ROC¶
A few graphs about ROC on the iris datasets.
[1]:
%matplotlib inline
import matplotlib.pyplot as plt
Iris datasets¶
[3]:
from sklearn import datasets
iris = datasets.load_iris()
X = iris.data[:, :2]
y = iris.target
[4]:
from sklearn.model_selection import train_test_split
X_train, X_test, y_train, y_test = train_test_split(X, y, test_size=0.33)
[5]:
from sklearn.linear_model import LogisticRegression
clf = LogisticRegression()
clf.fit(X_train, y_train)
[5]:
LogisticRegression(C=1.0, class_weight=None, dual=False, fit_intercept=True,
intercept_scaling=1, max_iter=100, multi_class='ovr', n_jobs=1,
penalty='l2', random_state=None, solver='liblinear', tol=0.0001,
verbose=0, warm_start=False)
[6]:
import numpy
ypred = clf.predict(X_test)
yprob = clf.predict_proba(X_test)
score = numpy.array(list(yprob[i, ypred[i]] for i in range(len(ypred))))
[7]:
data = numpy.zeros((len(ypred), 2))
data[:, 0] = score.ravel()
data[ypred == y_test, 1] = 1
data[:5]
[7]:
array([[ 0.70495209, 1. ],
[ 0.56148737, 0. ],
[ 0.56148737, 1. ],
[ 0.77416227, 1. ],
[ 0.58631799, 0. ]])
ROC with scikit-learn¶
We use the following example Receiver Operating Characteristic (ROC).
[8]:
from sklearn.metrics import roc_curve
fpr, tpr, th = roc_curve(y_test == ypred, score)
[9]:
plt.plot(fpr, tpr, label="ROC curve")
plt.plot([0, 1], [0, 1], linestyle="--")
plt.xlim([0.0, 1.0])
plt.ylim([0.0, 1.0])
plt.xlabel("False Positive Rate")
plt.ylabel("True Positive Rate")
plt.legend(loc="lower right")
[9]:
<matplotlib.legend.Legend at 0x268373a2128>
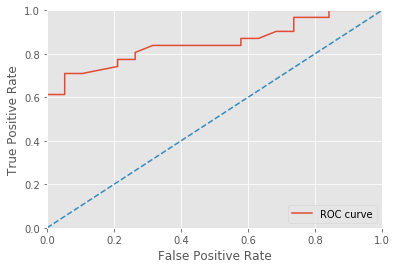
[10]:
import pandas
df = pandas.DataFrame(dict(fpr=fpr, tpr=tpr, threshold=th))
df
[10]:
fpr | threshold | tpr | |
---|---|---|---|
0 | 0.000000 | 0.910712 | 0.032258 |
1 | 0.000000 | 0.869794 | 0.096774 |
2 | 0.000000 | 0.863174 | 0.161290 |
3 | 0.000000 | 0.805864 | 0.258065 |
4 | 0.000000 | 0.790909 | 0.387097 |
5 | 0.000000 | 0.650510 | 0.612903 |
6 | 0.052632 | 0.634499 | 0.612903 |
7 | 0.052632 | 0.620319 | 0.709677 |
8 | 0.105263 | 0.615015 | 0.709677 |
9 | 0.210526 | 0.607975 | 0.741935 |
10 | 0.210526 | 0.604496 | 0.774194 |
11 | 0.263158 | 0.586318 | 0.774194 |
12 | 0.263158 | 0.584172 | 0.806452 |
13 | 0.315789 | 0.561487 | 0.838710 |
14 | 0.421053 | 0.556499 | 0.838710 |
15 | 0.578947 | 0.525449 | 0.838710 |
16 | 0.578947 | 0.522579 | 0.870968 |
17 | 0.631579 | 0.522551 | 0.870968 |
18 | 0.684211 | 0.520835 | 0.903226 |
19 | 0.736842 | 0.516687 | 0.903226 |
20 | 0.736842 | 0.453826 | 0.967742 |
21 | 0.842105 | 0.417941 | 0.967742 |
22 | 0.842105 | 0.412703 | 1.000000 |
23 | 1.000000 | 0.375573 | 1.000000 |
ROC - TPR / FPR¶
We do the same with the class this module provides ROC.
TPR = True Positive Rate
FPR = False Positive Rate
You can see as TPR the distribution function of a score for a positive example and the FPR the same for a negative example.
[11]:
from mlstatpy.ml.roc import ROC
[12]:
roc = ROC(df=data)
[13]:
roc
[13]:
Overall precision: 0.63 - AUC=0.850594
--------------
score label weight
0 0.375573 0.0 1.0
1 0.385480 0.0 1.0
2 0.412314 0.0 1.0
3 0.412703 1.0 1.0
4 0.417941 0.0 1.0
--------------
score label weight
45 0.863174 1.0 1.0
46 0.863174 1.0 1.0
47 0.869794 1.0 1.0
48 0.903335 1.0 1.0
49 0.910712 1.0 1.0
--------------
False Positive Rate True Positive Rate threshold
0 0.000000 0.032258 0.910712
1 0.000000 0.193548 0.828617
2 0.000000 0.354839 0.790909
3 0.000000 0.516129 0.737000
4 0.052632 0.645161 0.627589
5 0.157895 0.741935 0.607975
6 0.263158 0.838710 0.561487
7 0.526316 0.838710 0.542211
8 0.684211 0.903226 0.520835
9 0.842105 0.967742 0.417941
10 1.000000 1.000000 0.375573
--------------
error recall threshold
0 0.000000 0.02 0.910712
1 0.000000 0.12 0.828617
2 0.000000 0.22 0.790909
3 0.000000 0.32 0.737000
4 0.047619 0.42 0.627589
5 0.115385 0.52 0.607975
6 0.161290 0.62 0.561487
7 0.277778 0.72 0.542211
8 0.317073 0.82 0.520835
9 0.347826 0.92 0.417941
10 0.380000 1.00 0.375573
[14]:
roc.auc()
[14]:
0.85059422750424452
[15]:
roc.plot(nb=10)
[15]:
<matplotlib.axes._subplots.AxesSubplot at 0x2683ff2b668>
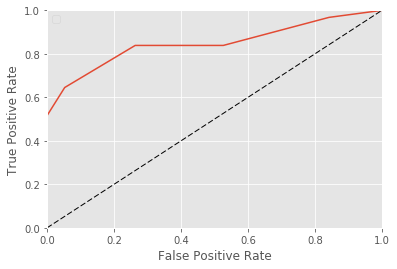
This function draws the curve with only 10 points but we can ask for more.
[16]:
roc.plot(nb=100)
[16]:
<matplotlib.axes._subplots.AxesSubplot at 0x2683feba240>
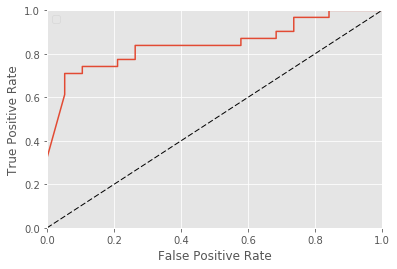
We can also ask to draw bootstropped curves to get a sense of the confidence.
[17]:
roc.plot(nb=10, bootstrap=10)
[17]:
<matplotlib.axes._subplots.AxesSubplot at 0x26840008748>
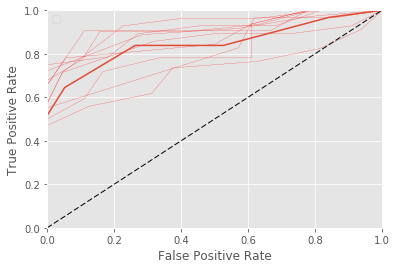
ROC - score distribution¶
This another representation for the metrics FPR and TPR. is the probability that a score for a positive example to be less than
.
is the probability that a score for a negative example to be higher than
. We assume in this case that the higher the better for the score.
[18]:
roc.plot(curve=ROC.CurveType.PROBSCORE, thresholds=True)
[18]:
<matplotlib.axes._subplots.AxesSubplot at 0x268410618d0>
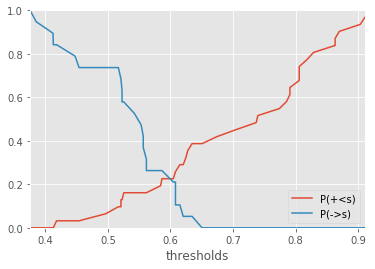
When curves intersects at score , error rates for positive and negative examples are equal. If we show the confusion matrix for this particular score
, it gives:
[19]:
conf = roc.confusion()
conf["P(+<s)"] = 1 - conf["True Positive"] / conf.loc[len(conf) - 1, "True Positive"]
conf["P(->s)"] = 1 - conf["True Negative"] / conf.loc[0, "True Negative"]
conf
[19]:
True Positive | False Positive | False Negative | True Negative | threshold | P(+<s) | P(->s) | |
---|---|---|---|---|---|---|---|
0 | 0.0 | 0.0 | 31.0 | 19.0 | 0.910712 | 1.000000 | 0.000000 |
1 | 1.0 | 0.0 | 30.0 | 19.0 | 0.910712 | 0.967742 | 0.000000 |
2 | 6.0 | 0.0 | 25.0 | 19.0 | 0.828617 | 0.806452 | 0.000000 |
3 | 11.0 | 0.0 | 20.0 | 19.0 | 0.790909 | 0.645161 | 0.000000 |
4 | 16.0 | 0.0 | 15.0 | 19.0 | 0.737000 | 0.483871 | 0.000000 |
5 | 20.0 | 1.0 | 11.0 | 18.0 | 0.627589 | 0.354839 | 0.052632 |
6 | 23.0 | 3.0 | 8.0 | 16.0 | 0.607975 | 0.258065 | 0.157895 |
7 | 26.0 | 5.0 | 5.0 | 14.0 | 0.561487 | 0.161290 | 0.263158 |
8 | 26.0 | 10.0 | 5.0 | 9.0 | 0.542211 | 0.161290 | 0.526316 |
9 | 28.0 | 13.0 | 3.0 | 6.0 | 0.520835 | 0.096774 | 0.684211 |
10 | 30.0 | 16.0 | 1.0 | 3.0 | 0.417941 | 0.032258 | 0.842105 |
11 | 31.0 | 19.0 | 0.0 | 0.0 | 0.375573 | 0.000000 | 1.000000 |
ROC - recall / precision¶
In this representation, we show the score.
[20]:
import matplotlib.pyplot as plt
fig, axes = plt.subplots(ncols=2, nrows=1, figsize=(14, 4))
roc.plot(curve=ROC.CurveType.RECPREC, thresholds=True, ax=axes[0])
roc.plot(curve=ROC.CurveType.RECPREC, ax=axes[1])
[20]:
<matplotlib.axes._subplots.AxesSubplot at 0x2684151d3c8>
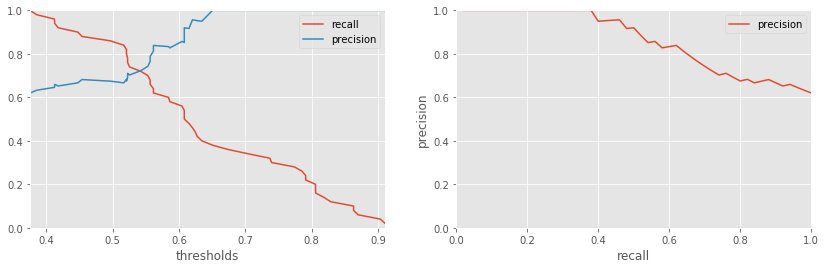
[21]: