Note
Go to the end to download the full example code.
Playground for big optimization pattern¶
# %% # Write the code producing the model # ==================================
import numpy as np
import onnx
import onnx.helper as oh
import onnx.numpy_helper as onh
from onnx_array_api.translate_api import translate
from experimental_experiment.xbuilder.reverse_graph_builder import to_graph_pattern_matching
onx = oh.make_model(
oh.make_graph(
[
oh.make_node("Cast", ["v0_0"], ["x1"], to=onnx.TensorProto.FLOAT),
oh.make_node("Cast", ["v0_0"], ["x2"], to=onnx.TensorProto.FLOAT),
oh.make_node("Flatten", ["x1"], ["f1"], axis=0),
oh.make_node("Flatten", ["x2"], ["f2"], axis=0),
oh.make_node("Concat", ["f1", "i1"], ["c1"], axis=1),
oh.make_node("Concat", ["f2", "i2"], ["c2"], axis=1),
oh.make_node("Reshape", ["c1", "s1"], ["m1"]),
oh.make_node("Reshape", ["c2", "s2"], ["m2"]),
oh.make_node("MatMul", ["m1", "m2"], ["mm"]),
oh.make_node("Identity", ["mm"], ["output"]),
],
"nd",
[oh.make_tensor_value_info("v0_0", onnx.TensorProto.DOUBLE, [5])],
[oh.make_tensor_value_info("output", onnx.TensorProto.FLOAT, [2, 3, 3, 3])],
[
onh.from_array(np.zeros((1, 49)).astype(np.float32), name="i1"),
onh.from_array(np.zeros((1, 4)).astype(np.float32), name="i2"),
onh.from_array(np.array([2, 3, 3, 3], dtype=np.int64), name="s1"),
onh.from_array(np.array([3, 3], dtype=np.int64), name="s2"),
],
),
opset_imports=[oh.make_opsetid("", 18)],
ir_version=9,
)
print(translate(onx, api="onnx-short"))
opset_imports = [
make_opsetid('', 18),
]
inputs = []
outputs = []
nodes = []
initializers = []
sparse_initializers = []
functions = []
value = np.random.randn(1, 49).astype(np.float32)
initializers.append(
from_array(
np.array(value, dtype=np.float32),
name='i1'
)
)
initializers.append(
from_array(
np.array([[0.0, 0.0, 0.0, 0.0]], dtype=np.float32),
name='i2'
)
)
initializers.append(
from_array(
np.array([2, 3, 3, 3], dtype=np.int64),
name='s1'
)
)
initializers.append(
from_array(
np.array([3, 3], dtype=np.int64),
name='s2'
)
)
inputs.append(make_tensor_value_info('v0_0', TensorProto.DOUBLE, shape=(5,)))
nodes.append(
make_node_extended(
'Cast',
['v0_0'],
['x1'],
to=1
)
)
nodes.append(
make_node_extended(
'Cast',
['v0_0'],
['x2'],
to=1
)
)
nodes.append(
make_node_extended(
'Flatten',
['x1'],
['f1'],
axis=0
)
)
nodes.append(
make_node_extended(
'Flatten',
['x2'],
['f2'],
axis=0
)
)
nodes.append(
make_node_extended(
'Concat',
['f1', 'i1'],
['c1'],
axis=1
)
)
nodes.append(
make_node_extended(
'Concat',
['f2', 'i2'],
['c2'],
axis=1
)
)
nodes.append(
make_node_extended(
'Reshape',
['c1', 's1'],
['m1']
)
)
nodes.append(
make_node_extended(
'Reshape',
['c2', 's2'],
['m2']
)
)
nodes.append(
make_node_extended(
'MatMul',
['m1', 'm2'],
['mm']
)
)
nodes.append(
make_node_extended(
'Identity',
['mm'],
['output']
)
)
outputs.append(make_tensor_value_info('output', TensorProto.FLOAT, shape=(2, 3, 3, 3)))
graph = make_graph(
nodes,
'nd',
inputs,
outputs,
initializers,
sparse_initializer=sparse_initializers,
)
model = make_model(
graph,
functions=functions,
opset_imports=opset_imports
)
Pattern Matching¶
pattern = to_graph_pattern_matching(onx)
print(pattern)
node_9_Identity = node
if node_9_Identity.op_type != 'Identity' or node_9_Identity.domain != '':
return self.none()
mm = node_9_Identity.input[0]
if g.is_used_more_than_once(mm):
return self.none(node, inspect.currentframe().f_lineno)
node_8_MatMul = g.node_before(mm)
if node_8_MatMul is None or node_8_MatMul.op_type != 'MatMul' or node_8_MatMul.domain != '':
return self.none(node, inspect.currentframe().f_lineno)
m1 = node_8_MatMul.input[0]
m2 = node_8_MatMul.input[1]
if g.is_used_more_than_once(m2):
return self.none(node, inspect.currentframe().f_lineno)
node_7_Reshape = g.node_before(m2)
if node_7_Reshape is None or node_7_Reshape.op_type != 'Reshape' or node_7_Reshape.domain != '':
return self.none(node, inspect.currentframe().f_lineno)
c2 = node_7_Reshape.input[0]
s2 = node_7_Reshape.input[1]
# s2 has no predecessor.
if g.is_used_more_than_once(c2):
return self.none(node, inspect.currentframe().f_lineno)
node_5_Concat = g.node_before(c2)
if node_5_Concat is None or node_5_Concat.op_type != 'Concat' or node_5_Concat.domain != '':
return self.none(node, inspect.currentframe().f_lineno)
f2 = node_5_Concat.input[0]
i2 = node_5_Concat.input[1]
# i2 has no predecessor.
if g.is_used_more_than_once(f2):
return self.none(node, inspect.currentframe().f_lineno)
node_3_Flatten = g.node_before(f2)
if node_3_Flatten is None or node_3_Flatten.op_type != 'Flatten' or node_3_Flatten.domain != '':
return self.none(node, inspect.currentframe().f_lineno)
x2 = node_3_Flatten.input[0]
if g.is_used_more_than_once(x2):
return self.none(node, inspect.currentframe().f_lineno)
node_1_Cast = g.node_before(x2)
if node_1_Cast is None or node_1_Cast.op_type != 'Cast' or node_1_Cast.domain != '':
return self.none(node, inspect.currentframe().f_lineno)
v0_0 = node_1_Cast.input[0]
# v0_0 has no predecessor.
if g.is_used_more_than_once(m1):
return self.none(node, inspect.currentframe().f_lineno)
node_6_Reshape = g.node_before(m1)
if node_6_Reshape is None or node_6_Reshape.op_type != 'Reshape' or node_6_Reshape.domain != '':
return self.none(node, inspect.currentframe().f_lineno)
c1 = node_6_Reshape.input[0]
s1 = node_6_Reshape.input[1]
# s1 has no predecessor.
if g.is_used_more_than_once(c1):
return self.none(node, inspect.currentframe().f_lineno)
node_4_Concat = g.node_before(c1)
if node_4_Concat is None or node_4_Concat.op_type != 'Concat' or node_4_Concat.domain != '':
return self.none(node, inspect.currentframe().f_lineno)
f1 = node_4_Concat.input[0]
i1 = node_4_Concat.input[1]
# i1 has no predecessor.
if g.is_used_more_than_once(f1):
return self.none(node, inspect.currentframe().f_lineno)
node_2_Flatten = g.node_before(f1)
if node_2_Flatten is None or node_2_Flatten.op_type != 'Flatten' or node_2_Flatten.domain != '':
return self.none(node, inspect.currentframe().f_lineno)
x1 = node_2_Flatten.input[0]
if g.is_used_more_than_once(x1):
return self.none(node, inspect.currentframe().f_lineno)
node_0_Cast = g.node_before(x1)
if node_0_Cast is None or node_0_Cast.op_type != 'Cast' or node_0_Cast.domain != '':
return self.none(node, inspect.currentframe().f_lineno)
v0_0 = node_0_Cast.input[0]
# v0_0 has no predecessor.
# list of nodes
nodes = [node_0_Cast, node_2_Flatten, node_4_Concat, node_6_Reshape, node_1_Cast, node_3_Flatten, node_5_Concat, node_7_Reshape, node_8_MatMul, node_9_Identity]
Total running time of the script: (0 minutes 0.006 seconds)
Related examples
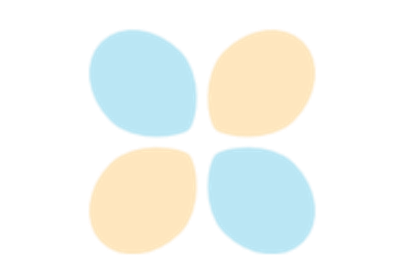
201: Use torch to export a scikit-learn model into ONNX
201: Use torch to export a scikit-learn model into ONNX