Note
Go to the end to download the full example code.
torch.onnx.export and a custom operator inplace¶
This example shows how to convert a custom operator as defined in the tutorial Python Custom Operators.
Inplace modification are not supported by onnx.
A model with a custom ops¶
import numpy as np
from onnx.printer import to_text
import onnxscript
import torch
We define a model with a custom operator.
@torch.library.custom_op("mylib::numpy_sin", mutates_args={"output"}, device_types="cpu")
def numpy_sin(x: torch.Tensor, output: torch.Tensor) -> None:
assert x.device == output.device
assert x.device.type == "cpu"
x_np = x.numpy()
output_np = output.numpy()
np.sin(x_np, out=output_np)
class ModuleWithACustomOperator(torch.nn.Module):
def forward(self, x):
out = torch.zeros(x.shape)
numpy_sin(x, out)
return out
model = ModuleWithACustomOperator()
Let’s check it runs.
x = torch.randn(1, 3)
model(x)
tensor([[ 0.9744, -0.9173, 0.6262]])
As expected, it does not export.
try:
torch.export.export(model, (x,))
raise AssertionError("This export should failed unless pytorch now supports this model.")
except Exception as e:
print(e)
This export should failed unless pytorch now supports this model.
The exporter fails with the same eror as it expects torch.export.export to work.
try:
torch.onnx.export(model, (x,), dynamo=True)
except Exception as e:
print(e)
~/github/onnxscript/onnxscript/converter.py:816: FutureWarning: 'onnxscript.values.Op.param_schemas' is deprecated in version 0.1 and will be removed in the future. Please use '.op_signature' instead.
param_schemas = callee.param_schemas()
~/github/onnxscript/onnxscript/converter.py:816: FutureWarning: 'onnxscript.values.OnnxFunction.param_schemas' is deprecated in version 0.1 and will be removed in the future. Please use '.op_signature' instead.
param_schemas = callee.param_schemas()
[torch.onnx] Obtain model graph for `ModuleWithACustomOperator()` with `torch.export.export(..., strict=False)`...
[torch.onnx] Obtain model graph for `ModuleWithACustomOperator()` with `torch.export.export(..., strict=False)`... ✅
[torch.onnx] Run decomposition...
[torch.onnx] Run decomposition... ✅
[torch.onnx] Translate the graph into ONNX...
[torch.onnx] Translate the graph into ONNX... ❌
Failed to convert the exported program to an ONNX model. This is step 3/3 of exporting the model to ONNX. Next steps:
- If there is a missing ONNX function, implement it and register it to the registry.
- If there is an internal error during ONNX conversion, debug the error and summit a PR to PyTorch.
- Create an error report with `torch.onnx.export(..., report=True)`, and save the ExportedProgram as a pt2 file. Create an issue in the PyTorch GitHub repository against the *onnx* component. Attach the error report and the pt2 model.
## Exception summary
<class 'torch.onnx._internal.exporter._errors.DispatchError'>: No ONNX function found for <torch._higher_order_ops.auto_functionalize.AutoFunctionalized object at 0x7f8bc0cb8530>. Failure message: No decompositions registered for the real-valued input
⬆️
<class 'torch.onnx._internal.exporter._errors.ConversionError'>: Error when translating node %auto_functionalized : [num_users=1] = call_function[target=torch.ops.higher_order.auto_functionalized](args = (mylib.numpy_sin.default,), kwargs = {x: %x, output: %zeros}). See the stack trace for more information.
(Refer to the full stack trace above for more information.)
Registration¶
The exporter how to convert the new exporter into ONNX. This must be defined. The first piece is to tell the exporter that the shape of the output is the same as x. input names must be the same.
@numpy_sin.register_fake
def numpy_sin_shape(x, output):
pass
Let’s see what the fx graph looks like.
print(torch.export.export(model, (x,)).graph)
graph():
%x : [num_users=1] = placeholder[target=x]
%zeros : [num_users=2] = call_function[target=torch.ops.aten.zeros.default](args = ([1, 3],), kwargs = {device: cpu, pin_memory: False})
%numpy_sin : [num_users=0] = call_function[target=torch.ops.mylib.numpy_sin.default](args = (%x, %zeros), kwargs = {})
return (zeros,)
Next is the conversion to onnx.
T = str # a tensor name
op = onnxscript.opset18
Let’s convert the custom op into onnx.
@onnxscript.script()
def numpy_sin_to_onnx(x) -> onnxscript.onnx_types.TensorType:
return op.Sin(x)
And we convert again.
try:
ep = torch.onnx.export(
model,
(x,),
custom_translation_table={torch.ops.mylib.numpy_sin.default: numpy_sin_to_onnx},
dynamo=True,
)
print(to_text(ep.model_proto))
except Exception as e:
print(f"ERROR: {e}")
[torch.onnx] Obtain model graph for `ModuleWithACustomOperator()` with `torch.export.export(..., strict=False)`...
[torch.onnx] Obtain model graph for `ModuleWithACustomOperator()` with `torch.export.export(..., strict=False)`... ✅
[torch.onnx] Run decomposition...
[torch.onnx] Run decomposition... ✅
[torch.onnx] Translate the graph into ONNX...
[torch.onnx] Translate the graph into ONNX... ❌
ERROR: Failed to convert the exported program to an ONNX model. This is step 3/3 of exporting the model to ONNX. Next steps:
- If there is a missing ONNX function, implement it and register it to the registry.
- If there is an internal error during ONNX conversion, debug the error and summit a PR to PyTorch.
- Create an error report with `torch.onnx.export(..., report=True)`, and save the ExportedProgram as a pt2 file. Create an issue in the PyTorch GitHub repository against the *onnx* component. Attach the error report and the pt2 model.
## Exception summary
<class 'torch.onnx._internal.exporter._errors.DispatchError'>: No ONNX function found for <torch._higher_order_ops.auto_functionalize.AutoFunctionalized object at 0x7f8bc0cb8530>. Failure message: No decompositions registered for the real-valued input
⬆️
<class 'torch.onnx._internal.exporter._errors.ConversionError'>: Error when translating node %auto_functionalized : [num_users=1] = call_function[target=torch.ops.higher_order.auto_functionalized](args = (mylib.numpy_sin.default,), kwargs = {x: %x, output: %zeros}). See the stack trace for more information.
(Refer to the full stack trace above for more information.)
Total running time of the script: (0 minutes 5.955 seconds)
Related examples
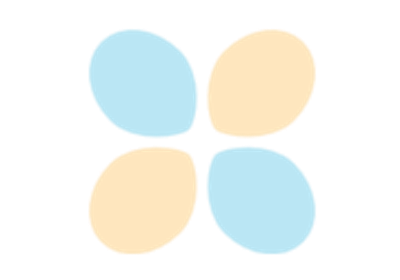
torch.onnx.export and a custom operator registered with a function
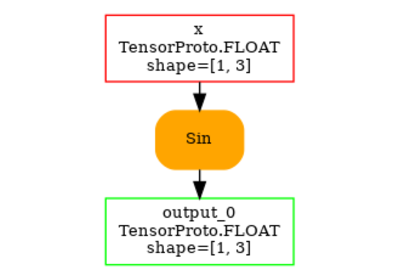
to_onnx and a custom operator registered with a function