NeuralTreeNet et coût#
La classe NeuralTreeNet convertit un arbre de décision en réseau de neurones. Si la conversion n’est pas exacte mais elle permet d’obtenir un modèle différentiable et apprenable avec un algorithme d’optimisation à base de gradient. Ce notebook compare le temps d’éxécution entre un arbre et le réseau de neurones.
[1]:
%matplotlib inline
Jeux de données#
On construit un jeu de données aléatoire.
[2]:
import numpy
X = numpy.random.randn(10000, 10)
y = X.sum(axis=1) / X.shape[1]
X = X.astype(numpy.float64)
y = y.astype(numpy.float64)
[3]:
middle = X.shape[0] // 2
X_train, X_test = X[:middle], X[middle:]
y_train, y_test = y[:middle], y[middle:]
Caler un arbre de décision#
[4]:
from sklearn.tree import DecisionTreeRegressor
tree = DecisionTreeRegressor(max_depth=7)
tree.fit(X_train, y_train)
tree.score(X_train, y_train), tree.score(X_test, y_test)
[4]:
(0.6225001966466359, 0.37938295559354807)
[5]:
from sklearn.metrics import r2_score
r2_score(y_test, tree.predict(X_test))
[5]:
0.37938295559354807
Covnersion de l’arbre en réseau de neurones
[6]:
from pandas import DataFrame
from mlstatpy.ml.neural_tree import NeuralTreeNet, NeuralTreeNetRegressor
xe = X_test.astype(numpy.float32)
expected = tree.predict(xe)
nn = NeuralTreeNetRegressor(NeuralTreeNet.create_from_tree(tree, arch="compact"))
got = nn.predict(xe)
me = numpy.abs(got - expected).mean()
mx = numpy.abs(got - expected).max()
DataFrame([{"average absolute error": me, "max absolute error": mx}]).T
[6]:
0 | |
---|---|
average absolute error | 0.208776 |
max absolute error | 1.427806 |
La conversion est loin d’être parfaite. La raison vient du fait que les fonctions de seuil sont approchées par des fonctions sigmoïdes. Il suffit d’une erreur minime pour que la décision prenne un chemin différent dans l’arbre et soit complètement différente.
Conversion au format ONNX#
[7]:
from skl2onnx import to_onnx
onx_tree = to_onnx(tree, X[:1].astype(numpy.float32))
onx_nn = to_onnx(nn, X[:1].astype(numpy.float32))
Le réseau de neurones peut être représenté comme suit.
[8]:
from onnx_array_api.plotting.text_plot import onnx_simple_text_plot
print(onnx_simple_text_plot(onx_nn))
opset: domain='' version=21
input: name='X' type=dtype('float32') shape=['', 10]
init: name='Ma_MatMulcst' type=dtype('float32') shape=(10, 127)
init: name='Ad_Addcst' type=dtype('float32') shape=(127,)
init: name='Mu_Mulcst' type=dtype('float32') shape=(1,) -- array([4.], dtype=float32)
init: name='Ma_MatMulcst1' type=dtype('float32') shape=(127, 128)
init: name='Ad_Addcst1' type=dtype('float32') shape=(128,)
init: name='Ma_MatMulcst2' type=dtype('float32') shape=(128, 1)
init: name='Ad_Addcst2' type=dtype('float32') shape=(1,) -- array([0.], dtype=float32)
MatMul(X, Ma_MatMulcst) -> Ma_Y02
Add(Ma_Y02, Ad_Addcst) -> Ad_C02
Mul(Ad_C02, Mu_Mulcst) -> Mu_C01
Sigmoid(Mu_C01) -> Si_Y01
MatMul(Si_Y01, Ma_MatMulcst1) -> Ma_Y01
Add(Ma_Y01, Ad_Addcst1) -> Ad_C01
Mul(Ad_C01, Mu_Mulcst) -> Mu_C0
Sigmoid(Mu_C0) -> Si_Y0
MatMul(Si_Y0, Ma_MatMulcst2) -> Ma_Y0
Add(Ma_Y0, Ad_Addcst2) -> Ad_C0
Identity(Ad_C0) -> variable
output: name='variable' type=dtype('float32') shape=['', 1]
Temps de calcul des prédictions#
[9]:
from onnxruntime import InferenceSession
oinf_tree = InferenceSession(onx_tree.SerializeToString())
oinf_nn = InferenceSession(onx_nn.SerializeToString())
%timeit tree.predict(xe)
584 μs ± 16.3 μs per loop (mean ± std. dev. of 7 runs, 1,000 loops each)
[10]:
%timeit oinf_tree.run(None, {'X': xe})
48.4 μs ± 1.16 μs per loop (mean ± std. dev. of 7 runs, 10,000 loops each)
[11]:
%timeit oinf_nn.run(None, {'X': xe})
1.28 ms ± 97.7 μs per loop (mean ± std. dev. of 7 runs, 1,000 loops each)
Le temps de calcul est nettement plus long pour le réseau de neurones. Si l’arbre de décision a une profondeur de d, l’arbre de décision va faire exactement d comparaisons. Le réseau de neurones quant à lui évalue tous les seuils pour chaque prédiction, soit \(2^d\). Vérifions cela en faisant variable la profondeur.
Temps de calcul en fonction de la profondeur#
[12]:
from tqdm import tqdm
from onnx_array_api.ext_test_case import measure_time
data = []
for d in tqdm(range(2, 10)):
tree = DecisionTreeRegressor(max_depth=d)
tree.fit(X_train, y_train)
obs = measure_time(lambda tree=tree: tree.predict(xe), number=20, repeat=20)
obs.update(dict(d=d, exp="skl"))
data.append(obs)
nn = NeuralTreeNetRegressor(NeuralTreeNet.create_from_tree(tree, arch="compact"))
onx_tree = to_onnx(tree, X[:1].astype(numpy.float32))
onx_nn = to_onnx(nn, X[:1].astype(numpy.float32))
oinf_tree = InferenceSession(
onx_tree.SerializePartialToString(), providers=["CPUExecutionProvider"]
)
oinf_nn = InferenceSession(
onx_nn.SerializePartialToString(), providers=["CPUExecutionProvider"]
)
obs = measure_time(
lambda oinf_tree=oinf_tree: oinf_tree.run(None, {"X": xe}), number=10, repeat=10
)
obs.update(dict(d=d, exp="onx_tree"))
data.append(obs)
obs = measure_time(
lambda oinf_nn=oinf_nn: oinf_nn.run(None, {"X": xe}), number=10, repeat=10
)
obs.update(dict(d=d, exp="onx_nn"))
data.append(obs)
df = DataFrame(data)
df
100%|██████████| 8/8 [00:04<00:00, 1.63it/s]
[12]:
average | deviation | min_exec | max_exec | repeat | number | ttime | context_size | d | exp | |
---|---|---|---|---|---|---|---|---|---|---|
0 | 0.000207 | 0.000045 | 0.000153 | 0.000317 | 20 | 20 | 0.004147 | 64 | 2 | skl |
1 | 0.000151 | 0.000246 | 0.000031 | 0.000825 | 10 | 10 | 0.001515 | 64 | 2 | onx_tree |
2 | 0.000178 | 0.000093 | 0.000119 | 0.000371 | 10 | 10 | 0.001781 | 64 | 2 | onx_nn |
3 | 0.000249 | 0.000036 | 0.000220 | 0.000360 | 20 | 20 | 0.004980 | 64 | 3 | skl |
4 | 0.000312 | 0.000156 | 0.000113 | 0.000661 | 10 | 10 | 0.003117 | 64 | 3 | onx_tree |
5 | 0.000352 | 0.000204 | 0.000182 | 0.000831 | 10 | 10 | 0.003523 | 64 | 3 | onx_nn |
6 | 0.000339 | 0.000073 | 0.000257 | 0.000487 | 20 | 20 | 0.006775 | 64 | 4 | skl |
7 | 0.000337 | 0.000423 | 0.000059 | 0.001537 | 10 | 10 | 0.003368 | 64 | 4 | onx_tree |
8 | 0.000619 | 0.000354 | 0.000221 | 0.001320 | 10 | 10 | 0.006194 | 64 | 4 | onx_nn |
9 | 0.000359 | 0.000038 | 0.000309 | 0.000453 | 20 | 20 | 0.007171 | 64 | 5 | skl |
10 | 0.000473 | 0.000565 | 0.000064 | 0.001923 | 10 | 10 | 0.004729 | 64 | 5 | onx_tree |
11 | 0.001197 | 0.000944 | 0.000309 | 0.003529 | 10 | 10 | 0.011973 | 64 | 5 | onx_nn |
12 | 0.000386 | 0.000022 | 0.000359 | 0.000439 | 20 | 20 | 0.007715 | 64 | 6 | skl |
13 | 0.000793 | 0.000770 | 0.000097 | 0.002445 | 10 | 10 | 0.007926 | 64 | 6 | onx_tree |
14 | 0.001521 | 0.000919 | 0.000652 | 0.003820 | 10 | 10 | 0.015207 | 64 | 6 | onx_nn |
15 | 0.000429 | 0.000024 | 0.000404 | 0.000494 | 20 | 20 | 0.008579 | 64 | 7 | skl |
16 | 0.000658 | 0.000662 | 0.000207 | 0.002484 | 10 | 10 | 0.006575 | 64 | 7 | onx_tree |
17 | 0.002925 | 0.002770 | 0.001489 | 0.011048 | 10 | 10 | 0.029251 | 64 | 7 | onx_nn |
18 | 0.000508 | 0.000059 | 0.000452 | 0.000733 | 20 | 20 | 0.010157 | 64 | 8 | skl |
19 | 0.001235 | 0.001208 | 0.000121 | 0.003842 | 10 | 10 | 0.012347 | 64 | 8 | onx_tree |
20 | 0.004627 | 0.004239 | 0.002962 | 0.017300 | 10 | 10 | 0.046271 | 64 | 8 | onx_nn |
21 | 0.000558 | 0.000045 | 0.000498 | 0.000700 | 20 | 20 | 0.011152 | 64 | 9 | skl |
22 | 0.000745 | 0.000540 | 0.000138 | 0.002166 | 10 | 10 | 0.007449 | 64 | 9 | onx_tree |
23 | 0.011127 | 0.004856 | 0.009014 | 0.025667 | 10 | 10 | 0.111265 | 64 | 9 | onx_nn |
[14]:
piv = df.pivot(index="d", columns="exp", values="average")
piv.plot(logy=True, title="Temps de calcul en fonction de la profondeur");
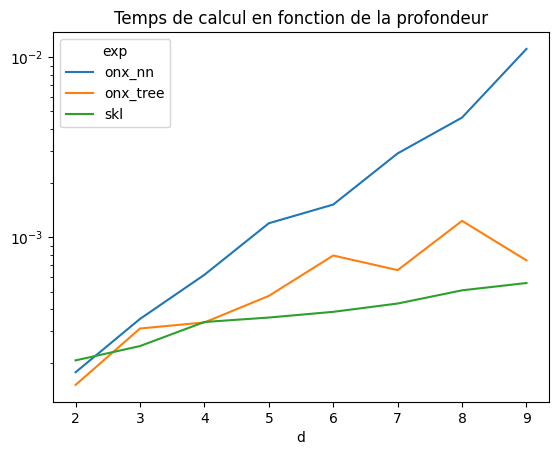
L’hypothèse est vérifiée.
[17]: