Note
Go to the end to download the full example code.
Linear Regression and export to ONNX¶
scikit-learn and torch to train a linear regression.
data¶
import numpy as np
from sklearn.datasets import make_regression
from sklearn.linear_model import LinearRegression, SGDRegressor
from sklearn.metrics import mean_squared_error, r2_score
from sklearn.model_selection import train_test_split
import torch
from onnxruntime import InferenceSession
from experimental_experiment.helpers import pretty_onnx
from onnx_array_api.plotting.graphviz_helper import plot_dot
X, y = make_regression(1000, n_features=5, noise=10.0, n_informative=2)
print(X.shape, y.shape)
X_train, X_test, y_train, y_test = train_test_split(X, y)
(1000, 5) (1000,)
scikit-learn: the simple regression¶
clr = LinearRegression()
clr.fit(X_train, y_train)
print(f"coefficients: {clr.coef_}, {clr.intercept_}")
coefficients: [54.71668674 -0.69106611 0.38543929 -0.41235158 51.81035561], 0.26255562385228726
Evaluation¶
LinearRegression: l2=91.84279545877556, r2=0.9843716282304464
scikit-learn: SGD algorithm¶
SGD = Stochastic Gradient Descent
clr = SGDRegressor(max_iter=5, verbose=1)
clr.fit(X_train, y_train)
print(f"coefficients: {clr.coef_}, {clr.intercept_}")
-- Epoch 1
Norm: 63.49, NNZs: 5, Bias: 0.491913, T: 750, Avg. loss: 613.818693
Total training time: 0.00 seconds.
-- Epoch 2
Norm: 71.87, NNZs: 5, Bias: 0.385489, T: 1500, Avg. loss: 75.098090
Total training time: 0.00 seconds.
-- Epoch 3
Norm: 74.06, NNZs: 5, Bias: 0.432932, T: 2250, Avg. loss: 52.338135
Total training time: 0.00 seconds.
-- Epoch 4
Norm: 74.93, NNZs: 5, Bias: 0.238982, T: 3000, Avg. loss: 50.086589
Total training time: 0.00 seconds.
-- Epoch 5
Norm: 75.28, NNZs: 5, Bias: 0.297224, T: 3750, Avg. loss: 49.691118
Total training time: 0.00 seconds.
/home/xadupre/vv/this312/lib/python3.12/site-packages/sklearn/linear_model/_stochastic_gradient.py:1608: ConvergenceWarning: Maximum number of iteration reached before convergence. Consider increasing max_iter to improve the fit.
warnings.warn(
coefficients: [54.6073198 -0.68918523 0.3951037 -0.17981909 51.80928025], [0.29722431]
Evaluation
SGDRegressor: sl2=91.6582928419564, sr2=0.9844030240026864
Linrar Regression with pytorch¶
class TorchLinearRegression(torch.nn.Module):
def __init__(self, n_dims: int, n_targets: int):
super().__init__()
self.linear = torch.nn.Linear(n_dims, n_targets)
def forward(self, x):
return self.linear(x)
def train_loop(dataloader, model, loss_fn, optimizer):
total_loss = 0.0
# Set the model to training mode - important for batch normalization and dropout layers
# Unnecessary in this situation but added for best practices
model.train()
for X, y in dataloader:
# Compute prediction and loss
pred = model(X)
loss = loss_fn(pred.ravel(), y)
# Backpropagation
loss.backward()
optimizer.step()
optimizer.zero_grad()
# training loss
total_loss += loss
return total_loss
model = TorchLinearRegression(X_train.shape[1], 1)
optimizer = torch.optim.SGD(model.parameters(), lr=1e-3)
loss_fn = torch.nn.MSELoss()
device = "cpu"
model = model.to(device)
dataset = torch.utils.data.TensorDataset(
torch.Tensor(X_train).to(device), torch.Tensor(y_train).to(device)
)
dataloader = torch.utils.data.DataLoader(dataset, batch_size=1)
for i in range(5):
loss = train_loop(dataloader, model, loss_fn, optimizer)
print(f"iteration {i}, loss={loss}")
iteration 0, loss=1432482.875
iteration 1, loss=147046.625
iteration 2, loss=78746.578125
iteration 3, loss=74954.1484375
iteration 4, loss=74755.9765625
Let’s check the error
TorchLinearRegression: tl2=92.71648654069564, tr2=0.9842229571346705
And the coefficients.
print("coefficients:")
for p in model.parameters():
print(p)
coefficients:
Parameter containing:
tensor([[55.1917, -0.8006, 0.5471, -0.3315, 51.3658]], requires_grad=True)
Parameter containing:
tensor([0.1978], requires_grad=True)
Conversion to ONNX¶
Let’s convert it to ONNX.
ep = torch.onnx.export(model, (torch.Tensor(X_test[:2]),), dynamo=True)
onx = ep.model_proto
/home/xadupre/github/onnxscript/onnxscript/converter.py:823: FutureWarning: 'onnxscript.values.Op.param_schemas' is deprecated in version 0.1 and will be removed in the future. Please use '.op_signature' instead.
param_schemas = callee.param_schemas()
/home/xadupre/github/onnxscript/onnxscript/converter.py:823: FutureWarning: 'onnxscript.values.OnnxFunction.param_schemas' is deprecated in version 0.1 and will be removed in the future. Please use '.op_signature' instead.
param_schemas = callee.param_schemas()
[torch.onnx] Obtain model graph for `TorchLinearRegression([...]` with `torch.export.export(..., strict=False)`...
[torch.onnx] Obtain model graph for `TorchLinearRegression([...]` with `torch.export.export(..., strict=False)`... ✅
[torch.onnx] Run decomposition...
[torch.onnx] Run decomposition... ✅
[torch.onnx] Translate the graph into ONNX...
[torch.onnx] Translate the graph into ONNX... ✅
Let’s check it is work.
sess = InferenceSession(onx.SerializeToString(), providers=["CPUExecutionProvider"])
res = sess.run(None, {"x": X_test.astype(np.float32)[:2]})
print(res)
[array([[-44.3172 ],
[121.82564]], dtype=float32)]
And the model.
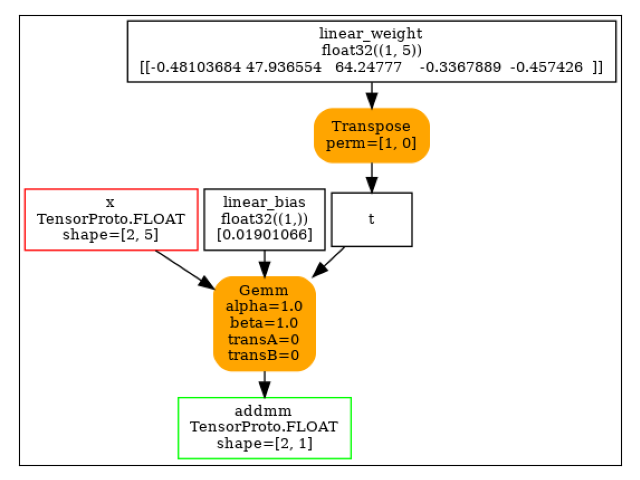
Optimization¶
By default, the exported model is not optimized and leaves many local functions. They can be inlined and the model optimized with method optimize.
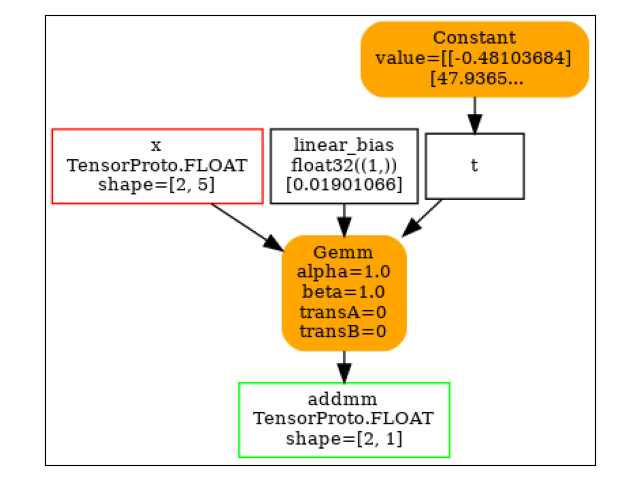
With dynamic shapes¶
The dynamic shapes are used by torch.export.export()
and must
follow the convention described there.
ep = torch.onnx.export(
model,
(torch.Tensor(X_test[:2]),),
dynamic_shapes={"x": {0: torch.export.Dim("batch")}},
dynamo=True,
)
ep.optimize()
onx = ep.model_proto
print(pretty_onnx(onx))
[torch.onnx] Obtain model graph for `TorchLinearRegression([...]` with `torch.export.export(..., strict=False)`...
[torch.onnx] Obtain model graph for `TorchLinearRegression([...]` with `torch.export.export(..., strict=False)`... ✅
[torch.onnx] Run decomposition...
[torch.onnx] Run decomposition... ✅
[torch.onnx] Translate the graph into ONNX...
[torch.onnx] Translate the graph into ONNX... ✅
opset: domain='pkg.onnxscript.torch_lib.common' version=1
opset: domain='' version=18
input: name='x' type=dtype('float32') shape=['s0', 5]
init: name='linear.weight' type=float32 shape=(1, 5)
init: name='linear.bias' type=float32 shape=(1,) -- array([0.19783159], dtype=float32)
Gemm(x, linear.weight, linear.bias, beta=1.00, transB=1, alpha=1.00, transA=0) -> linear
output: name='linear' type=dtype('float32') shape=['s0', 1]
For simplicity, it is possible to use torch.export.Dim.DYNAMIC
or torch.export.Dim.AUTO
.
ep = torch.onnx.export(
model,
(torch.Tensor(X_test[:2]),),
dynamic_shapes={"x": {0: torch.export.Dim.AUTO}},
dynamo=True,
)
ep.optimize()
onx = ep.model_proto
print(pretty_onnx(onx))
[torch.onnx] Obtain model graph for `TorchLinearRegression([...]` with `torch.export.export(..., strict=False)`...
[torch.onnx] Obtain model graph for `TorchLinearRegression([...]` with `torch.export.export(..., strict=False)`... ✅
[torch.onnx] Run decomposition...
[torch.onnx] Run decomposition... ✅
[torch.onnx] Translate the graph into ONNX...
[torch.onnx] Translate the graph into ONNX... ✅
opset: domain='pkg.onnxscript.torch_lib.common' version=1
opset: domain='' version=18
input: name='x' type=dtype('float32') shape=['s0', 5]
init: name='linear.weight' type=float32 shape=(1, 5)
init: name='linear.bias' type=float32 shape=(1,) -- array([0.19783159], dtype=float32)
Gemm(x, linear.weight, linear.bias, beta=1.00, transB=1, alpha=1.00, transA=0) -> linear
output: name='linear' type=dtype('float32') shape=['s0', 1]
Total running time of the script: (0 minutes 8.854 seconds)
Related examples