splits with positive gain, best gain: -inf
[LightGBM] [Warning] No further splits with positive gain, best gain: -inf
[LightGBM] [Warning] No further splits with positive gain, best gain: -inf
[LightGBM] [Warning] No further splits with positive gain, best gain: -inf
[LightGBM] [Warning] No further splits with positive gain, best gain: -inf
60%|██████ | 15/25 [00:01<00:01, 8.81it/s]
[LightGBM] [Warning] Accuracy may be bad since you didn't explicitly set num_leaves OR 2^max_depth > num_leaves. (num_leaves=31).
[LightGBM] [Warning] Accuracy may be bad since you didn't explicitly set num_leaves OR 2^max_depth > num_leaves. (num_leaves=31).
[LightGBM] [Warning] Accuracy may be bad since you didn't explicitly set num_leaves OR 2^max_depth > num_leaves. (num_leaves=31).
[LightGBM] [Info] Auto-choosing row-wise multi-threading, the overhead of testing was 0.000043 seconds.
You can set `force_row_wise=true` to remove the overhead.
And if memory is not enough, you can set `force_col_wise=true`.
[LightGBM] [Info] Total Bins 250
[LightGBM] [Info] Number of data points in the train set: 750, number of used features: 1
[LightGBM] [Info] Start training from score 155.140242
[LightGBM] [Warning] No further splits with positive gain, best gain: -inf
[LightGBM] [Warning] No further splits with positive gain, best gain: -inf
[LightGBM] [Warning] No further splits with positive gain, best gain: -inf
[LightGBM] [Warning] No further splits with positive gain, best gain: -inf
[LightGBM] [Warning] No further splits with positive gain, best gain: -inf
[LightGBM] [Warning] No further splits with positive gain, best gain: -inf
[LightGBM] [Warning] No further splits with positive gain, best gain: -inf
[LightGBM] [Warning] No further splits with positive gain, best gain: -inf
[LightGBM] [Warning] No further splits with positive gain, best gain: -inf
[LightGBM] [Warning] No further splits with positive gain, best gain: -inf
[LightGBM] [Warning] No further splits with positive gain, best gain: -inf
[LightGBM] [Warning] No further splits with positive gain, best gain: -inf
[LightGBM] [Warning] No further splits with positive gain, best gain: -inf
[LightGBM] [Warning] No further splits with positive gain, best gain: -inf
[LightGBM] [Warning] No further splits with positive gain, best gain: -inf
[LightGBM] [Warning] No further splits with positive gain, best gain: -inf
[LightGBM] [Warning] No further splits with positive gain, best gain: -inf
[LightGBM] [Warning] No further splits with positive gain, best gain: -inf
[LightGBM] [Warning] No further splits with positive gain, best gain: -inf
[LightGBM] [Warning] No further splits with positive gain, best gain: -inf
[LightGBM] [Warning] Accuracy may be bad since you didn't explicitly set num_leaves OR 2^max_depth > num_leaves. (num_leaves=31).
[LightGBM] [Warning] Accuracy may be bad since you didn't explicitly set num_leaves OR 2^max_depth > num_leaves. (num_leaves=31).
[LightGBM] [Warning] Accuracy may be bad since you didn't explicitly set num_leaves OR 2^max_depth > num_leaves. (num_leaves=31).
[LightGBM] [Info] Auto-choosing col-wise multi-threading, the overhead of testing was 0.000042 seconds.
You can set `force_col_wise=true` to remove the overhead.
[LightGBM] [Info] Total Bins 250
[LightGBM] [Info] Number of data points in the train set: 750, number of used features: 1
[LightGBM] [Info] Start training from score 155.140242
[LightGBM] [Warning] No further splits with positive gain, best gain: -inf
[LightGBM] [Warning] No further splits with positive gain, best gain: -inf
[LightGBM] [Warning] No further splits with positive gain, best gain: -inf
[LightGBM] [Warning] No further splits with positive gain, best gain: -inf
[LightGBM] [Warning] No further splits with positive gain, best gain: -inf
[LightGBM] [Warning] No further splits with positive gain, best gain: -inf
[LightGBM] [Warning] No further splits with positive gain, best gain: -inf
[LightGBM] [Warning] No further splits with positive gain, best gain: -inf
[LightGBM] [Warning] No further splits with positive gain, best gain: -inf
[LightGBM] [Warning] No further splits with positive gain, best gain: -inf
[LightGBM] [Warning] No further splits with positive gain, best gain: -inf
[LightGBM] [Warning] No further splits with positive gain, best gain: -inf
[LightGBM] [Warning] No further splits with positive gain, best gain: -inf
[LightGBM] [Warning] No further splits with positive gain, best gain: -inf
[LightGBM] [Warning] No further splits with positive gain, best gain: -inf
[LightGBM] [Warning] No further splits with positive gain, best gain: -inf
[LightGBM] [Warning] No further splits with positive gain, best gain: -inf
[LightGBM] [Warning] No further splits with positive gain, best gain: -inf
[LightGBM] [Warning] No further splits with positive gain, best gain: -inf
[LightGBM] [Warning] No further splits with positive gain, best gain: -inf
[LightGBM] [Warning] Accuracy may be bad since you didn't explicitly set num_leaves OR 2^max_depth > num_leaves. (num_leaves=31).
[LightGBM] [Warning] Accuracy may be bad since you didn't explicitly set num_leaves OR 2^max_depth > num_leaves. (num_leaves=31).
[LightGBM] [Warning] Accuracy may be bad since you didn't explicitly set num_leaves OR 2^max_depth > num_leaves. (num_leaves=31).
[LightGBM] [Info] Auto-choosing col-wise multi-threading, the overhead of testing was 0.000077 seconds.
You can set `force_col_wise=true` to remove the overhead.
[LightGBM] [Info] Total Bins 250
[LightGBM] [Info] Number of data points in the train set: 750, number of used features: 1
[LightGBM] [Info] Start training from score 155.140242
[LightGBM] [Warning] No further splits with positive gain, best gain: -inf
[LightGBM] [Warning] No further splits with positive gain, best gain: -inf
[LightGBM] [Warning] No further splits with positive gain, best gain: -inf
[LightGBM] [Warning] No further splits with positive gain, best gain: -inf
[LightGBM] [Warning] No further splits with positive gain, best gain: -inf
[LightGBM] [Warning] No further splits with positive gain, best gain: -inf
[LightGBM] [Warning] No further splits with positive gain, best gain: -inf
[LightGBM] [Warning] No further splits with positive gain, best gain: -inf
[LightGBM] [Warning] No further splits with positive gain, best gain: -inf
[LightGBM] [Warning] No further splits with positive gain, best gain: -inf
[LightGBM] [Warning] No further splits with positive gain, best gain: -inf
[LightGBM] [Warning] No further splits with positive gain, best gain: -inf
[LightGBM] [Warning] No further splits with positive gain, best gain: -inf
[LightGBM] [Warning] No further splits with positive gain, best gain: -inf
[LightGBM] [Warning] No further splits with positive gain, best gain: -inf
[LightGBM] [Warning] No further splits with positive gain, best gain: -inf
[LightGBM] [Warning] No further splits with positive gain, best gain: -inf
[LightGBM] [Warning] No further splits with positive gain, best gain: -inf
[LightGBM] [Warning] No further splits with positive gain, best gain: -inf
[LightGBM] [Warning] No further splits with positive gain, best gain: -inf
[LightGBM] [Warning] Accuracy may be bad since you didn't explicitly set num_leaves OR 2^max_depth > num_leaves. (num_leaves=31).
[LightGBM] [Warning] Accuracy may be bad since you didn't explicitly set num_leaves OR 2^max_depth > num_leaves. (num_leaves=31).
[LightGBM] [Warning] Accuracy may be bad since you didn't explicitly set num_leaves OR 2^max_depth > num_leaves. (num_leaves=31).
[LightGBM] [Info] Auto-choosing col-wise multi-threading, the overhead of testing was 0.000069 seconds.
You can set `force_col_wise=true` to remove the overhead.
[LightGBM] [Info] Total Bins 250
[LightGBM] [Info] Number of data points in the train set: 750, number of used features: 1
[LightGBM] [Info] Start training from score 155.432455
[LightGBM] [Warning] No further splits with positive gain, best gain: -inf
[LightGBM] [Warning] No further splits with positive gain, best gain: -inf
[LightGBM] [Warning] No further splits with positive gain, best gain: -inf
[LightGBM] [Warning] No further splits with positive gain, best gain: -inf
[LightGBM] [Warning] No further splits with positive gain, best gain: -inf
[LightGBM] [Warning] No further splits with positive gain, best gain: -inf
[LightGBM] [Warning] No further splits with positive gain, best gain: -inf
[LightGBM] [Warning] No further splits with positive gain, best gain: -inf
[LightGBM] [Warning] No further splits with positive gain, best gain: -inf
[LightGBM] [Warning] No further splits with positive gain, best gain: -inf
[LightGBM] [Warning] No further splits with positive gain, best gain: -inf
[LightGBM] [Warning] No further splits with positive gain, best gain: -inf
[LightGBM] [Warning] No further splits with positive gain, best gain: -inf
[LightGBM] [Warning] No further splits with positive gain, best gain: -inf
[LightGBM] [Warning] No further splits with positive gain, best gain: -inf
[LightGBM] [Warning] No further splits with positive gain, best gain: -inf
[LightGBM] [Warning] No further splits with positive gain, best gain: -inf
[LightGBM] [Warning] No further splits with positive gain, best gain: -inf
[LightGBM] [Warning] No further splits with positive gain, best gain: -inf
[LightGBM] [Warning] No further splits with positive gain, best gain: -inf
[LightGBM] [Warning] Accuracy may be bad since you didn't explicitly set num_leaves OR 2^max_depth > num_leaves. (num_leaves=31).
[LightGBM] [Warning] Accuracy may be bad since you didn't explicitly set num_leaves OR 2^max_depth > num_leaves. (num_leaves=31).
[LightGBM] [Warning] Accuracy may be bad since you didn't explicitly set num_leaves OR 2^max_depth > num_leaves. (num_leaves=31).
[LightGBM] [Info] Auto-choosing col-wise multi-threading, the overhead of testing was 0.000068 seconds.
You can set `force_col_wise=true` to remove the overhead.
[LightGBM] [Info] Total Bins 250
[LightGBM] [Info] Number of data points in the train set: 750, number of used features: 1
[LightGBM] [Info] Start training from score 155.432455
[LightGBM] [Warning] No further splits with positive gain, best gain: -inf
[LightGBM] [Warning] No further splits with positive gain, best gain: -inf
[LightGBM] [Warning] No further splits with positive gain, best gain: -inf
[LightGBM] [Warning] No further splits with positive gain, best gain: -inf
[LightGBM] [Warning] No further splits with positive gain, best gain: -inf
[LightGBM] [Warning] No further splits with positive gain, best gain: -inf
[LightGBM] [Warning] No further splits with positive gain, best gain: -inf
[LightGBM] [Warning] No further splits with positive gain, best gain: -inf
[LightGBM] [Warning] No further splits with positive gain, best gain: -inf
[LightGBM] [Warning] No further splits with positive gain, best gain: -inf
[LightGBM] [Warning] No further splits with positive gain, best gain: -inf
[LightGBM] [Warning] No further splits with positive gain, best gain: -inf
[LightGBM] [Warning] No further splits with positive gain, best gain: -inf
[LightGBM] [Warning] No further splits with positive gain, best gain: -inf
[LightGBM] [Warning] No further splits with positive gain, best gain: -inf
[LightGBM] [Warning] No further splits with positive gain, best gain: -inf
[LightGBM] [Warning] No further splits with positive gain, best gain: -inf
[LightGBM] [Warning] No further splits with positive gain, best gain: -inf
[LightGBM] [Warning] No further splits with positive gain, best gain: -inf
[LightGBM] [Warning] No further splits with positive gain, best gain: -inf
[LightGBM] [Warning] Accuracy may be bad since you didn't explicitly set num_leaves OR 2^max_depth > num_leaves. (num_leaves=31).
72%|███████▏ | 18/25 [00:01<00:00, 8.19it/s]
[LightGBM] [Warning] Accuracy may be bad since you didn't explicitly set num_leaves OR 2^max_depth > num_leaves. (num_leaves=31).
[LightGBM] [Warning] Accuracy may be bad since you didn't explicitly set num_leaves OR 2^max_depth > num_leaves. (num_leaves=31).
[LightGBM] [Info] Auto-choosing col-wise multi-threading, the overhead of testing was 0.000070 seconds.
You can set `force_col_wise=true` to remove the overhead.
[LightGBM] [Info] Total Bins 250
[LightGBM] [Info] Number of data points in the train set: 750, number of used features: 1
[LightGBM] [Info] Start training from score 155.432455
[LightGBM] [Warning] No further splits with positive gain, best gain: -inf
[LightGBM] [Warning] No further splits with positive gain, best gain: -inf
[LightGBM] [Warning] No further splits with positive gain, best gain: -inf
[LightGBM] [Warning] No further splits with positive gain, best gain: -inf
[LightGBM] [Warning] No further splits with positive gain, best gain: -inf
[LightGBM] [Warning] No further splits with positive gain, best gain: -inf
[LightGBM] [Warning] No further splits with positive gain, best gain: -inf
[LightGBM] [Warning] No further splits with positive gain, best gain: -inf
[LightGBM] [Warning] No further splits with positive gain, best gain: -inf
[LightGBM] [Warning] No further splits with positive gain, best gain: -inf
[LightGBM] [Warning] No further splits with positive gain, best gain: -inf
[LightGBM] [Warning] No further splits with positive gain, best gain: -inf
[LightGBM] [Warning] No further splits with positive gain, best gain: -inf
[LightGBM] [Warning] No further splits with positive gain, best gain: -inf
[LightGBM] [Warning] No further splits with positive gain, best gain: -inf
[LightGBM] [Warning] No further splits with positive gain, best gain: -inf
[LightGBM] [Warning] No further splits with positive gain, best gain: -inf
[LightGBM] [Warning] No further splits with positive gain, best gain: -inf
[LightGBM] [Warning] No further splits with positive gain, best gain: -inf
[LightGBM] [Warning] No further splits with positive gain, best gain: -inf
[LightGBM] [Warning] Accuracy may be bad since you didn't explicitly set num_leaves OR 2^max_depth > num_leaves. (num_leaves=31).
[LightGBM] [Warning] Accuracy may be bad since you didn't explicitly set num_leaves OR 2^max_depth > num_leaves. (num_leaves=31).
[LightGBM] [Warning] Accuracy may be bad since you didn't explicitly set num_leaves OR 2^max_depth > num_leaves. (num_leaves=31).
[LightGBM] [Info] Auto-choosing col-wise multi-threading, the overhead of testing was 0.000085 seconds.
You can set `force_col_wise=true` to remove the overhead.
[LightGBM] [Info] Total Bins 250
[LightGBM] [Info] Number of data points in the train set: 750, number of used features: 1
[LightGBM] [Info] Start training from score 155.319151
[LightGBM] [Warning] No further splits with positive gain, best gain: -inf
[LightGBM] [Warning] No further splits with positive gain, best gain: -inf
[LightGBM] [Warning] No further splits with positive gain, best gain: -inf
[LightGBM] [Warning] No further splits with positive gain, best gain: -inf
[LightGBM] [Warning] No further splits with positive gain, best gain: -inf
[LightGBM] [Warning] No further splits with positive gain, best gain: -inf
[LightGBM] [Warning] No further splits with positive gain, best gain: -inf
[LightGBM] [Warning] No further splits with positive gain, best gain: -inf
[LightGBM] [Warning] No further splits with positive gain, best gain: -inf
[LightGBM] [Warning] No further splits with positive gain, best gain: -inf
[LightGBM] [Warning] No further splits with positive gain, best gain: -inf
[LightGBM] [Warning] No further splits with positive gain, best gain: -inf
[LightGBM] [Warning] No further splits with positive gain, best gain: -inf
[LightGBM] [Warning] No further splits with positive gain, best gain: -inf
[LightGBM] [Warning] No further splits with positive gain, best gain: -inf
[LightGBM] [Warning] No further splits with positive gain, best gain: -inf
[LightGBM] [Warning] No further splits with positive gain, best gain: -inf
[LightGBM] [Warning] No further splits with positive gain, best gain: -inf
[LightGBM] [Warning] No further splits with positive gain, best gain: -inf
[LightGBM] [Warning] No further splits with positive gain, best gain: -inf
[LightGBM] [Warning] Accuracy may be bad since you didn't explicitly set num_leaves OR 2^max_depth > num_leaves. (num_leaves=31).
[LightGBM] [Warning] Accuracy may be bad since you didn't explicitly set num_leaves OR 2^max_depth > num_leaves. (num_leaves=31).
[LightGBM] [Warning] Accuracy may be bad since you didn't explicitly set num_leaves OR 2^max_depth > num_leaves. (num_leaves=31).
[LightGBM] [Info] Auto-choosing row-wise multi-threading, the overhead of testing was 0.000059 seconds.
You can set `force_row_wise=true` to remove the overhead.
And if memory is not enough, you can set `force_col_wise=true`.
[LightGBM] [Info] Total Bins 250
[LightGBM] [Info] Number of data points in the train set: 750, number of used features: 1
[LightGBM] [Info] Start training from score 155.319151
[LightGBM] [Warning] No further splits with positive gain, best gain: -inf
[LightGBM] [Warning] No further splits with positive gain, best gain: -inf
[LightGBM] [Warning] No further splits with positive gain, best gain: -inf
[LightGBM] [Warning] No further splits with positive gain, best gain: -inf
[LightGBM] [Warning] No further splits with positive gain, best gain: -inf
[LightGBM] [Warning] No further splits with positive gain, best gain: -inf
[LightGBM] [Warning] No further splits with positive gain, best gain: -inf
[LightGBM] [Warning] No further splits with positive gain, best gain: -inf
[LightGBM] [Warning] No further splits with positive gain, best gain: -inf
[LightGBM] [Warning] No further splits with positive gain, best gain: -inf
[LightGBM] [Warning] No further splits with positive gain, best gain: -inf
[LightGBM] [Warning] No further splits with positive gain, best gain: -inf
[LightGBM] [Warning] No further splits with positive gain, best gain: -inf
[LightGBM] [Warning] No further splits with positive gain, best gain: -inf
[LightGBM] [Warning] No further splits with positive gain, best gain: -inf
[LightGBM] [Warning] No further splits with positive gain, best gain: -inf
[LightGBM] [Warning] No further splits with positive gain, best gain: -inf
[LightGBM] [Warning] No further splits with positive gain, best gain: -inf
[LightGBM] [Warning] No further splits with positive gain, best gain: -inf
[LightGBM] [Warning] No further splits with positive gain, best gain: -inf
[LightGBM] [Warning] Accuracy may be bad since you didn't explicitly set num_leaves OR 2^max_depth > num_leaves. (num_leaves=31).
[LightGBM] [Warning] Accuracy may be bad since you didn't explicitly set num_leaves OR 2^max_depth > num_leaves. (num_leaves=31).
[LightGBM] [Warning] Accuracy may be bad since you didn't explicitly set num_leaves OR 2^max_depth > num_leaves. (num_leaves=31).
[LightGBM] [Info] Auto-choosing col-wise multi-threading, the overhead of testing was 0.000041 seconds.
You can set `force_col_wise=true` to remove the overhead.
[LightGBM] [Info] Total Bins 250
[LightGBM] [Info] Number of data points in the train set: 750, number of used features: 1
[LightGBM] [Info] Start training from score 155.319151
[LightGBM] [Warning] No further splits with positive gain, best gain: -inf
[LightGBM] [Warning] No further splits with positive gain, best gain: -inf
[LightGBM] [Warning] No further splits with positive gain, best gain: -inf
[LightGBM] [Warning] No further splits with positive gain, best gain: -inf
[LightGBM] [Warning] No further splits with positive gain, best gain: -inf
[LightGBM] [Warning] No further splits with positive gain, best gain: -inf
[LightGBM] [Warning] No further splits with positive gain, best gain: -inf
[LightGBM] [Warning] No further splits with positive gain, best gain: -inf
[LightGBM] [Warning] No further splits with positive gain, best gain: -inf
[LightGBM] [Warning] No further splits with positive gain, best gain: -inf
[LightGBM] [Warning] No further splits with positive gain, best gain: -inf
[LightGBM] [Warning] No further splits with positive gain, best gain: -inf
[LightGBM] [Warning] No further splits with positive gain, best gain: -inf
[LightGBM] [Warning] No further splits with positive gain, best gain: -inf
[LightGBM] [Warning] No further splits with positive gain, best gain: -inf
[LightGBM] [Warning] No further splits with positive gain, best gain: -inf
[LightGBM] [Warning] No further splits with positive gain, best gain: -inf
[LightGBM] [Warning] No further splits with positive gain, best gain: -inf
[LightGBM] [Warning] No further splits with positive gain, best gain: -inf
[LightGBM] [Warning] No further splits with positive gain, best gain: -inf
[LightGBM] [Warning] Accuracy may be bad since you didn't explicitly set num_leaves OR 2^max_depth > num_leaves. (num_leaves=31).
80%|████████ | 20/25 [00:02<00:00, 9.29it/s]
[LightGBM] [Warning] Accuracy may be bad since you didn't explicitly set num_leaves OR 2^max_depth > num_leaves. (num_leaves=31).
[LightGBM] [Warning] Accuracy may be bad since you didn't explicitly set num_leaves OR 2^max_depth > num_leaves. (num_leaves=31).
[LightGBM] [Info] Auto-choosing col-wise multi-threading, the overhead of testing was 0.000043 seconds.
You can set `force_col_wise=true` to remove the overhead.
[LightGBM] [Info] Total Bins 250
[LightGBM] [Info] Number of data points in the train set: 750, number of used features: 1
[LightGBM] [Info] Start training from score 155.163655
[LightGBM] [Warning] No further splits with positive gain, best gain: -inf
[LightGBM] [Warning] No further splits with positive gain, best gain: -inf
[LightGBM] [Warning] No further splits with positive gain, best gain: -inf
[LightGBM] [Warning] No further splits with positive gain, best gain: -inf
[LightGBM] [Warning] No further splits with positive gain, best gain: -inf
[LightGBM] [Warning] No further splits with positive gain, best gain: -inf
[LightGBM] [Warning] No further splits with positive gain, best gain: -inf
[LightGBM] [Warning] No further splits with positive gain, best gain: -inf
[LightGBM] [Warning] No further splits with positive gain, best gain: -inf
[LightGBM] [Warning] No further splits with positive gain, best gain: -inf
[LightGBM] [Warning] No further splits with positive gain, best gain: -inf
[LightGBM] [Warning] No further splits with positive gain, best gain: -inf
[LightGBM] [Warning] No further splits with positive gain, best gain: -inf
[LightGBM] [Warning] No further splits with positive gain, best gain: -inf
[LightGBM] [Warning] No further splits with positive gain, best gain: -inf
[LightGBM] [Warning] No further splits with positive gain, best gain: -inf
[LightGBM] [Warning] No further splits with positive gain, best gain: -inf
[LightGBM] [Warning] No further splits with positive gain, best gain: -inf
[LightGBM] [Warning] No further splits with positive gain, best gain: -inf
[LightGBM] [Warning] No further splits with positive gain, best gain: -inf
[LightGBM] [Warning] Accuracy may be bad since you didn't explicitly set num_leaves OR 2^max_depth > num_leaves. (num_leaves=31).
[LightGBM] [Warning] Accuracy may be bad since you didn't explicitly set num_leaves OR 2^max_depth > num_leaves. (num_leaves=31).
[LightGBM] [Warning] Accuracy may be bad since you didn't explicitly set num_leaves OR 2^max_depth > num_leaves. (num_leaves=31).
[LightGBM] [Info] Auto-choosing col-wise multi-threading, the overhead of testing was 0.000042 seconds.
You can set `force_col_wise=true` to remove the overhead.
[LightGBM] [Info] Total Bins 250
[LightGBM] [Info] Number of data points in the train set: 750, number of used features: 1
[LightGBM] [Info] Start training from score 155.163655
[LightGBM] [Warning] No further splits with positive gain, best gain: -inf
[LightGBM] [Warning] No further splits with positive gain, best gain: -inf
[LightGBM] [Warning] No further splits with positive gain, best gain: -inf
[LightGBM] [Warning] No further splits with positive gain, best gain: -inf
[LightGBM] [Warning] No further splits with positive gain, best gain: -inf
[LightGBM] [Warning] No further splits with positive gain, best gain: -inf
[LightGBM] [Warning] No further splits with positive gain, best gain: -inf
[LightGBM] [Warning] No further splits with positive gain, best gain: -inf
[LightGBM] [Warning] No further splits with positive gain, best gain: -inf
[LightGBM] [Warning] No further splits with positive gain, best gain: -inf
[LightGBM] [Warning] No further splits with positive gain, best gain: -inf
[LightGBM] [Warning] No further splits with positive gain, best gain: -inf
[LightGBM] [Warning] No further splits with positive gain, best gain: -inf
[LightGBM] [Warning] No further splits with positive gain, best gain: -inf
[LightGBM] [Warning] No further splits with positive gain, best gain: -inf
[LightGBM] [Warning] No further splits with positive gain, best gain: -inf
[LightGBM] [Warning] No further splits with positive gain, best gain: -inf
[LightGBM] [Warning] No further splits with positive gain, best gain: -inf
[LightGBM] [Warning] No further splits with positive gain, best gain: -inf
[LightGBM] [Warning] No further splits with positive gain, best gain: -inf
[LightGBM] [Warning] Accuracy may be bad since you didn't explicitly set num_leaves OR 2^max_depth > num_leaves. (num_leaves=31).
[LightGBM] [Warning] Accuracy may be bad since you didn't explicitly set num_leaves OR 2^max_depth > num_leaves. (num_leaves=31).
[LightGBM] [Warning] Accuracy may be bad since you didn't explicitly set num_leaves OR 2^max_depth > num_leaves. (num_leaves=31).
[LightGBM] [Info] Auto-choosing col-wise multi-threading, the overhead of testing was 0.000045 seconds.
You can set `force_col_wise=true` to remove the overhead.
[LightGBM] [Info] Total Bins 250
[LightGBM] [Info] Number of data points in the train set: 750, number of used features: 1
[LightGBM] [Info] Start training from score 155.163655
[LightGBM] [Warning] No further splits with positive gain, best gain: -inf
[LightGBM] [Warning] No further splits with positive gain, best gain: -inf
[LightGBM] [Warning] No further splits with positive gain, best gain: -inf
[LightGBM] [Warning] No further splits with positive gain, best gain: -inf
[LightGBM] [Warning] No further splits with positive gain, best gain: -inf
[LightGBM] [Warning] No further splits with positive gain, best gain: -inf
[LightGBM] [Warning] No further splits with positive gain, best gain: -inf
[LightGBM] [Warning] No further splits with positive gain, best gain: -inf
[LightGBM] [Warning] No further splits with positive gain, best gain: -inf
[LightGBM] [Warning] No further splits with positive gain, best gain: -inf
[LightGBM] [Warning] No further splits with positive gain, best gain: -inf
[LightGBM] [Warning] No further splits with positive gain, best gain: -inf
[LightGBM] [Warning] No further splits with positive gain, best gain: -inf
[LightGBM] [Warning] No further splits with positive gain, best gain: -inf
[LightGBM] [Warning] No further splits with positive gain, best gain: -inf
[LightGBM] [Warning] No further splits with positive gain, best gain: -inf
[LightGBM] [Warning] No further splits with positive gain, best gain: -inf
[LightGBM] [Warning] No further splits with positive gain, best gain: -inf
[LightGBM] [Warning] No further splits with positive gain, best gain: -inf
[LightGBM] [Warning] No further splits with positive gain, best gain: -inf
[LightGBM] [Warning] Accuracy may be bad since you didn't explicitly set num_leaves OR 2^max_depth > num_leaves. (num_leaves=31).
[LightGBM] [Warning] Accuracy may be bad since you didn't explicitly set num_leaves OR 2^max_depth > num_leaves. (num_leaves=31).
[LightGBM] [Warning] Accuracy may be bad since you didn't explicitly set num_leaves OR 2^max_depth > num_leaves. (num_leaves=31).
[LightGBM] [Info] Auto-choosing col-wise multi-threading, the overhead of testing was 0.000036 seconds.
You can set `force_col_wise=true` to remove the overhead.
[LightGBM] [Info] Total Bins 251
[LightGBM] [Info] Number of data points in the train set: 750, number of used features: 1
[LightGBM] [Info] Start training from score 155.329555
[LightGBM] [Warning] No further splits with positive gain, best gain: -inf
[LightGBM] [Warning] No further splits with positive gain, best gain: -inf
[LightGBM] [Warning] No further splits with positive gain, best gain: -inf
[LightGBM] [Warning] No further splits with positive gain, best gain: -inf
[LightGBM] [Warning] No further splits with positive gain, best gain: -inf
[LightGBM] [Warning] No further splits with positive gain, best gain: -inf
[LightGBM] [Warning] No further splits with positive gain, best gain: -inf
[LightGBM] [Warning] No further splits with positive gain, best gain: -inf
[LightGBM] [Warning] No further splits with positive gain, best gain: -inf
[LightGBM] [Warning] No further splits with positive gain, best gain: -inf
[LightGBM] [Warning] No further splits with positive gain, best gain: -inf
[LightGBM] [Warning] No further splits with positive gain, best gain: -inf
[LightGBM] [Warning] No further splits with positive gain, best gain: -inf
[LightGBM] [Warning] No further splits with positive gain, best gain: -inf
[LightGBM] [Warning] No further splits with positive gain, best gain: -inf
[LightGBM] [Warning] No further splits with positive gain, best gain: -inf
[LightGBM] [Warning] No further splits with positive gain, best gain: -inf
[LightGBM] [Warning] No further splits with positive gain, best gain: -inf
[LightGBM] [Warning] No further splits with positive gain, best gain: -inf
[LightGBM] [Warning] No further splits with positive gain, best gain: -inf
[LightGBM] [Warning] Accuracy may be bad since you didn't explicitly set num_leaves OR 2^max_depth > num_leaves. (num_leaves=31).
[LightGBM] [Warning] Accuracy may be bad since you didn't explicitly set num_leaves OR 2^max_depth > num_leaves. (num_leaves=31).
[LightGBM] [Warning] Accuracy may be bad since you didn't explicitly set num_leaves OR 2^max_depth > num_leaves. (num_leaves=31).
[LightGBM] [Info] Auto-choosing col-wise multi-threading, the overhead of testing was 0.000044 seconds.
You can set `force_col_wise=true` to remove the overhead.
[LightGBM] [Info] Total Bins 251
[LightGBM] [Info] Number of data points in the train set: 750, number of used features: 1
[LightGBM] [Info] Start training from score 155.329555
[LightGBM] [Warning] No further splits with positive gain, best gain: -inf
[LightGBM] [Warning] No further splits with positive gain, best gain: -inf
[LightGBM] [Warning] No further splits with positive gain, best gain: -inf
[LightGBM] [Warning] No further splits with positive gain, best gain: -inf
[LightGBM] [Warning] No further splits with positive gain, best gain: -inf
[LightGBM] [Warning] No further splits with positive gain, best gain: -inf
[LightGBM] [Warning] No further splits with positive gain, best gain: -inf
[LightGBM] [Warning] No further splits with positive gain, best gain: -inf
[LightGBM] [Warning] No further splits with positive gain, best gain: -inf
[LightGBM] [Warning] No further splits with positive gain, best gain: -inf
[LightGBM] [Warning] No further splits with positive gain, best gain: -inf
[LightGBM] [Warning] No further splits with positive gain, best gain: -inf
[LightGBM] [Warning] No further splits with positive gain, best gain: -inf
[LightGBM] [Warning] No further splits with positive gain, best gain: -inf
[LightGBM] [Warning] No further splits with positive gain, best gain: -inf
[LightGBM] [Warning] No further splits with positive gain, best gain: -inf
[LightGBM] [Warning] No further splits with positive gain, best gain: -inf
[LightGBM] [Warning] No further splits with positive gain, best gain: -inf
[LightGBM] [Warning] No further splits with positive gain, best gain: -inf
[LightGBM] [Warning] No further splits with positive gain, best gain: -inf
[LightGBM] [Warning] Accuracy may be bad since you didn't explicitly set num_leaves OR 2^max_depth > num_leaves. (num_leaves=31).
[LightGBM] [Warning] Accuracy may be bad since you didn't explicitly set num_leaves OR 2^max_depth > num_leaves. (num_leaves=31).
[LightGBM] [Warning] Accuracy may be bad since you didn't explicitly set num_leaves OR 2^max_depth > num_leaves. (num_leaves=31).
[LightGBM] [Info] Auto-choosing col-wise multi-threading, the overhead of testing was 0.000041 seconds.
You can set `force_col_wise=true` to remove the overhead.
[LightGBM] [Info] Total Bins 251
[LightGBM] [Info] Number of data points in the train set: 750, number of used features: 1
[LightGBM] [Info] Start training from score 155.329555
[LightGBM] [Warning] No further splits with positive gain, best gain: -inf
[LightGBM] [Warning] No further splits with positive gain, best gain: -inf
[LightGBM] [Warning] No further splits with positive gain, best gain: -inf
[LightGBM] [Warning] No further splits with positive gain, best gain: -inf
[LightGBM] [Warning] No further splits with positive gain, best gain: -inf
[LightGBM] [Warning] No further splits with positive gain, best gain: -inf
[LightGBM] [Warning] No further splits with positive gain, best gain: -inf
[LightGBM] [Warning] No further splits with positive gain, best gain: -inf
[LightGBM] [Warning] No further splits with positive gain, best gain: -inf
[LightGBM] [Warning] No further splits with positive gain, best gain: -inf
[LightGBM] [Warning] No further splits with positive gain, best gain: -inf
[LightGBM] [Warning] No further splits with positive gain, best gain: -inf
[LightGBM] [Warning] No further splits with positive gain, best gain: -inf
[LightGBM] [Warning] No further splits with positive gain, best gain: -inf
[LightGBM] [Warning] No further splits with positive gain, best gain: -inf
[LightGBM] [Warning] No further splits with positive gain, best gain: -inf
[LightGBM] [Warning] No further splits with positive gain, best gain: -inf
[LightGBM] [Warning] No further splits with positive gain, best gain: -inf
[LightGBM] [Warning] No further splits with positive gain, best gain: -inf
[LightGBM] [Warning] No further splits with positive gain, best gain: -inf
[LightGBM] [Warning] Accuracy may be bad since you didn't explicitly set num_leaves OR 2^max_depth > num_leaves. (num_leaves=31).
88%|████████▊ | 22/25 [00:02<00:00, 7.86it/s]
[LightGBM] [Warning] Accuracy may be bad since you didn't explicitly set num_leaves OR 2^max_depth > num_leaves. (num_leaves=31).
[LightGBM] [Warning] Accuracy may be bad since you didn't explicitly set num_leaves OR 2^max_depth > num_leaves. (num_leaves=31).
[LightGBM] [Info] Auto-choosing col-wise multi-threading, the overhead of testing was 0.000046 seconds.
You can set `force_col_wise=true` to remove the overhead.
[LightGBM] [Info] Total Bins 251
[LightGBM] [Info] Number of data points in the train set: 750, number of used features: 1
[LightGBM] [Info] Start training from score 155.444763
[LightGBM] [Warning] No further splits with positive gain, best gain: -inf
[LightGBM] [Warning] No further splits with positive gain, best gain: -inf
[LightGBM] [Warning] No further splits with positive gain, best gain: -inf
[LightGBM] [Warning] No further splits with positive gain, best gain: -inf
[LightGBM] [Warning] No further splits with positive gain, best gain: -inf
[LightGBM] [Warning] No further splits with positive gain, best gain: -inf
[LightGBM] [Warning] No further splits with positive gain, best gain: -inf
[LightGBM] [Warning] No further splits with positive gain, best gain: -inf
[LightGBM] [Warning] No further splits with positive gain, best gain: -inf
[LightGBM] [Warning] No further splits with positive gain, best gain: -inf
[LightGBM] [Warning] No further splits with positive gain, best gain: -inf
[LightGBM] [Warning] No further splits with positive gain, best gain: -inf
[LightGBM] [Warning] No further splits with positive gain, best gain: -inf
[LightGBM] [Warning] No further splits with positive gain, best gain: -inf
[LightGBM] [Warning] No further splits with positive gain, best gain: -inf
[LightGBM] [Warning] No further splits with positive gain, best gain: -inf
[LightGBM] [Warning] No further splits with positive gain, best gain: -inf
[LightGBM] [Warning] No further splits with positive gain, best gain: -inf
[LightGBM] [Warning] No further splits with positive gain, best gain: -inf
[LightGBM] [Warning] No further splits with positive gain, best gain: -inf
[LightGBM] [Warning] Accuracy may be bad since you didn't explicitly set num_leaves OR 2^max_depth > num_leaves. (num_leaves=31).
[LightGBM] [Warning] Accuracy may be bad since you didn't explicitly set num_leaves OR 2^max_depth > num_leaves. (num_leaves=31).
[LightGBM] [Warning] Accuracy may be bad since you didn't explicitly set num_leaves OR 2^max_depth > num_leaves. (num_leaves=31).
[LightGBM] [Info] Auto-choosing col-wise multi-threading, the overhead of testing was 0.000044 seconds.
You can set `force_col_wise=true` to remove the overhead.
[LightGBM] [Info] Total Bins 251
[LightGBM] [Info] Number of data points in the train set: 750, number of used features: 1
[LightGBM] [Info] Start training from score 155.444763
[LightGBM] [Warning] No further splits with positive gain, best gain: -inf
[LightGBM] [Warning] No further splits with positive gain, best gain: -inf
[LightGBM] [Warning] No further splits with positive gain, best gain: -inf
[LightGBM] [Warning] No further splits with positive gain, best gain: -inf
[LightGBM] [Warning] No further splits with positive gain, best gain: -inf
[LightGBM] [Warning] No further splits with positive gain, best gain: -inf
[LightGBM] [Warning] No further splits with positive gain, best gain: -inf
[LightGBM] [Warning] No further splits with positive gain, best gain: -inf
[LightGBM] [Warning] No further splits with positive gain, best gain: -inf
[LightGBM] [Warning] No further splits with positive gain, best gain: -inf
[LightGBM] [Warning] No further splits with positive gain, best gain: -inf
[LightGBM] [Warning] No further splits with positive gain, best gain: -inf
[LightGBM] [Warning] No further splits with positive gain, best gain: -inf
[LightGBM] [Warning] No further splits with positive gain, best gain: -inf
[LightGBM] [Warning] No further splits with positive gain, best gain: -inf
[LightGBM] [Warning] No further splits with positive gain, best gain: -inf
[LightGBM] [Warning] No further splits with positive gain, best gain: -inf
[LightGBM] [Warning] No further splits with positive gain, best gain: -inf
[LightGBM] [Warning] No further splits with positive gain, best gain: -inf
[LightGBM] [Warning] No further splits with positive gain, best gain: -inf
[LightGBM] [Warning] Accuracy may be bad since you didn't explicitly set num_leaves OR 2^max_depth > num_leaves. (num_leaves=31).
[LightGBM] [Warning] Accuracy may be bad since you didn't explicitly set num_leaves OR 2^max_depth > num_leaves. (num_leaves=31).
[LightGBM] [Warning] Accuracy may be bad since you didn't explicitly set num_leaves OR 2^max_depth > num_leaves. (num_leaves=31).
[LightGBM] [Info] Auto-choosing col-wise multi-threading, the overhead of testing was 0.000077 seconds.
You can set `force_col_wise=true` to remove the overhead.
[LightGBM] [Info] Total Bins 251
[LightGBM] [Info] Number of data points in the train set: 750, number of used features: 1
[LightGBM] [Info] Start training from score 155.444763
[LightGBM] [Warning] No further splits with positive gain, best gain: -inf
[LightGBM] [Warning] No further splits with positive gain, best gain: -inf
[LightGBM] [Warning] No further splits with positive gain, best gain: -inf
[LightGBM] [Warning] No further splits with positive gain, best gain: -inf
[LightGBM] [Warning] No further splits with positive gain, best gain: -inf
[LightGBM] [Warning] No further splits with positive gain, best gain: -inf
[LightGBM] [Warning] No further splits with positive gain, best gain: -inf
[LightGBM] [Warning] No further splits with positive gain, best gain: -inf
[LightGBM] [Warning] No further splits with positive gain, best gain: -inf
[LightGBM] [Warning] No further splits with positive gain, best gain: -inf
[LightGBM] [Warning] No further splits with positive gain, best gain: -inf
[LightGBM] [Warning] No further splits with positive gain, best gain: -inf
[LightGBM] [Warning] No further splits with positive gain, best gain: -inf
[LightGBM] [Warning] No further splits with positive gain, best gain: -inf
[LightGBM] [Warning] No further splits with positive gain, best gain: -inf
[LightGBM] [Warning] No further splits with positive gain, best gain: -inf
[LightGBM] [Warning] No further splits with positive gain, best gain: -inf
[LightGBM] [Warning] No further splits with positive gain, best gain: -inf
[LightGBM] [Warning] No further splits with positive gain, best gain: -inf
[LightGBM] [Warning] No further splits with positive gain, best gain: -inf
[LightGBM] [Warning] Accuracy may be bad since you didn't explicitly set num_leaves OR 2^max_depth > num_leaves. (num_leaves=31).
[LightGBM] [Warning] Accuracy may be bad since you didn't explicitly set num_leaves OR 2^max_depth > num_leaves. (num_leaves=31).
[LightGBM] [Warning] Accuracy may be bad since you didn't explicitly set num_leaves OR 2^max_depth > num_leaves. (num_leaves=31).
[LightGBM] [Info] Auto-choosing col-wise multi-threading, the overhead of testing was 0.000043 seconds.
You can set `force_col_wise=true` to remove the overhead.
[LightGBM] [Info] Total Bins 251
[LightGBM] [Info] Number of data points in the train set: 750, number of used features: 1
[LightGBM] [Info] Start training from score 155.058841
[LightGBM] [Warning] No further splits with positive gain, best gain: -inf
[LightGBM] [Warning] No further splits with positive gain, best gain: -inf
[LightGBM] [Warning] No further splits with positive gain, best gain: -inf
[LightGBM] [Warning] No further splits with positive gain, best gain: -inf
[LightGBM] [Warning] No further splits with positive gain, best gain: -inf
[LightGBM] [Warning] No further splits with positive gain, best gain: -inf
[LightGBM] [Warning] No further splits with positive gain, best gain: -inf
[LightGBM] [Warning] No further splits with positive gain, best gain: -inf
[LightGBM] [Warning] No further splits with positive gain, best gain: -inf
[LightGBM] [Warning] No further splits with positive gain, best gain: -inf
[LightGBM] [Warning] No further splits with positive gain, best gain: -inf
[LightGBM] [Warning] No further splits with positive gain, best gain: -inf
[LightGBM] [Warning] No further splits with positive gain, best gain: -inf
[LightGBM] [Warning] No further splits with positive gain, best gain: -inf
[LightGBM] [Warning] No further splits with positive gain, best gain: -inf
[LightGBM] [Warning] No further splits with positive gain, best gain: -inf
[LightGBM] [Warning] No further splits with positive gain, best gain: -inf
[LightGBM] [Warning] No further splits with positive gain, best gain: -inf
[LightGBM] [Warning] No further splits with positive gain, best gain: -inf
[LightGBM] [Warning] No further splits with positive gain, best gain: -inf
[LightGBM] [Warning] Accuracy may be bad since you didn't explicitly set num_leaves OR 2^max_depth > num_leaves. (num_leaves=31).
[LightGBM] [Warning] Accuracy may be bad since you didn't explicitly set num_leaves OR 2^max_depth > num_leaves. (num_leaves=31).
[LightGBM] [Warning] Accuracy may be bad since you didn't explicitly set num_leaves OR 2^max_depth > num_leaves. (num_leaves=31).
[LightGBM] [Info] Auto-choosing col-wise multi-threading, the overhead of testing was 0.000047 seconds.
You can set `force_col_wise=true` to remove the overhead.
[LightGBM] [Info] Total Bins 251
[LightGBM] [Info] Number of data points in the train set: 750, number of used features: 1
[LightGBM] [Info] Start training from score 155.058841
[LightGBM] [Warning] No further splits with positive gain, best gain: -inf
[LightGBM] [Warning] No further splits with positive gain, best gain: -inf
[LightGBM] [Warning] No further splits with positive gain, best gain: -inf
[LightGBM] [Warning] No further splits with positive gain, best gain: -inf
[LightGBM] [Warning] No further splits with positive gain, best gain: -inf
[LightGBM] [Warning] No further splits with positive gain, best gain: -inf
[LightGBM] [Warning] No further splits with positive gain, best gain: -inf
[LightGBM] [Warning] No further splits with positive gain, best gain: -inf
[LightGBM] [Warning] No further splits with positive gain, best gain: -inf
[LightGBM] [Warning] No further splits with positive gain, best gain: -inf
[LightGBM] [Warning] No further splits with positive gain, best gain: -inf
[LightGBM] [Warning] No further splits with positive gain, best gain: -inf
[LightGBM] [Warning] No further splits with positive gain, best gain: -inf
[LightGBM] [Warning] No further splits with positive gain, best gain: -inf
[LightGBM] [Warning] No further splits with positive gain, best gain: -inf
[LightGBM] [Warning] No further splits with positive gain, best gain: -inf
[LightGBM] [Warning] No further splits with positive gain, best gain: -inf
[LightGBM] [Warning] No further splits with positive gain, best gain: -inf
[LightGBM] [Warning] No further splits with positive gain, best gain: -inf
[LightGBM] [Warning] No further splits with positive gain, best gain: -inf
[LightGBM] [Warning] Accuracy may be bad since you didn't explicitly set num_leaves OR 2^max_depth > num_leaves. (num_leaves=31).
[LightGBM] [Warning] Accuracy may be bad since you didn't explicitly set num_leaves OR 2^max_depth > num_leaves. (num_leaves=31).
[LightGBM] [Warning] Accuracy may be bad since you didn't explicitly set num_leaves OR 2^max_depth > num_leaves. (num_leaves=31).
[LightGBM] [Info] Auto-choosing col-wise multi-threading, the overhead of testing was 0.000045 seconds.
You can set `force_col_wise=true` to remove the overhead.
[LightGBM] [Info] Total Bins 251
[LightGBM] [Info] Number of data points in the train set: 750, number of used features: 1
[LightGBM] [Info] Start training from score 155.058841
[LightGBM] [Warning] No further splits with positive gain, best gain: -inf
[LightGBM] [Warning] No further splits with positive gain, best gain: -inf
[LightGBM] [Warning] No further splits with positive gain, best gain: -inf
[LightGBM] [Warning] No further splits with positive gain, best gain: -inf
[LightGBM] [Warning] No further splits with positive gain, best gain: -inf
[LightGBM] [Warning] No further splits with positive gain, best gain: -inf
[LightGBM] [Warning] No further splits with positive gain, best gain: -inf
[LightGBM] [Warning] No further splits with positive gain, best gain: -inf
[LightGBM] [Warning] No further splits with positive gain, best gain: -inf
[LightGBM] [Warning] No further splits with positive gain, best gain: -inf
[LightGBM] [Warning] No further splits with positive gain, best gain: -inf
[LightGBM] [Warning] No further splits with positive gain, best gain: -inf
[LightGBM] [Warning] No further splits with positive gain, best gain: -inf
[LightGBM] [Warning] No further splits with positive gain, best gain: -inf
[LightGBM] [Warning] No further splits with positive gain, best gain: -inf
[LightGBM] [Warning] No further splits with positive gain, best gain: -inf
[LightGBM] [Warning] No further splits with positive gain, best gain: -inf
[LightGBM] [Warning] No further splits with positive gain, best gain: -inf
[LightGBM] [Warning] No further splits with positive gain, best gain: -inf
[LightGBM] [Warning] No further splits with positive gain, best gain: -inf
[LightGBM] [Warning] Accuracy may be bad since you didn't explicitly set num_leaves OR 2^max_depth > num_leaves. (num_leaves=31).
100%|██████████| 25/25 [00:02<00:00, 9.30it/s]
[LightGBM] [Warning] Accuracy may be bad since you didn't explicitly set num_leaves OR 2^max_depth > num_leaves. (num_leaves=31).
[LightGBM] [Warning] Accuracy may be bad since you didn't explicitly set num_leaves OR 2^max_depth > num_leaves. (num_leaves=31).
[LightGBM] [Info] Auto-choosing col-wise multi-threading, the overhead of testing was 0.000043 seconds.
You can set `force_col_wise=true` to remove the overhead.
[LightGBM] [Info] Total Bins 250
[LightGBM] [Info] Number of data points in the train set: 750, number of used features: 1
[LightGBM] [Info] Start training from score 155.162133
[LightGBM] [Warning] No further splits with positive gain, best gain: -inf
[LightGBM] [Warning] No further splits with positive gain, best gain: -inf
[LightGBM] [Warning] No further splits with positive gain, best gain: -inf
[LightGBM] [Warning] No further splits with positive gain, best gain: -inf
[LightGBM] [Warning] No further splits with positive gain, best gain: -inf
[LightGBM] [Warning] No further splits with positive gain, best gain: -inf
[LightGBM] [Warning] No further splits with positive gain, best gain: -inf
[LightGBM] [Warning] No further splits with positive gain, best gain: -inf
[LightGBM] [Warning] No further splits with positive gain, best gain: -inf
[LightGBM] [Warning] No further splits with positive gain, best gain: -inf
[LightGBM] [Warning] No further splits with positive gain, best gain: -inf
[LightGBM] [Warning] No further splits with positive gain, best gain: -inf
[LightGBM] [Warning] No further splits with positive gain, best gain: -inf
[LightGBM] [Warning] No further splits with positive gain, best gain: -inf
[LightGBM] [Warning] No further splits with positive gain, best gain: -inf
[LightGBM] [Warning] No further splits with positive gain, best gain: -inf
[LightGBM] [Warning] No further splits with positive gain, best gain: -inf
[LightGBM] [Warning] No further splits with positive gain, best gain: -inf
[LightGBM] [Warning] No further splits with positive gain, best gain: -inf
[LightGBM] [Warning] No further splits with positive gain, best gain: -inf
[LightGBM] [Warning] Accuracy may be bad since you didn't explicitly set num_leaves OR 2^max_depth > num_leaves. (num_leaves=31).
[LightGBM] [Warning] Accuracy may be bad since you didn't explicitly set num_leaves OR 2^max_depth > num_leaves. (num_leaves=31).
[LightGBM] [Warning] Accuracy may be bad since you didn't explicitly set num_leaves OR 2^max_depth > num_leaves. (num_leaves=31).
[LightGBM] [Info] Auto-choosing col-wise multi-threading, the overhead of testing was 0.000041 seconds.
You can set `force_col_wise=true` to remove the overhead.
[LightGBM] [Info] Total Bins 250
[LightGBM] [Info] Number of data points in the train set: 750, number of used features: 1
[LightGBM] [Info] Start training from score 155.162133
[LightGBM] [Warning] No further splits with positive gain, best gain: -inf
[LightGBM] [Warning] No further splits with positive gain, best gain: -inf
[LightGBM] [Warning] No further splits with positive gain, best gain: -inf
[LightGBM] [Warning] No further splits with positive gain, best gain: -inf
[LightGBM] [Warning] No further splits with positive gain, best gain: -inf
[LightGBM] [Warning] No further splits with positive gain, best gain: -inf
[LightGBM] [Warning] No further splits with positive gain, best gain: -inf
[LightGBM] [Warning] No further splits with positive gain, best gain: -inf
[LightGBM] [Warning] No further splits with positive gain, best gain: -inf
[LightGBM] [Warning] No further splits with positive gain, best gain: -inf
[LightGBM] [Warning] No further splits with positive gain, best gain: -inf
[LightGBM] [Warning] No further splits with positive gain, best gain: -inf
[LightGBM] [Warning] No further splits with positive gain, best gain: -inf
[LightGBM] [Warning] No further splits with positive gain, best gain: -inf
[LightGBM] [Warning] No further splits with positive gain, best gain: -inf
[LightGBM] [Warning] No further splits with positive gain, best gain: -inf
[LightGBM] [Warning] No further splits with positive gain, best gain: -inf
[LightGBM] [Warning] No further splits with positive gain, best gain: -inf
[LightGBM] [Warning] No further splits with positive gain, best gain: -inf
[LightGBM] [Warning] No further splits with positive gain, best gain: -inf
[LightGBM] [Warning] Accuracy may be bad since you didn't explicitly set num_leaves OR 2^max_depth > num_leaves. (num_leaves=31).
[LightGBM] [Warning] Accuracy may be bad since you didn't explicitly set num_leaves OR 2^max_depth > num_leaves. (num_leaves=31).
[LightGBM] [Warning] Accuracy may be bad since you didn't explicitly set num_leaves OR 2^max_depth > num_leaves. (num_leaves=31).
[LightGBM] [Info] Auto-choosing col-wise multi-threading, the overhead of testing was 0.000043 seconds.
You can set `force_col_wise=true` to remove the overhead.
[LightGBM] [Info] Total Bins 250
[LightGBM] [Info] Number of data points in the train set: 750, number of used features: 1
[LightGBM] [Info] Start training from score 155.162133
[LightGBM] [Warning] No further splits with positive gain, best gain: -inf
[LightGBM] [Warning] No further splits with positive gain, best gain: -inf
[LightGBM] [Warning] No further splits with positive gain, best gain: -inf
[LightGBM] [Warning] No further splits with positive gain, best gain: -inf
[LightGBM] [Warning] No further splits with positive gain, best gain: -inf
[LightGBM] [Warning] No further splits with positive gain, best gain: -inf
[LightGBM] [Warning] No further splits with positive gain, best gain: -inf
[LightGBM] [Warning] No further splits with positive gain, best gain: -inf
[LightGBM] [Warning] No further splits with positive gain, best gain: -inf
[LightGBM] [Warning] No further splits with positive gain, best gain: -inf
[LightGBM] [Warning] No further splits with positive gain, best gain: -inf
[LightGBM] [Warning] No further splits with positive gain, best gain: -inf
[LightGBM] [Warning] No further splits with positive gain, best gain: -inf
[LightGBM] [Warning] No further splits with positive gain, best gain: -inf
[LightGBM] [Warning] No further splits with positive gain, best gain: -inf
[LightGBM] [Warning] No further splits with positive gain, best gain: -inf
[LightGBM] [Warning] No further splits with positive gain, best gain: -inf
[LightGBM] [Warning] No further splits with positive gain, best gain: -inf
[LightGBM] [Warning] No further splits with positive gain, best gain: -inf
[LightGBM] [Warning] No further splits with positive gain, best gain: -inf
[LightGBM] [Warning] Accuracy may be bad since you didn't explicitly set num_leaves OR 2^max_depth > num_leaves. (num_leaves=31).
[LightGBM] [Warning] Accuracy may be bad since you didn't explicitly set num_leaves OR 2^max_depth > num_leaves. (num_leaves=31).
[LightGBM] [Warning] Accuracy may be bad since you didn't explicitly set num_leaves OR 2^max_depth > num_leaves. (num_leaves=31).
[LightGBM] [Info] Auto-choosing col-wise multi-threading, the overhead of testing was 0.000035 seconds.
You can set `force_col_wise=true` to remove the overhead.
[LightGBM] [Info] Total Bins 250
[LightGBM] [Info] Number of data points in the train set: 750, number of used features: 1
[LightGBM] [Info] Start training from score 155.433042
[LightGBM] [Warning] No further splits with positive gain, best gain: -inf
[LightGBM] [Warning] No further splits with positive gain, best gain: -inf
[LightGBM] [Warning] No further splits with positive gain, best gain: -inf
[LightGBM] [Warning] No further splits with positive gain, best gain: -inf
[LightGBM] [Warning] No further splits with positive gain, best gain: -inf
[LightGBM] [Warning] No further splits with positive gain, best gain: -inf
[LightGBM] [Warning] No further splits with positive gain, best gain: -inf
[LightGBM] [Warning] No further splits with positive gain, best gain: -inf
[LightGBM] [Warning] No further splits with positive gain, best gain: -inf
[LightGBM] [Warning] No further splits with positive gain, best gain: -inf
[LightGBM] [Warning] No further splits with positive gain, best gain: -inf
[LightGBM] [Warning] No further splits with positive gain, best gain: -inf
[LightGBM] [Warning] No further splits with positive gain, best gain: -inf
[LightGBM] [Warning] No further splits with positive gain, best gain: -inf
[LightGBM] [Warning] No further splits with positive gain, best gain: -inf
[LightGBM] [Warning] No further splits with positive gain, best gain: -inf
[LightGBM] [Warning] No further splits with positive gain, best gain: -inf
[LightGBM] [Warning] No further splits with positive gain, best gain: -inf
[LightGBM] [Warning] No further splits with positive gain, best gain: -inf
[LightGBM] [Warning] No further splits with positive gain, best gain: -inf
[LightGBM] [Warning] Accuracy may be bad since you didn't explicitly set num_leaves OR 2^max_depth > num_leaves. (num_leaves=31).
[LightGBM] [Warning] Accuracy may be bad since you didn't explicitly set num_leaves OR 2^max_depth > num_leaves. (num_leaves=31).
[LightGBM] [Warning] Accuracy may be bad since you didn't explicitly set num_leaves OR 2^max_depth > num_leaves. (num_leaves=31).
[LightGBM] [Info] Auto-choosing col-wise multi-threading, the overhead of testing was 0.000032 seconds.
You can set `force_col_wise=true` to remove the overhead.
[LightGBM] [Info] Total Bins 250
[LightGBM] [Info] Number of data points in the train set: 750, number of used features: 1
[LightGBM] [Info] Start training from score 155.433042
[LightGBM] [Warning] No further splits with positive gain, best gain: -inf
[LightGBM] [Warning] No further splits with positive gain, best gain: -inf
[LightGBM] [Warning] No further splits with positive gain, best gain: -inf
[LightGBM] [Warning] No further splits with positive gain, best gain: -inf
[LightGBM] [Warning] No further splits with positive gain, best gain: -inf
[LightGBM] [Warning] No further splits with positive gain, best gain: -inf
[LightGBM] [Warning] No further splits with positive gain, best gain: -inf
[LightGBM] [Warning] No further splits with positive gain, best gain: -inf
[LightGBM] [Warning] No further splits with positive gain, best gain: -inf
[LightGBM] [Warning] No further splits with positive gain, best gain: -inf
[LightGBM] [Warning] No further splits with positive gain, best gain: -inf
[LightGBM] [Warning] No further splits with positive gain, best gain: -inf
[LightGBM] [Warning] No further splits with positive gain, best gain: -inf
[LightGBM] [Warning] No further splits with positive gain, best gain: -inf
[LightGBM] [Warning] No further splits with positive gain, best gain: -inf
[LightGBM] [Warning] No further splits with positive gain, best gain: -inf
[LightGBM] [Warning] No further splits with positive gain, best gain: -inf
[LightGBM] [Warning] No further splits with positive gain, best gain: -inf
[LightGBM] [Warning] No further splits with positive gain, best gain: -inf
[LightGBM] [Warning] No further splits with positive gain, best gain: -inf
[LightGBM] [Warning] Accuracy may be bad since you didn't explicitly set num_leaves OR 2^max_depth > num_leaves. (num_leaves=31).
[LightGBM] [Warning] Accuracy may be bad since you didn't explicitly set num_leaves OR 2^max_depth > num_leaves. (num_leaves=31).
[LightGBM] [Warning] Accuracy may be bad since you didn't explicitly set num_leaves OR 2^max_depth > num_leaves. (num_leaves=31).
[LightGBM] [Info] Auto-choosing col-wise multi-threading, the overhead of testing was 0.000046 seconds.
You can set `force_col_wise=true` to remove the overhead.
[LightGBM] [Info] Total Bins 250
[LightGBM] [Info] Number of data points in the train set: 750, number of used features: 1
[LightGBM] [Info] Start training from score 155.433042
[LightGBM] [Warning] No further splits with positive gain, best gain: -inf
[LightGBM] [Warning] No further splits with positive gain, best gain: -inf
[LightGBM] [Warning] No further splits with positive gain, best gain: -inf
[LightGBM] [Warning] No further splits with positive gain, best gain: -inf
[LightGBM] [Warning] No further splits with positive gain, best gain: -inf
[LightGBM] [Warning] No further splits with positive gain, best gain: -inf
[LightGBM] [Warning] No further splits with positive gain, best gain: -inf
[LightGBM] [Warning] No further splits with positive gain, best gain: -inf
[LightGBM] [Warning] No further splits with positive gain, best gain: -inf
[LightGBM] [Warning] No further splits with positive gain, best gain: -inf
[LightGBM] [Warning] No further splits with positive gain, best gain: -inf
[LightGBM] [Warning] No further splits with positive gain, best gain: -inf
[LightGBM] [Warning] No further splits with positive gain, best gain: -inf
[LightGBM] [Warning] No further splits with positive gain, best gain: -inf
[LightGBM] [Warning] No further splits with positive gain, best gain: -inf
[LightGBM] [Warning] No further splits with positive gain, best gain: -inf
[LightGBM] [Warning] No further splits with positive gain, best gain: -inf
[LightGBM] [Warning] No further splits with positive gain, best gain: -inf
[LightGBM] [Warning] No further splits with positive gain, best gain: -inf
[LightGBM] [Warning] No further splits with positive gain, best gain: -inf
[LightGBM] [Warning] Accuracy may be bad since you didn't explicitly set num_leaves OR 2^max_depth > num_leaves. (num_leaves=31).
[LightGBM] [Warning] Accuracy may be bad since you didn't explicitly set num_leaves OR 2^max_depth > num_leaves. (num_leaves=31).
[LightGBM] [Warning] Accuracy may be bad since you didn't explicitly set num_leaves OR 2^max_depth > num_leaves. (num_leaves=31).
[LightGBM] [Info] Auto-choosing col-wise multi-threading, the overhead of testing was 0.000039 seconds.
You can set `force_col_wise=true` to remove the overhead.
[LightGBM] [Info] Total Bins 250
[LightGBM] [Info] Number of data points in the train set: 750, number of used features: 1
[LightGBM] [Info] Start training from score 155.500633
[LightGBM] [Warning] No further splits with positive gain, best gain: -inf
[LightGBM] [Warning] No further splits with positive gain, best gain: -inf
[LightGBM] [Warning] No further splits with positive gain, best gain: -inf
[LightGBM] [Warning] No further splits with positive gain, best gain: -inf
[LightGBM] [Warning] No further splits with positive gain, best gain: -inf
[LightGBM] [Warning] No further splits with positive gain, best gain: -inf
[LightGBM] [Warning] No further splits with positive gain, best gain: -inf
[LightGBM] [Warning] No further splits with positive gain, best gain: -inf
[LightGBM] [Warning] No further splits with positive gain, best gain: -inf
[LightGBM] [Warning] No further splits with positive gain, best gain: -inf
[LightGBM] [Warning] No further splits with positive gain, best gain: -inf
[LightGBM] [Warning] No further splits with positive gain, best gain: -inf
[LightGBM] [Warning] No further splits with positive gain, best gain: -inf
[LightGBM] [Warning] No further splits with positive gain, best gain: -inf
[LightGBM] [Warning] No further splits with positive gain, best gain: -inf
[LightGBM] [Warning] No further splits with positive gain, best gain: -inf
[LightGBM] [Warning] No further splits with positive gain, best gain: -inf
[LightGBM] [Warning] No further splits with positive gain, best gain: -inf
[LightGBM] [Warning] No further splits with positive gain, best gain: -inf
[LightGBM] [Warning] No further splits with positive gain, best gain: -inf
[LightGBM] [Warning] Accuracy may be bad since you didn't explicitly set num_leaves OR 2^max_depth > num_leaves. (num_leaves=31).
[LightGBM] [Warning] Accuracy may be bad since you didn't explicitly set num_leaves OR 2^max_depth > num_leaves. (num_leaves=31).
[LightGBM] [Warning] Accuracy may be bad since you didn't explicitly set num_leaves OR 2^max_depth > num_leaves. (num_leaves=31).
[LightGBM] [Info] Auto-choosing col-wise multi-threading, the overhead of testing was 0.000039 seconds.
You can set `force_col_wise=true` to remove the overhead.
[LightGBM] [Info] Total Bins 250
[LightGBM] [Info] Number of data points in the train set: 750, number of used features: 1
[LightGBM] [Info] Start training from score 155.500633
[LightGBM] [Warning] No further splits with positive gain, best gain: -inf
[LightGBM] [Warning] No further splits with positive gain, best gain: -inf
[LightGBM] [Warning] No further splits with positive gain, best gain: -inf
[LightGBM] [Warning] No further splits with positive gain, best gain: -inf
[LightGBM] [Warning] No further splits with positive gain, best gain: -inf
[LightGBM] [Warning] No further splits with positive gain, best gain: -inf
[LightGBM] [Warning] No further splits with positive gain, best gain: -inf
[LightGBM] [Warning] No further splits with positive gain, best gain: -inf
[LightGBM] [Warning] No further splits with positive gain, best gain: -inf
[LightGBM] [Warning] No further splits with positive gain, best gain: -inf
[LightGBM] [Warning] No further splits with positive gain, best gain: -inf
[LightGBM] [Warning] No further splits with positive gain, best gain: -inf
[LightGBM] [Warning] No further splits with positive gain, best gain: -inf
[LightGBM] [Warning] No further splits with positive gain, best gain: -inf
[LightGBM] [Warning] No further splits with positive gain, best gain: -inf
[LightGBM] [Warning] No further splits with positive gain, best gain: -inf
[LightGBM] [Warning] No further splits with positive gain, best gain: -inf
[LightGBM] [Warning] No further splits with positive gain, best gain: -inf
[LightGBM] [Warning] No further splits with positive gain, best gain: -inf
[LightGBM] [Warning] No further splits with positive gain, best gain: -inf
[LightGBM] [Warning] Accuracy may be bad since you didn't explicitly set num_leaves OR 2^max_depth > num_leaves. (num_leaves=31).
[LightGBM] [Warning] Accuracy may be bad since you didn't explicitly set num_leaves OR 2^max_depth > num_leaves. (num_leaves=31).
[LightGBM] [Warning] Accuracy may be bad since you didn't explicitly set num_leaves OR 2^max_depth > num_leaves. (num_leaves=31).
[LightGBM] [Info] Auto-choosing col-wise multi-threading, the overhead of testing was 0.000053 seconds.
You can set `force_col_wise=true` to remove the overhead.
[LightGBM] [Info] Total Bins 250
[LightGBM] [Info] Number of data points in the train set: 750, number of used features: 1
[LightGBM] [Info] Start training from score 155.500633
[LightGBM] [Warning] No further splits with positive gain, best gain: -inf
[LightGBM] [Warning] No further splits with positive gain, best gain: -inf
[LightGBM] [Warning] No further splits with positive gain, best gain: -inf
[LightGBM] [Warning] No further splits with positive gain, best gain: -inf
[LightGBM] [Warning] No further splits with positive gain, best gain: -inf
[LightGBM] [Warning] No further splits with positive gain, best gain: -inf
[LightGBM] [Warning] No further splits with positive gain, best gain: -inf
[LightGBM] [Warning] No further splits with positive gain, best gain: -inf
[LightGBM] [Warning] No further splits with positive gain, best gain: -inf
[LightGBM] [Warning] No further splits with positive gain, best gain: -inf
[LightGBM] [Warning] No further splits with positive gain, best gain: -inf
[LightGBM] [Warning] No further splits with positive gain, best gain: -inf
[LightGBM] [Warning] No further splits with positive gain, best gain: -inf
[LightGBM] [Warning] No further splits with positive gain, best gain: -inf
[LightGBM] [Warning] No further splits with positive gain, best gain: -inf
[LightGBM] [Warning] No further splits with positive gain, best gain: -inf
[LightGBM] [Warning] No further splits with positive gain, best gain: -inf
[LightGBM] [Warning] No further splits with positive gain, best gain: -inf
[LightGBM] [Warning] No further splits with positive gain, best gain: -inf
[LightGBM] [Warning] No further splits with positive gain, best gain: -inf
[LightGBM] [Warning] Accuracy may be bad since you didn't explicitly set num_leaves OR 2^max_depth > num_leaves. (num_leaves=31).
[58]:
[[0.8384828369380417,
0.7470535641154521,
0.8395674441554806,
0.8460697933405346],
[0.8966181473711606,
0.7767690018820292,
0.8808463799894176,
0.8937462469421166]]
[59]:
fig, ax = plt.subplots(1, 1, figsize=(10, 4))
ax.plot([_[0] for _ in scores], label="RandomForestRegressor(5)")
ax.plot([_[1] for _ in scores], label="LGB(5, lr=0.05)")
ax.plot([_[2] for _ in scores], label="LGB(5, lr=0.1)")
ax.plot([_[3] for _ in scores], label="LGB(5, lr=0.2)")
ax.set_title(
"Comparaison pour différents learning_rate\net des arbres de décisions "
"avec LightGBM"
)
ax.legend();
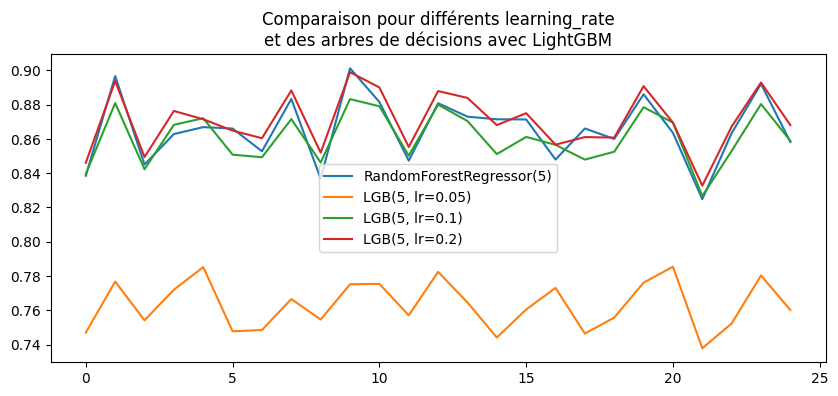
LightGBM paraît moins sensible au learning_rate que XGBoost.
CatBoost¶
CatBoost est une des plus récentes. Elle est sensée être plus efficace pour les catégories ce qui n’est pas le cas ici.
[60]:
from catboost import CatBoostRegressor
[61]:
scores = experiment(
[
RandomForestRegressor(max_depth=5, n_estimators=20),
CatBoostRegressor(
max_depth=1, n_estimators=20, learning_rate=0.05, verbose=False
),
CatBoostRegressor(
max_depth=1, n_estimators=20, learning_rate=0.1, verbose=False
),
CatBoostRegressor(
max_depth=1, n_estimators=20, learning_rate=0.2, verbose=False
),
]
)
scores[:2]
100%|██████████| 25/25 [00:11<00:00, 2.26it/s]
[61]:
[[0.8878458732034202,
0.5106308809971148,
0.6633876012158881,
0.7962100349534728],
[0.8702333178716275,
0.47507766677086194,
0.632648027686756,
0.7779024827838191]]
[62]:
fig, ax = plt.subplots(1, 1, figsize=(10, 4))
ax.plot([_[0] for _ in scores], label="RandomForestRegressor(5)")
ax.plot([_[1] for _ in scores], label="CAT(1, lr=0.05)")
ax.plot([_[2] for _ in scores], label="CAT(1, lr=0.1)")
ax.plot([_[3] for _ in scores], label="CAT(1, lr=0.2)")
ax.set_title(
"Comparaison pour différents learning_rate\net des fonctions en escalier "
"avec CatBoost"
)
ax.legend();
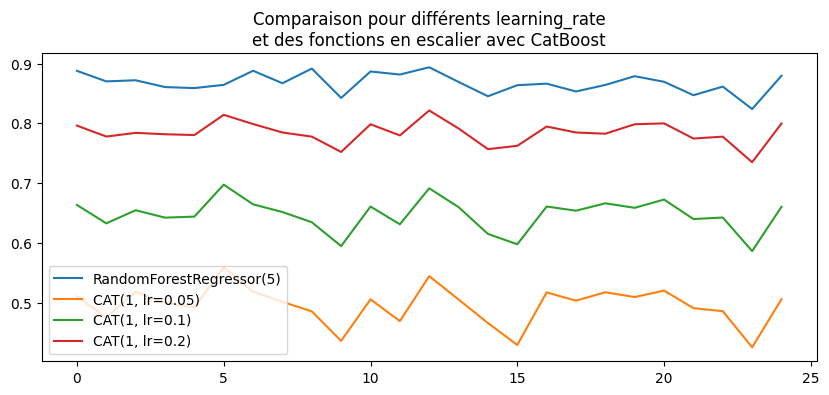
[63]:
scores = experiment(
[
RandomForestRegressor(max_depth=5, n_estimators=20),
CatBoostRegressor(
max_depth=5, n_estimators=20, learning_rate=0.05, verbose=False
),
CatBoostRegressor(
max_depth=5, n_estimators=20, learning_rate=0.1, verbose=False
),
CatBoostRegressor(
max_depth=5, n_estimators=20, learning_rate=0.2, verbose=False
),
]
)
scores[:2]
100%|██████████| 25/25 [00:12<00:00, 1.97it/s]
[63]:
[[0.8605624010619503,
0.7022983070352993,
0.8366211999791443,
0.8636015275401271],
[0.8456129223719402,
0.7027514870740816,
0.8333140676555679,
0.8596728951071267]]
[64]:
fig, ax = plt.subplots(1, 1, figsize=(10, 4))
ax.plot([_[0] for _ in scores], label="RandomForestRegressor(5)")
ax.plot([_[1] for _ in scores], label="CAT(5, lr=0.05)")
ax.plot([_[2] for _ in scores], label="CAT(5, lr=0.1)")
ax.plot([_[3] for _ in scores], label="CAT(5, lr=0.2)")
ax.set_title(
"Comparaison pour différents learning_rate\net des fonctions en escalier "
"avec CatBoost"
)
ax.legend();
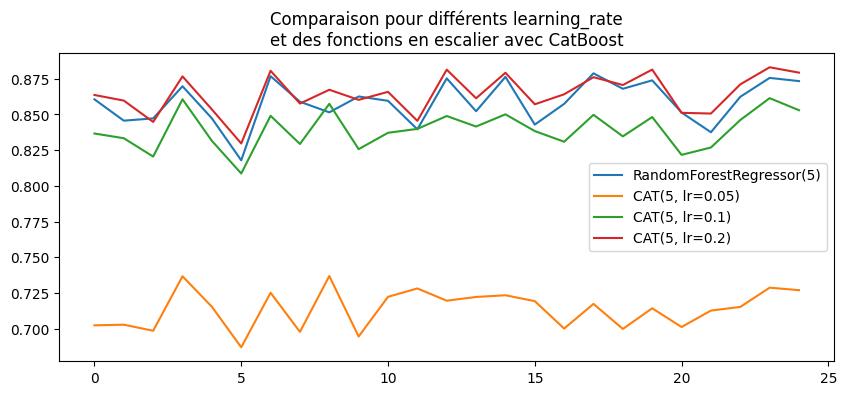