Note
Go to the end to download the full example code.
Export Phi-3.5-mini-instruct piece by piece¶
torch.export.export()
often breaks on big models because there
are control flows or instructions breaking the propagation of
dynamic shapes (see …). The function usually gives an indication where
the model implementation can be fixed but in case, that is not possible,
we can try to export the model piece by piece: every module
is converted separately from its submodule. A model can be exported even
if one of its submodules cannot.
Model¶
import pprint
from typing import Any, Dict
import torch
import torch._export.tools
import transformers
from experimental_experiment.helpers import string_type
from experimental_experiment.torch_interpreter.piece_by_piece import (
trace_execution_piece_by_piece,
)
def get_phi35_untrained(batch_size: int = 2, **kwargs) -> Dict[str, Any]:
"""
Gets a non initialized model with two sets of inputs and different shapes.
:param batch_size: batch size
:param kwargs: to overwrite the configuration, example ``num_hidden_layers=1``
:return: dictionary
See `Phi-3.5-mini-instruct/config.json
<https://huggingface.co/microsoft/Phi-3.5-mini-instruct/blob/main/config.json>`_.
"""
config = {
"_name_or_path": "Phi-3.5-mini-instruct",
"architectures": ["Phi3ForCausalLM"],
"attention_dropout": 0.0,
"auto_map": {
"AutoConfig": "configuration_phi3.Phi3Config",
"AutoModelForCausalLM": "modeling_phi3.Phi3ForCausalLM",
},
"bos_token_id": 1,
"embd_pdrop": 0.0,
"eos_token_id": 32000,
"hidden_act": "silu",
"hidden_size": 3072,
"initializer_range": 0.02,
"intermediate_size": 8192,
"max_position_embeddings": 131072,
"model_type": "phi3",
"num_attention_heads": 32,
"num_hidden_layers": 32,
"num_key_value_heads": 32,
"original_max_position_embeddings": 4096,
"pad_token_id": 32000,
"resid_pdrop": 0.0,
"rms_norm_eps": 1e-05,
"rope_scaling": {
"long_factor": [
1.0800000429153442,
1.1100000143051147,
1.1399999856948853,
1.340000033378601,
1.5899999141693115,
1.600000023841858,
1.6200000047683716,
2.620000123977661,
3.2300000190734863,
3.2300000190734863,
4.789999961853027,
7.400000095367432,
7.700000286102295,
9.09000015258789,
12.199999809265137,
17.670000076293945,
24.46000099182129,
28.57000160217285,
30.420001983642578,
30.840002059936523,
32.590003967285156,
32.93000411987305,
42.320003509521484,
44.96000289916992,
50.340003967285156,
50.45000457763672,
57.55000305175781,
57.93000411987305,
58.21000289916992,
60.1400032043457,
62.61000442504883,
62.62000274658203,
62.71000289916992,
63.1400032043457,
63.1400032043457,
63.77000427246094,
63.93000411987305,
63.96000289916992,
63.970001220703125,
64.02999877929688,
64.06999969482422,
64.08000183105469,
64.12000274658203,
64.41000366210938,
64.4800033569336,
64.51000213623047,
64.52999877929688,
64.83999633789062,
],
"short_factor": [
1.0,
1.0199999809265137,
1.0299999713897705,
1.0299999713897705,
1.0499999523162842,
1.0499999523162842,
1.0499999523162842,
1.0499999523162842,
1.0499999523162842,
1.0699999332427979,
1.0999999046325684,
1.1099998950958252,
1.1599998474121094,
1.1599998474121094,
1.1699998378753662,
1.2899998426437378,
1.339999794960022,
1.679999828338623,
1.7899998426437378,
1.8199998140335083,
1.8499997854232788,
1.8799997568130493,
1.9099997282028198,
1.9399996995925903,
1.9899996519088745,
2.0199997425079346,
2.0199997425079346,
2.0199997425079346,
2.0199997425079346,
2.0199997425079346,
2.0199997425079346,
2.0299997329711914,
2.0299997329711914,
2.0299997329711914,
2.0299997329711914,
2.0299997329711914,
2.0299997329711914,
2.0299997329711914,
2.0299997329711914,
2.0299997329711914,
2.0799996852874756,
2.0899996757507324,
2.189999580383301,
2.2199995517730713,
2.5899994373321533,
2.729999542236328,
2.749999523162842,
2.8399994373321533,
],
"type": "longrope",
},
"rope_theta": 10000.0,
"sliding_window": 262144,
"tie_word_embeddings": False,
"torch_dtype": "bfloat16",
"use_cache": True,
"attention_bias": False,
"vocab_size": 32064,
}
config.update(**kwargs)
conf = transformers.Phi3Config(**config)
model = transformers.Phi3ForCausalLM(conf)
model.eval()
cache = transformers.cache_utils.DynamicCache(config["num_hidden_layers"])
for i in range(config["num_hidden_layers"]):
cache.update(
torch.randn(batch_size, 32, 30, 96), torch.randn(batch_size, 32, 30, 96), i
)
cache2 = transformers.cache_utils.DynamicCache(config["num_hidden_layers"])
for i in range(config["num_hidden_layers"]):
cache2.update(
torch.randn(batch_size + 1, 32, 31, 96),
torch.randn(batch_size + 1, 32, 31, 96),
i,
)
inputs = dict(
input_ids=torch.randint(0, 32064, (batch_size, 3)).to(torch.int64),
attention_mask=torch.ones((batch_size, 33)).to(torch.int64),
past_key_values=cache,
)
inputs2 = dict(
input_ids=torch.randint(0, 32064, (batch_size + 1, 4)).to(torch.int64),
attention_mask=torch.ones((batch_size + 1, 35)).to(torch.int64),
past_key_values=cache2,
)
return dict(inputs=inputs, model=model, inputs2=inputs2)
data = get_phi35_untrained(num_hidden_layers=2)
model, inputs, inputs2 = data["model"], data["inputs"], data["inputs2"]
print(string_type(inputs, with_shape=True))
dict(input_ids:T7s2x3,attention_mask:T7s2x33,past_key_values:DynamicCache(key_cache=#2[T1s2x32x30x96,T1s2x32x30x96], value_cache=#2[T1s2x32x30x96,T1s2x32x30x96]))
Dynamic Shapes¶
We want to infer the dynamic shapes from the two sets of inputs we gave. For that, we use a function to trace the execution of the model including its submodules. It is going to execute the model twice with the two sets of inputs and stores every intermediate input and output.
diag = trace_execution_piece_by_piece(model, [inputs, inputs2], verbose=2)
[_trace_forward_execution] -trace- M:__main__-Phi3ForCausalLM.forward
[_trace_forward_execution] -trace- .. M:model-Phi3Model.forward
[_trace_forward_execution] -trace- .... M:embed_tokens-Embedding.forward
[_trace_forward_execution] -trace- .... M:layers[0]-Phi3DecoderLayer.forward
[_trace_forward_execution] -trace- ...... M:self_attn-Phi3Attention.forward
[_trace_forward_execution] -trace- ........ M:o_proj-Linear.forward
[_trace_forward_execution] -trace- ........ M:qkv_proj-Linear.forward
[_trace_forward_execution] -trace- ...... M:mlp-Phi3MLP.forward
[_trace_forward_execution] -trace- ........ M:gate_up_proj-Linear.forward
[_trace_forward_execution] -trace- ........ M:down_proj-Linear.forward
[_trace_forward_execution] -trace- ........ M:activation_fn-SiLU.forward
[_trace_forward_execution] -trace- ...... M:input_layernorm-Phi3RMSNorm.forward
[_trace_forward_execution] -trace- ...... M:post_attention_layernorm-Phi3RMSNorm.forward
[_trace_forward_execution] -trace- ...... M:resid_attn_dropout-Dropout.forward
[_trace_forward_execution] -trace- ...... M:resid_mlp_dropout-Dropout.forward
[_trace_forward_execution] -trace- .... M:layers[1]-Phi3DecoderLayer.forward
[_trace_forward_execution] -trace- ...... M:self_attn-Phi3Attention.forward
[_trace_forward_execution] -trace- ........ M:o_proj-Linear.forward
[_trace_forward_execution] -trace- ........ M:qkv_proj-Linear.forward
[_trace_forward_execution] -trace- ...... M:mlp-Phi3MLP.forward
[_trace_forward_execution] -trace- ........ M:gate_up_proj-Linear.forward
[_trace_forward_execution] -trace- ........ M:down_proj-Linear.forward
[_trace_forward_execution] -trace- ........ M:activation_fn-SiLU.forward
[_trace_forward_execution] -trace- ...... M:input_layernorm-Phi3RMSNorm.forward
[_trace_forward_execution] -trace- ...... M:post_attention_layernorm-Phi3RMSNorm.forward
[_trace_forward_execution] -trace- ...... M:resid_attn_dropout-Dropout.forward
[_trace_forward_execution] -trace- ...... M:resid_mlp_dropout-Dropout.forward
[_trace_forward_execution] -trace- .... M:norm-Phi3RMSNorm.forward
[_trace_forward_execution] -trace- .... M:rotary_emb-Phi3RotaryEmbedding.forward
[_trace_forward_execution] -trace- .. M:lm_head-Linear.forward
[trace_execution_piece_by_piece] run with dict(args:(),kwargs:dict(input_ids:T7s2x3,attention_mask:T7s2x33,past_key_values:DynamicCache(key_cache=#2[T1s2x32x30x96,T1s2x32x30x96], value_cache=#2[T1s2x32x30x96,T1s2x32x30x96])))
[__main__:Phi3ForCausalLM] > **dict(input_ids:T7r2,attention_mask:T7r2,past_key_values:DynamicCache(key_cache=#2[T1r4,T1r4], value_cache=#2[T1r4,T1r4]))
[model:Phi3Model] > **dict(input_ids:T7r2,attention_mask:T7r2,position_ids:None,past_key_values:DynamicCache(key_cache=#2[T1r4,T1r4], value_cache=#2[T1r4,T1r4]),inputs_embeds:None,use_cache:None,output_attentions:bool,output_hidden_states:bool,return_dict:bool,cache_position:None)
[embed_tokens:Embedding] > T7r2
[embed_tokens:Embedding] < T1r3
[rotary_emb:Phi3RotaryEmbedding] > *(T1r3,T7r2)
[rotary_emb:Phi3RotaryEmbedding] < *(T1r3,T1r3)
[layers[0]:Phi3DecoderLayer] > *(T1r3,), **dict(attention_mask:T1r4,position_ids:T7r2,past_key_value:DynamicCache(key_cache=#2[T1r4,T1r4], value_cache=#2[T1r4,T1r4]),output_attentions:bool,use_cache:bool,cache_position:T7r1,position_embeddings:(T1r3,T1r3))
[input_layernorm:Phi3RMSNorm] > T1r3
[input_layernorm:Phi3RMSNorm] < T1r3
[self_attn:Phi3Attention] > **dict(hidden_states:T1r3,attention_mask:T1r4,position_ids:T7r2,past_key_value:DynamicCache(key_cache=#2[T1r4,T1r4], value_cache=#2[T1r4,T1r4]),output_attentions:bool,use_cache:bool,cache_position:T7r1,position_embeddings:(T1r3,T1r3))
[qkv_proj:Linear] > T1r3
[qkv_proj:Linear] < T1r3
[o_proj:Linear] > T1r3
[o_proj:Linear] < T1r3
[self_attn:Phi3Attention] < *(T1r3,None)
[resid_attn_dropout:Dropout] > T1r3
[resid_attn_dropout:Dropout] < T1r3
[post_attention_layernorm:Phi3RMSNorm] > T1r3
[post_attention_layernorm:Phi3RMSNorm] < T1r3
[mlp:Phi3MLP] > T1r3
[gate_up_proj:Linear] > T1r3
[gate_up_proj:Linear] < T1r3
[activation_fn:SiLU] > T1r3
[activation_fn:SiLU] < T1r3
[down_proj:Linear] > T1r3
[down_proj:Linear] < T1r3
[mlp:Phi3MLP] < T1r3
[resid_mlp_dropout:Dropout] > T1r3
[resid_mlp_dropout:Dropout] < T1r3
[layers[0]:Phi3DecoderLayer] < *(T1r3,)
[layers[1]:Phi3DecoderLayer] > *(T1r3,), **dict(attention_mask:T1r4,position_ids:T7r2,past_key_value:DynamicCache(key_cache=#2[T1r4,T1r4], value_cache=#2[T1r4,T1r4]),output_attentions:bool,use_cache:bool,cache_position:T7r1,position_embeddings:(T1r3,T1r3))
[input_layernorm:Phi3RMSNorm] > T1r3
[input_layernorm:Phi3RMSNorm] < T1r3
[self_attn:Phi3Attention] > **dict(hidden_states:T1r3,attention_mask:T1r4,position_ids:T7r2,past_key_value:DynamicCache(key_cache=#2[T1r4,T1r4], value_cache=#2[T1r4,T1r4]),output_attentions:bool,use_cache:bool,cache_position:T7r1,position_embeddings:(T1r3,T1r3))
[qkv_proj:Linear] > T1r3
[qkv_proj:Linear] < T1r3
[o_proj:Linear] > T1r3
[o_proj:Linear] < T1r3
[self_attn:Phi3Attention] < *(T1r3,None)
[resid_attn_dropout:Dropout] > T1r3
[resid_attn_dropout:Dropout] < T1r3
[post_attention_layernorm:Phi3RMSNorm] > T1r3
[post_attention_layernorm:Phi3RMSNorm] < T1r3
[mlp:Phi3MLP] > T1r3
[gate_up_proj:Linear] > T1r3
[gate_up_proj:Linear] < T1r3
[activation_fn:SiLU] > T1r3
[activation_fn:SiLU] < T1r3
[down_proj:Linear] > T1r3
[down_proj:Linear] < T1r3
[mlp:Phi3MLP] < T1r3
[resid_mlp_dropout:Dropout] > T1r3
[resid_mlp_dropout:Dropout] < T1r3
[layers[1]:Phi3DecoderLayer] < *(T1r3,)
[norm:Phi3RMSNorm] > T1r3
[norm:Phi3RMSNorm] < T1r3
[model:Phi3Model] < *dict(last_hidden_state:T1r3,past_key_values:DynamicCache(key_cache=#2[T1r4,T1r4], value_cache=#2[T1r4,T1r4]))
[lm_head:Linear] > T1r3
[lm_head:Linear] < T1r3
[__main__:Phi3ForCausalLM] < *dict(logits:T1r3,past_key_values:DynamicCache(key_cache=#2[T1r4,T1r4], value_cache=#2[T1r4,T1r4]))
[trace_execution_piece_by_piece] run with dict(args:(),kwargs:dict(input_ids:T7s3x4,attention_mask:T7s3x35,past_key_values:DynamicCache(key_cache=#2[T1s3x32x31x96,T1s3x32x31x96], value_cache=#2[T1s3x32x31x96,T1s3x32x31x96])))
[__main__:Phi3ForCausalLM] > **dict(input_ids:T7r2,attention_mask:T7r2,past_key_values:DynamicCache(key_cache=#2[T1r4,T1r4], value_cache=#2[T1r4,T1r4]))
[model:Phi3Model] > **dict(input_ids:T7r2,attention_mask:T7r2,position_ids:None,past_key_values:DynamicCache(key_cache=#2[T1r4,T1r4], value_cache=#2[T1r4,T1r4]),inputs_embeds:None,use_cache:None,output_attentions:bool,output_hidden_states:bool,return_dict:bool,cache_position:None)
[embed_tokens:Embedding] > T7r2
[embed_tokens:Embedding] < T1r3
[rotary_emb:Phi3RotaryEmbedding] > *(T1r3,T7r2)
[rotary_emb:Phi3RotaryEmbedding] < *(T1r3,T1r3)
[layers[0]:Phi3DecoderLayer] > *(T1r3,), **dict(attention_mask:T1r4,position_ids:T7r2,past_key_value:DynamicCache(key_cache=#2[T1r4,T1r4], value_cache=#2[T1r4,T1r4]),output_attentions:bool,use_cache:bool,cache_position:T7r1,position_embeddings:(T1r3,T1r3))
[input_layernorm:Phi3RMSNorm] > T1r3
[input_layernorm:Phi3RMSNorm] < T1r3
[self_attn:Phi3Attention] > **dict(hidden_states:T1r3,attention_mask:T1r4,position_ids:T7r2,past_key_value:DynamicCache(key_cache=#2[T1r4,T1r4], value_cache=#2[T1r4,T1r4]),output_attentions:bool,use_cache:bool,cache_position:T7r1,position_embeddings:(T1r3,T1r3))
[qkv_proj:Linear] > T1r3
[qkv_proj:Linear] < T1r3
[o_proj:Linear] > T1r3
[o_proj:Linear] < T1r3
[self_attn:Phi3Attention] < *(T1r3,None)
[resid_attn_dropout:Dropout] > T1r3
[resid_attn_dropout:Dropout] < T1r3
[post_attention_layernorm:Phi3RMSNorm] > T1r3
[post_attention_layernorm:Phi3RMSNorm] < T1r3
[mlp:Phi3MLP] > T1r3
[gate_up_proj:Linear] > T1r3
[gate_up_proj:Linear] < T1r3
[activation_fn:SiLU] > T1r3
[activation_fn:SiLU] < T1r3
[down_proj:Linear] > T1r3
[down_proj:Linear] < T1r3
[mlp:Phi3MLP] < T1r3
[resid_mlp_dropout:Dropout] > T1r3
[resid_mlp_dropout:Dropout] < T1r3
[layers[0]:Phi3DecoderLayer] < *(T1r3,)
[layers[1]:Phi3DecoderLayer] > *(T1r3,), **dict(attention_mask:T1r4,position_ids:T7r2,past_key_value:DynamicCache(key_cache=#2[T1r4,T1r4], value_cache=#2[T1r4,T1r4]),output_attentions:bool,use_cache:bool,cache_position:T7r1,position_embeddings:(T1r3,T1r3))
[input_layernorm:Phi3RMSNorm] > T1r3
[input_layernorm:Phi3RMSNorm] < T1r3
[self_attn:Phi3Attention] > **dict(hidden_states:T1r3,attention_mask:T1r4,position_ids:T7r2,past_key_value:DynamicCache(key_cache=#2[T1r4,T1r4], value_cache=#2[T1r4,T1r4]),output_attentions:bool,use_cache:bool,cache_position:T7r1,position_embeddings:(T1r3,T1r3))
[qkv_proj:Linear] > T1r3
[qkv_proj:Linear] < T1r3
[o_proj:Linear] > T1r3
[o_proj:Linear] < T1r3
[self_attn:Phi3Attention] < *(T1r3,None)
[resid_attn_dropout:Dropout] > T1r3
[resid_attn_dropout:Dropout] < T1r3
[post_attention_layernorm:Phi3RMSNorm] > T1r3
[post_attention_layernorm:Phi3RMSNorm] < T1r3
[mlp:Phi3MLP] > T1r3
[gate_up_proj:Linear] > T1r3
[gate_up_proj:Linear] < T1r3
[activation_fn:SiLU] > T1r3
[activation_fn:SiLU] < T1r3
[down_proj:Linear] > T1r3
[down_proj:Linear] < T1r3
[mlp:Phi3MLP] < T1r3
[resid_mlp_dropout:Dropout] > T1r3
[resid_mlp_dropout:Dropout] < T1r3
[layers[1]:Phi3DecoderLayer] < *(T1r3,)
[norm:Phi3RMSNorm] > T1r3
[norm:Phi3RMSNorm] < T1r3
[model:Phi3Model] < *dict(last_hidden_state:T1r3,past_key_values:DynamicCache(key_cache=#2[T1r4,T1r4], value_cache=#2[T1r4,T1r4]))
[lm_head:Linear] > T1r3
[lm_head:Linear] < T1r3
[__main__:Phi3ForCausalLM] < *dict(logits:T1r3,past_key_values:DynamicCache(key_cache=#2[T1r4,T1r4], value_cache=#2[T1r4,T1r4]))
[trace_forward_execution] traced execution of model Phi3ForCausalLM
>>> __main__: Phi3ForCausalLM
> ((),dict(input_ids:CT7s2x3[7675,21707:A14320.666666666666],attention_mask:CT7s2x33[1,1:A1.0],past_key_values:DynamicCache(key_cache=#2[CT1s2x32x30x96[-4.143186092376709,4.480688571929932:A0.0008577694740877779],CT1s2x32x30x96[-4.347227573394775,4.55678129196167:A-0.00033970271848547825]], value_cache=#2[CT1s2x32x30x96[-4.442789077758789,4.48254919052124:A0.0014336849355417532],CT1s2x32x30x96[-4.140044689178467,4.500824451446533:A0.004017313765510237]])))
> ((),dict(input_ids:CT7s3x4[830,28543:A13849.083333333334],attention_mask:CT7s3x35[1,1:A1.0],past_key_values:DynamicCache(key_cache=#2[CT1s3x32x31x96[-4.465207099914551,4.81513786315918:A0.002684910137815105],CT1s3x32x31x96[-4.275788307189941,5.158080101013184:A-0.00010541313345598764]], value_cache=#2[CT1s3x32x31x96[-4.699131011962891,4.6095075607299805:A0.0034093885894590815],CT1s3x32x31x96[-4.692654132843018,4.596354007720947:A0.0011176538469116694]])))
>>> model: Phi3Model
> ((),dict(input_ids:CT7s2x3[7675,21707:A14320.666666666666],attention_mask:CT7s2x33[1,1:A1.0],position_ids:None,past_key_values:DynamicCache(key_cache=#2[CT1s2x32x30x96[-4.143186092376709,4.480688571929932:A0.0008577694740877779],CT1s2x32x30x96[-4.347227573394775,4.55678129196167:A-0.00033970271848547825]], value_cache=#2[CT1s2x32x30x96[-4.442789077758789,4.48254919052124:A0.0014336849355417532],CT1s2x32x30x96[-4.140044689178467,4.500824451446533:A0.004017313765510237]]),inputs_embeds:None,use_cache:None,output_attentions:bool=False,output_hidden_states:bool=False,return_dict:bool=True,cache_position:None))
> ((),dict(input_ids:CT7s3x4[830,28543:A13849.083333333334],attention_mask:CT7s3x35[1,1:A1.0],position_ids:None,past_key_values:DynamicCache(key_cache=#2[CT1s3x32x31x96[-4.465207099914551,4.81513786315918:A0.002684910137815105],CT1s3x32x31x96[-4.275788307189941,5.158080101013184:A-0.00010541313345598764]], value_cache=#2[CT1s3x32x31x96[-4.699131011962891,4.6095075607299805:A0.0034093885894590815],CT1s3x32x31x96[-4.692654132843018,4.596354007720947:A0.0011176538469116694]]),inputs_embeds:None,use_cache:None,output_attentions:bool=False,output_hidden_states:bool=False,return_dict:bool=True,cache_position:None))
>>> embed_tokens: Embedding
> ((CT7s2x3[7675,21707:A14320.666666666666],),{})
> ((CT7s3x4[830,28543:A13849.083333333334],),{})
< (CT1s2x3x3072[-0.0821705013513565,0.07243882864713669:A2.9103279443904203e-05],)
< (CT1s3x4x3072[-0.07996544241905212,0.07827455550432205:A1.0599782303485134e-05],)
<<<
>>> layers[0]: Phi3DecoderLayer
> ((CT1s2x3x3072[-0.0821705013513565,0.07243882864713669:A2.9103279443904203e-05],),dict(attention_mask:CT1s2x1x3x33[-3.4028234663852886e+38,-0.0:A-1.0311586261773601e+37],position_ids:CT7s1x3[30,32:A31.0],past_key_value:DynamicCache(key_cache=#2[CT1s2x32x30x96[-4.143186092376709,4.480688571929932:A0.0008577694740877779],CT1s2x32x30x96[-4.347227573394775,4.55678129196167:A-0.00033970271848547825]], value_cache=#2[CT1s2x32x30x96[-4.442789077758789,4.48254919052124:A0.0014336849355417532],CT1s2x32x30x96[-4.140044689178467,4.500824451446533:A0.004017313765510237]]),output_attentions:bool=False,use_cache:bool=True,cache_position:CT7s3[30,32:A31.0],position_embeddings:(CT1s1x3x96[-1.1855769157409668,1.1902371644973755:A0.746652018013669],CT1s1x3x96[-1.1887905597686768,1.190193772315979:A0.1589894221542636])))
> ((CT1s3x4x3072[-0.07996544241905212,0.07827455550432205:A1.0599782303485134e-05],),dict(attention_mask:CT1s3x1x4x35[-3.4028234663852886e+38,-0.0:A-1.4583529141651237e+37],position_ids:CT7s1x4[31,34:A32.5],past_key_value:DynamicCache(key_cache=#2[CT1s3x32x31x96[-4.465207099914551,4.81513786315918:A0.002684910137815105],CT1s3x32x31x96[-4.275788307189941,5.158080101013184:A-0.00010541313345598764]], value_cache=#2[CT1s3x32x31x96[-4.699131011962891,4.6095075607299805:A0.0034093885894590815],CT1s3x32x31x96[-4.692654132843018,4.596354007720947:A0.0011176538469116694]]),output_attentions:bool=False,use_cache:bool=True,cache_position:CT7s4[31,34:A32.5],position_embeddings:(CT1s1x4x96[-1.1855769157409668,1.190237045288086:A0.7129333875218435],CT1s1x4x96[-1.1719439029693604,1.1902378797531128:A0.18296290554159592])))
>>> self_attn: Phi3Attention
> ((),dict(hidden_states:CT1s2x3x3072[-4.047475814819336,3.568122386932373:A0.0014399883224896924],attention_mask:CT1s2x1x3x33[-3.4028234663852886e+38,-0.0:A-1.0311586261773601e+37],position_ids:CT7s1x3[30,32:A31.0],past_key_value:DynamicCache(key_cache=#2[CT1s2x32x30x96[-4.143186092376709,4.480688571929932:A0.0008577694740877779],CT1s2x32x30x96[-4.347227573394775,4.55678129196167:A-0.00033970271848547825]], value_cache=#2[CT1s2x32x30x96[-4.442789077758789,4.48254919052124:A0.0014336849355417532],CT1s2x32x30x96[-4.140044689178467,4.500824451446533:A0.004017313765510237]]),output_attentions:bool=False,use_cache:bool=True,cache_position:CT7s3[30,32:A31.0],position_embeddings:(CT1s1x3x96[-1.1855769157409668,1.1902371644973755:A0.746652018013669],CT1s1x3x96[-1.1887905597686768,1.190193772315979:A0.1589894221542636])))
> ((),dict(hidden_states:CT1s3x4x3072[-3.9000232219696045,3.8280856609344482:A0.0005184027855419012],attention_mask:CT1s3x1x4x35[-3.4028234663852886e+38,-0.0:A-1.4583529141651237e+37],position_ids:CT7s1x4[31,34:A32.5],past_key_value:DynamicCache(key_cache=#2[CT1s3x32x31x96[-4.465207099914551,4.81513786315918:A0.002684910137815105],CT1s3x32x31x96[-4.275788307189941,5.158080101013184:A-0.00010541313345598764]], value_cache=#2[CT1s3x32x31x96[-4.699131011962891,4.6095075607299805:A0.0034093885894590815],CT1s3x32x31x96[-4.692654132843018,4.596354007720947:A0.0011176538469116694]]),output_attentions:bool=False,use_cache:bool=True,cache_position:CT7s4[31,34:A32.5],position_embeddings:(CT1s1x4x96[-1.1855769157409668,1.190237045288086:A0.7129333875218435],CT1s1x4x96[-1.1719439029693604,1.1902378797531128:A0.18296290554159592])))
>>> o_proj: Linear
> ((CT1s2x3x3072[-2.022639513015747,2.1115992069244385:A0.000877390108562606],),{})
> ((CT1s3x4x3072[-2.536278486251831,2.6110496520996094:A0.0015839050395390784],),{})
< (CT1s2x3x3072[-1.5196510553359985,1.3292466402053833:A0.0018243379798579757],)
< (CT1s3x4x3072[-1.7035083770751953,1.8120808601379395:A-0.0009211854248576401],)
<<<
>>> qkv_proj: Linear
> ((CT1s2x3x3072[-4.047475814819336,3.568122386932373:A0.0014399883224896924],),{})
> ((CT1s3x4x3072[-3.9000232219696045,3.8280856609344482:A0.0005184027855419012],),{})
< (CT1s2x3x9216[-4.4089035987854,5.01821231842041:A0.004201373171112108],)
< (CT1s3x4x9216[-4.953425407409668,4.941999912261963:A0.0007640326514762011],)
<<<
< (CT1s2x3x3072[-1.5196510553359985,1.3292466402053833:A0.0018243379798579757],None)
< (CT1s3x4x3072[-1.7035083770751953,1.8120808601379395:A-0.0009211854248576401],None)
<<<
>>> mlp: Phi3MLP
> ((CT1s2x3x3072[-4.038560390472412,3.5235748291015625:A0.004950067032954111],),{})
> ((CT1s3x4x3072[-4.022910118103027,4.3432416915893555:A-0.0031142394036321403],),{})
>>> gate_up_proj: Linear
> ((CT1s2x3x3072[-4.038560390472412,3.5235748291015625:A0.004950067032954111],),{})
> ((CT1s3x4x3072[-4.022910118103027,4.3432416915893555:A-0.0031142394036321403],),{})
< (CT1s2x3x16384[-4.77255916595459,5.009945392608643:A-9.592560125781802e-05],)
< (CT1s3x4x16384[-4.959973335266113,5.325800895690918:A0.001419190028326393],)
<<<
>>> down_proj: Linear
> ((CT1s2x3x8192[-8.703527450561523,8.217426300048828:A-0.0015533265535950525],),{})
> ((CT1s3x4x8192[-9.443089485168457,10.611668586730957:A0.0017220513075193132],),{})
< (CT1s2x3x3072[-5.378640174865723,5.69869327545166:A-0.007589203342402268],)
< (CT1s3x4x3072[-5.333456039428711,5.4323859214782715:A0.011663692468333566],)
<<<
>>> activation_fn: SiLU
> ((CT1s2x3x8192[-4.77255916595459,5.009945392608643:A-0.006894949193882856],),{})
> ((CT1s3x4x8192[-4.749669075012207,4.572937965393066:A0.0014222971228032104],),{})
< (CT1s2x3x8192[-0.27846455574035645,4.976744174957275:A0.24378813599679394],)
< (CT1s3x4x8192[-0.27846455574035645,4.526193141937256:A0.24585226157759354],)
<<<
< (CT1s2x3x3072[-5.378640174865723,5.69869327545166:A-0.007589203342402268],)
< (CT1s3x4x3072[-5.333456039428711,5.4323859214782715:A0.011663692468333566],)
<<<
>>> input_layernorm: Phi3RMSNorm
> ((CT1s2x3x3072[-0.0821705013513565,0.07243882864713669:A2.9103279443904203e-05],),{})
> ((CT1s3x4x3072[-0.07996544241905212,0.07827455550432205:A1.0599782303485134e-05],),{})
< (CT1s2x3x3072[-4.047475814819336,3.568122386932373:A0.0014399883224896924],)
< (CT1s3x4x3072[-3.9000232219696045,3.8280856609344482:A0.0005184027855419012],)
<<<
>>> post_attention_layernorm: Phi3RMSNorm
> ((CT1s2x3x3072[-1.5411796569824219,1.2933858633041382:A0.0018534411310295379],),{})
> ((CT1s3x4x3072[-1.6927239894866943,1.7622013092041016:A-0.0009105856577388888],),{})
< (CT1s2x3x3072[-4.038560390472412,3.5235748291015625:A0.004950067032954111],)
< (CT1s3x4x3072[-4.022910118103027,4.3432416915893555:A-0.0031142394036321403],)
<<<
>>> resid_attn_dropout: Dropout
> ((CT1s2x3x3072[-1.5196510553359985,1.3292466402053833:A0.0018243379798579757],),{})
> ((CT1s3x4x3072[-1.7035083770751953,1.8120808601379395:A-0.0009211854248576401],),{})
< (CT1s2x3x3072[-1.5196510553359985,1.3292466402053833:A0.0018243379798579757],)
< (CT1s3x4x3072[-1.7035083770751953,1.8120808601379395:A-0.0009211854248576401],)
<<<
>>> resid_mlp_dropout: Dropout
> ((CT1s2x3x3072[-5.378640174865723,5.69869327545166:A-0.007589203342402268],),{})
> ((CT1s3x4x3072[-5.333456039428711,5.4323859214782715:A0.011663692468333566],),{})
< (CT1s2x3x3072[-5.378640174865723,5.69869327545166:A-0.007589203342402268],)
< (CT1s3x4x3072[-5.333456039428711,5.4323859214782715:A0.011663692468333566],)
<<<
< (CT1s2x3x3072[-4.941531181335449,6.185527801513672:A-0.005735762384069353],)
< (CT1s3x4x3072[-5.470054626464844,5.551934719085693:A0.010753106478622007],)
<<<
>>> layers[1]: Phi3DecoderLayer
> ((CT1s2x3x3072[-4.941531181335449,6.185527801513672:A-0.005735762384069353],),dict(attention_mask:CT1s2x1x3x33[-3.4028234663852886e+38,-0.0:A-1.0311586261773601e+37],position_ids:CT7s1x3[30,32:A31.0],past_key_value:DynamicCache(key_cache=#2[CT1s2x32x33x96[-5.372235298156738,5.624973297119141:A0.001415606942792894],CT1s2x32x30x96[-4.347227573394775,4.55678129196167:A-0.00033970271848547825]], value_cache=#2[CT1s2x32x33x96[-4.442789077758789,4.48254919052124:A0.0016316771621383164],CT1s2x32x30x96[-4.140044689178467,4.500824451446533:A0.004017313765510237]]),output_attentions:bool=False,use_cache:bool=True,cache_position:CT7s3[30,32:A31.0],position_embeddings:(CT1s1x3x96[-1.1855769157409668,1.1902371644973755:A0.746652018013669],CT1s1x3x96[-1.1887905597686768,1.190193772315979:A0.1589894221542636])))
> ((CT1s3x4x3072[-5.470054626464844,5.551934719085693:A0.010753106478622007],),dict(attention_mask:CT1s3x1x4x35[-3.4028234663852886e+38,-0.0:A-1.4583529141651237e+37],position_ids:CT7s1x4[31,34:A32.5],past_key_value:DynamicCache(key_cache=#2[CT1s3x32x35x96[-5.197488307952881,5.8184494972229:A0.00265941596204836],CT1s3x32x31x96[-4.275788307189941,5.158080101013184:A-0.00010541313345598764]], value_cache=#2[CT1s3x32x35x96[-4.953425407409668,4.6095075607299805:A0.0024469109531196453],CT1s3x32x31x96[-4.692654132843018,4.596354007720947:A0.0011176538469116694]]),output_attentions:bool=False,use_cache:bool=True,cache_position:CT7s4[31,34:A32.5],position_embeddings:(CT1s1x4x96[-1.1855769157409668,1.190237045288086:A0.7129333875218435],CT1s1x4x96[-1.1719439029693604,1.1902378797531128:A0.18296290554159592])))
>>> self_attn: Phi3Attention
> ((),dict(hidden_states:CT1s2x3x3072[-3.4361770153045654,4.301210880279541:A-0.00419270722959926],attention_mask:CT1s2x1x3x33[-3.4028234663852886e+38,-0.0:A-1.0311586261773601e+37],position_ids:CT7s1x3[30,32:A31.0],past_key_value:DynamicCache(key_cache=#2[CT1s2x32x33x96[-5.372235298156738,5.624973297119141:A0.001415606942792894],CT1s2x32x30x96[-4.347227573394775,4.55678129196167:A-0.00033970271848547825]], value_cache=#2[CT1s2x32x33x96[-4.442789077758789,4.48254919052124:A0.0016316771621383164],CT1s2x32x30x96[-4.140044689178467,4.500824451446533:A0.004017313765510237]]),output_attentions:bool=False,use_cache:bool=True,cache_position:CT7s3[30,32:A31.0],position_embeddings:(CT1s1x3x96[-1.1855769157409668,1.1902371644973755:A0.746652018013669],CT1s1x3x96[-1.1887905597686768,1.190193772315979:A0.1589894221542636])))
> ((),dict(hidden_states:CT1s3x4x3072[-3.896446943283081,3.8954379558563232:A0.007486624904945277],attention_mask:CT1s3x1x4x35[-3.4028234663852886e+38,-0.0:A-1.4583529141651237e+37],position_ids:CT7s1x4[31,34:A32.5],past_key_value:DynamicCache(key_cache=#2[CT1s3x32x35x96[-5.197488307952881,5.8184494972229:A0.00265941596204836],CT1s3x32x31x96[-4.275788307189941,5.158080101013184:A-0.00010541313345598764]], value_cache=#2[CT1s3x32x35x96[-4.953425407409668,4.6095075607299805:A0.0024469109531196453],CT1s3x32x31x96[-4.692654132843018,4.596354007720947:A0.0011176538469116694]]),output_attentions:bool=False,use_cache:bool=True,cache_position:CT7s4[31,34:A32.5],position_embeddings:(CT1s1x4x96[-1.1855769157409668,1.190237045288086:A0.7129333875218435],CT1s1x4x96[-1.1719439029693604,1.1902378797531128:A0.18296290554159592])))
>>> o_proj: Linear
> ((CT1s2x3x3072[-2.3118679523468018,2.1321918964385986:A0.004468887742732106],),{})
> ((CT1s3x4x3072[-2.5761353969573975,2.3879923820495605:A0.001925627289149927],),{})
< (CT1s2x3x3072[-1.669111967086792,1.6165475845336914:A0.002107051708561711],)
< (CT1s3x4x3072[-1.6189470291137695,1.8605895042419434:A0.0012024212607634076],)
<<<
>>> qkv_proj: Linear
> ((CT1s2x3x3072[-3.4361770153045654,4.301210880279541:A-0.00419270722959926],),{})
> ((CT1s3x4x3072[-3.896446943283081,3.8954379558563232:A0.007486624904945277],),{})
< (CT1s2x3x9216[-4.4062819480896,4.4155168533325195:A-0.002304110934694058],)
< (CT1s3x4x9216[-4.735391139984131,4.895002365112305:A0.00396526381984409],)
<<<
< (CT1s2x3x3072[-1.669111967086792,1.6165475845336914:A0.002107051708561711],None)
< (CT1s3x4x3072[-1.6189470291137695,1.8605895042419434:A0.0012024212607634076],None)
<<<
>>> mlp: Phi3MLP
> ((CT1s2x3x3072[-3.8036937713623047,4.038718223571777:A-0.0026494789857428223],),{})
> ((CT1s3x4x3072[-4.082477569580078,3.8404171466827393:A0.00814076230415934],),{})
>>> gate_up_proj: Linear
> ((CT1s2x3x3072[-3.8036937713623047,4.038718223571777:A-0.0026494789857428223],),{})
> ((CT1s3x4x3072[-4.082477569580078,3.8404171466827393:A0.00814076230415934],),{})
< (CT1s2x3x16384[-4.655969619750977,4.613260269165039:A0.00017156268975308345],)
< (CT1s3x4x16384[-4.791086196899414,5.44322395324707:A-0.004767606700279388],)
<<<
>>> down_proj: Linear
> ((CT1s2x3x8192[-10.599555969238281,9.737308502197266:A-0.0008998088124496303],),{})
> ((CT1s3x4x8192[-10.0259370803833,10.6680269241333:A-0.0002496852013821208],),{})
< (CT1s2x3x3072[-5.4764862060546875,5.631614685058594:A0.007689572318390169],)
< (CT1s3x4x3072[-5.459272861480713,6.003582954406738:A-0.013098056915787816],)
<<<
>>> activation_fn: SiLU
> ((CT1s2x3x8192[-4.563692092895508,4.613260269165039:A-0.003570237220931934],),{})
> ((CT1s3x4x8192[-4.7784037590026855,5.44322395324707:A-0.00511337001454167],),{})
< (CT1s2x3x8192[-0.27846455574035645,4.567948818206787:A0.24505810833321873],)
< (CT1s3x4x8192[-0.27846455574035645,5.419780731201172:A0.2426484803581621],)
<<<
< (CT1s2x3x3072[-5.4764862060546875,5.631614685058594:A0.007689572318390169],)
< (CT1s3x4x3072[-5.459272861480713,6.003582954406738:A-0.013098056915787816],)
<<<
>>> input_layernorm: Phi3RMSNorm
> ((CT1s2x3x3072[-4.941531181335449,6.185527801513672:A-0.005735762384069353],),{})
> ((CT1s3x4x3072[-5.470054626464844,5.551934719085693:A0.010753106478622007],),{})
< (CT1s2x3x3072[-3.4361770153045654,4.301210880279541:A-0.00419270722959926],)
< (CT1s3x4x3072[-3.896446943283081,3.8954379558563232:A0.007486624904945277],)
<<<
>>> post_attention_layernorm: Phi3RMSNorm
> ((CT1s2x3x3072[-5.518604278564453,5.699517250061035:A-0.003628710872362717],),{})
> ((CT1s3x4x3072[-5.853446006774902,5.695523738861084:A0.011955527800157344],),{})
< (CT1s2x3x3072[-3.8036937713623047,4.038718223571777:A-0.0026494789857428223],)
< (CT1s3x4x3072[-4.082477569580078,3.8404171466827393:A0.00814076230415934],)
<<<
>>> resid_attn_dropout: Dropout
> ((CT1s2x3x3072[-1.669111967086792,1.6165475845336914:A0.002107051708561711],),{})
> ((CT1s3x4x3072[-1.6189470291137695,1.8605895042419434:A0.0012024212607634076],),{})
< (CT1s2x3x3072[-1.669111967086792,1.6165475845336914:A0.002107051708561711],)
< (CT1s3x4x3072[-1.6189470291137695,1.8605895042419434:A0.0012024212607634076],)
<<<
>>> resid_mlp_dropout: Dropout
> ((CT1s2x3x3072[-5.4764862060546875,5.631614685058594:A0.007689572318390169],),{})
> ((CT1s3x4x3072[-5.459272861480713,6.003582954406738:A-0.013098056915787816],),{})
< (CT1s2x3x3072[-5.4764862060546875,5.631614685058594:A0.007689572318390169],)
< (CT1s3x4x3072[-5.459272861480713,6.003582954406738:A-0.013098056915787816],)
<<<
< (CT1s2x3x3072[-7.664382457733154,7.848972797393799:A0.004060861120605195],)
< (CT1s3x4x3072[-7.896722316741943,8.055901527404785:A-0.0011425288950401107],)
<<<
>>> norm: Phi3RMSNorm
> ((CT1s2x3x3072[-7.664382457733154,7.848972797393799:A0.004060861120605195],),{})
> ((CT1s3x4x3072[-7.896722316741943,8.055901527404785:A-0.0011425288950401107],),{})
< (CT1s2x3x3072[-3.9506890773773193,3.929338216781616:A0.00207373970610626],)
< (CT1s3x4x3072[-4.118087291717529,4.060365676879883:A-0.00048603209650227575],)
<<<
>>> rotary_emb: Phi3RotaryEmbedding
> ((CT1s2x3x3072[-0.0821705013513565,0.07243882864713669:A2.9103279443904203e-05],CT7s1x3[30,32:A31.0]),{})
> ((CT1s3x4x3072[-0.07996544241905212,0.07827455550432205:A1.0599782303485134e-05],CT7s1x4[31,34:A32.5]),{})
< (CT1s1x3x96[-1.1855769157409668,1.1902371644973755:A0.746652018013669],CT1s1x3x96[-1.1887905597686768,1.190193772315979:A0.1589894221542636])
< (CT1s1x4x96[-1.1855769157409668,1.190237045288086:A0.7129333875218435],CT1s1x4x96[-1.1719439029693604,1.1902378797531128:A0.18296290554159592])
<<<
< (dict(last_hidden_state:CT1s2x3x3072[-3.9506890773773193,3.929338216781616:A0.00207373970610626],past_key_values:DynamicCache(key_cache=#2[CT1s2x32x33x96[-5.372235298156738,5.624973297119141:A0.001415606942792894],CT1s2x32x33x96[-5.70737361907959,5.014967918395996:A-0.0008282634507489216]], value_cache=#2[CT1s2x32x33x96[-4.442789077758789,4.48254919052124:A0.0016316771621383164],CT1s2x32x33x96[-4.4062819480896,4.500824451446533:A0.003503265759676259]])),)
< (dict(last_hidden_state:CT1s3x4x3072[-4.118087291717529,4.060365676879883:A-0.00048603209650227575],past_key_values:DynamicCache(key_cache=#2[CT1s3x32x35x96[-5.197488307952881,5.8184494972229:A0.00265941596204836],CT1s3x32x35x96[-5.370776176452637,5.158080101013184:A8.010243163269966e-05]], value_cache=#2[CT1s3x32x35x96[-4.953425407409668,4.6095075607299805:A0.0024469109531196453],CT1s3x32x35x96[-4.735391139984131,4.7360310554504395:A0.001602801948693938]])),)
<<<
>>> lm_head: Linear
> ((CT1s2x3x3072[-3.9506890773773193,3.929338216781616:A0.00207373970610626],),{})
> ((CT1s3x4x3072[-4.118087291717529,4.060365676879883:A-0.00048603209650227575],),{})
< (CT1s2x3x32064[-5.118677616119385,4.6263628005981445:A0.000804852578185648],)
< (CT1s3x4x32064[-5.093557357788086,5.201630115509033:A0.0005855126074918249],)
<<<
< (dict(logits:CT1s2x3x32064[-5.118677616119385,4.6263628005981445:A0.000804852578185648],past_key_values:DynamicCache(key_cache=#2[CT1s2x32x33x96[-5.372235298156738,5.624973297119141:A0.001415606942792894],CT1s2x32x33x96[-5.70737361907959,5.014967918395996:A-0.0008282634507489216]], value_cache=#2[CT1s2x32x33x96[-4.442789077758789,4.48254919052124:A0.0016316771621383164],CT1s2x32x33x96[-4.4062819480896,4.500824451446533:A0.003503265759676259]])),)
< (dict(logits:CT1s3x4x32064[-5.093557357788086,5.201630115509033:A0.0005855126074918249],past_key_values:DynamicCache(key_cache=#2[CT1s3x32x35x96[-5.197488307952881,5.8184494972229:A0.00265941596204836],CT1s3x32x35x96[-5.370776176452637,5.158080101013184:A8.010243163269966e-05]], value_cache=#2[CT1s3x32x35x96[-4.953425407409668,4.6095075607299805:A0.0024469109531196453],CT1s3x32x35x96[-4.735391139984131,4.7360310554504395:A0.001602801948693938]])),)
<<<
[_untrace_forward_execution] M:__main__-Phi3ForCausalLM
[_untrace_forward_execution] .. M:model-Phi3Model
[_untrace_forward_execution] .... M:embed_tokens-Embedding
[_untrace_forward_execution] .... M:layers[0]-Phi3DecoderLayer
[_untrace_forward_execution] ...... M:self_attn-Phi3Attention
[_untrace_forward_execution] ........ M:o_proj-Linear
[_untrace_forward_execution] ........ M:qkv_proj-Linear
[_untrace_forward_execution] ...... M:mlp-Phi3MLP
[_untrace_forward_execution] ........ M:gate_up_proj-Linear
[_untrace_forward_execution] ........ M:down_proj-Linear
[_untrace_forward_execution] ........ M:activation_fn-SiLU
[_untrace_forward_execution] ...... M:input_layernorm-Phi3RMSNorm
[_untrace_forward_execution] ...... M:post_attention_layernorm-Phi3RMSNorm
[_untrace_forward_execution] ...... M:resid_attn_dropout-Dropout
[_untrace_forward_execution] ...... M:resid_mlp_dropout-Dropout
[_untrace_forward_execution] .... M:layers[1]-Phi3DecoderLayer
[_untrace_forward_execution] ...... M:self_attn-Phi3Attention
[_untrace_forward_execution] ........ M:o_proj-Linear
[_untrace_forward_execution] ........ M:qkv_proj-Linear
[_untrace_forward_execution] ...... M:mlp-Phi3MLP
[_untrace_forward_execution] ........ M:gate_up_proj-Linear
[_untrace_forward_execution] ........ M:down_proj-Linear
[_untrace_forward_execution] ........ M:activation_fn-SiLU
[_untrace_forward_execution] ...... M:input_layernorm-Phi3RMSNorm
[_untrace_forward_execution] ...... M:post_attention_layernorm-Phi3RMSNorm
[_untrace_forward_execution] ...... M:resid_attn_dropout-Dropout
[_untrace_forward_execution] ...... M:resid_mlp_dropout-Dropout
[_untrace_forward_execution] .... M:norm-Phi3RMSNorm
[_untrace_forward_execution] .... M:rotary_emb-Phi3RotaryEmbedding
[_untrace_forward_execution] .. M:lm_head-Linear
Now we keep in memory every input/output for the submodules, we can guess the dynamic shapes for every of them. The final ones:
dynamic_shapes = diag.guess_dynamic_shapes()
print("The dynamic shapes are:")
pprint.pprint(dynamic_shapes)
The dynamic shapes are:
((),
{'attention_mask': {0: <_DimHint.DYNAMIC: 3>, 1: <_DimHint.DYNAMIC: 3>},
'input_ids': {0: <_DimHint.DYNAMIC: 3>, 1: <_DimHint.DYNAMIC: 3>},
'past_key_values': [[{0: <_DimHint.DYNAMIC: 3>, 2: <_DimHint.DYNAMIC: 3>},
{0: <_DimHint.DYNAMIC: 3>, 2: <_DimHint.DYNAMIC: 3>}],
[{0: <_DimHint.DYNAMIC: 3>, 2: <_DimHint.DYNAMIC: 3>},
{0: <_DimHint.DYNAMIC: 3>, 2: <_DimHint.DYNAMIC: 3>}]]})
And all the dynamic shapes all along the traced submodules.
print(
diag.pretty_text(
with_dynamic_shape=True,
with_shape=False,
with_min_max=False,
with_device=False,
with_inputs=False,
).replace("<_DimHint.DYNAMIC: 3>", "DYN")
)
>>> __main__: Phi3ForCausalLM
DS=((), {'attention_mask': {0: DYN, 1: DYN}, 'input_ids': {0: DYN, 1: DYN}, 'past_key_values': [[{0: DYN, 2: DYN}, {0: DYN, 2: DYN}], [{0: DYN, 2: DYN}, {0: DYN, 2: DYN}]]})
>>> model: Phi3Model
DS=((), {'attention_mask': {0: DYN, 1: DYN}, 'cache_position': None, 'input_ids': {0: DYN, 1: DYN}, 'inputs_embeds': None, 'output_attentions': None, 'output_hidden_states': None, 'past_key_values': [[{0: DYN, 2: DYN}, {0: DYN, 2: DYN}], [{0: DYN, 2: DYN}, {0: DYN, 2: DYN}]], 'position_ids': None, 'return_dict': None, 'use_cache': None})
>>> embed_tokens: Embedding: DS=(({0: DYN, 1: DYN},), {}) <<<
>>> layers[0]: Phi3DecoderLayer
DS=(({0: DYN, 1: DYN},), {'attention_mask': {0: DYN, 2: DYN, 3: DYN}, 'cache_position': {0: DYN}, 'output_attentions': None, 'past_key_value': [[{0: DYN, 2: DYN}, {0: DYN, 2: DYN}], [{0: DYN, 2: DYN}, {0: DYN, 2: DYN}]], 'position_embeddings': ({1: DYN}, {1: DYN}), 'position_ids': {1: DYN}, 'use_cache': None})
>>> self_attn: Phi3Attention
DS=((), {'attention_mask': {0: DYN, 2: DYN, 3: DYN}, 'cache_position': {0: DYN}, 'hidden_states': {0: DYN, 1: DYN}, 'output_attentions': None, 'past_key_value': [[{0: DYN, 2: DYN}, {0: DYN, 2: DYN}], [{0: DYN, 2: DYN}, {0: DYN, 2: DYN}]], 'position_embeddings': ({1: DYN}, {1: DYN}), 'position_ids': {1: DYN}, 'use_cache': None})
>>> o_proj: Linear: DS=(({0: DYN, 1: DYN},), {}) <<<
>>> qkv_proj: Linear: DS=(({0: DYN, 1: DYN},), {}) <<<
<<<
>>> mlp: Phi3MLP
DS=(({0: DYN, 1: DYN},), {})
>>> gate_up_proj: Linear: DS=(({0: DYN, 1: DYN},), {}) <<<
>>> down_proj: Linear: DS=(({0: DYN, 1: DYN},), {}) <<<
>>> activation_fn: SiLU: DS=(({0: DYN, 1: DYN},), {}) <<<
<<<
>>> input_layernorm: Phi3RMSNorm: DS=(({0: DYN, 1: DYN},), {}) <<<
>>> post_attention_layernorm: Phi3RMSNorm: DS=(({0: DYN, 1: DYN},), {}) <<<
>>> resid_attn_dropout: Dropout: DS=(({0: DYN, 1: DYN},), {}) <<<
>>> resid_mlp_dropout: Dropout: DS=(({0: DYN, 1: DYN},), {}) <<<
<<<
>>> layers[1]: Phi3DecoderLayer
DS=(({0: DYN, 1: DYN},), {'attention_mask': {0: DYN, 2: DYN, 3: DYN}, 'cache_position': {0: DYN}, 'output_attentions': None, 'past_key_value': [[{0: DYN, 2: DYN}, {0: DYN, 2: DYN}], [{0: DYN, 2: DYN}, {0: DYN, 2: DYN}]], 'position_embeddings': ({1: DYN}, {1: DYN}), 'position_ids': {1: DYN}, 'use_cache': None})
>>> self_attn: Phi3Attention
DS=((), {'attention_mask': {0: DYN, 2: DYN, 3: DYN}, 'cache_position': {0: DYN}, 'hidden_states': {0: DYN, 1: DYN}, 'output_attentions': None, 'past_key_value': [[{0: DYN, 2: DYN}, {0: DYN, 2: DYN}], [{0: DYN, 2: DYN}, {0: DYN, 2: DYN}]], 'position_embeddings': ({1: DYN}, {1: DYN}), 'position_ids': {1: DYN}, 'use_cache': None})
>>> o_proj: Linear: DS=(({0: DYN, 1: DYN},), {}) <<<
>>> qkv_proj: Linear: DS=(({0: DYN, 1: DYN},), {}) <<<
<<<
>>> mlp: Phi3MLP
DS=(({0: DYN, 1: DYN},), {})
>>> gate_up_proj: Linear: DS=(({0: DYN, 1: DYN},), {}) <<<
>>> down_proj: Linear: DS=(({0: DYN, 1: DYN},), {}) <<<
>>> activation_fn: SiLU: DS=(({0: DYN, 1: DYN},), {}) <<<
<<<
>>> input_layernorm: Phi3RMSNorm: DS=(({0: DYN, 1: DYN},), {}) <<<
>>> post_attention_layernorm: Phi3RMSNorm: DS=(({0: DYN, 1: DYN},), {}) <<<
>>> resid_attn_dropout: Dropout: DS=(({0: DYN, 1: DYN},), {}) <<<
>>> resid_mlp_dropout: Dropout: DS=(({0: DYN, 1: DYN},), {}) <<<
<<<
>>> norm: Phi3RMSNorm: DS=(({0: DYN, 1: DYN},), {}) <<<
>>> rotary_emb: Phi3RotaryEmbedding: DS=(({0: DYN, 1: DYN}, {1: DYN}), {}) <<<
<<<
>>> lm_head: Linear: DS=(({0: DYN, 1: DYN},), {}) <<<
<<<
Evaluate the export¶
In many cases, the export (to torch.fx.Graph
, to ONNX)
does not work on the first try. We need a way to understand
how much the model can be exported. It can be used to evaluate
the how much code needs to be rewritten or patched to be exportable.
The verbosity can be increase to show dynamic shapes, results
of the discrepancies.
Let’s display the module and its submodule first.
print(
diag.pretty_text(
with_dynamic_shape=False,
with_shape=False,
with_min_max=False,
with_device=False,
with_inputs=False,
)
)
>>> __main__: Phi3ForCausalLM
>>> model: Phi3Model
>>> embed_tokens: Embedding <<<
>>> layers[0]: Phi3DecoderLayer
>>> self_attn: Phi3Attention
>>> o_proj: Linear <<<
>>> qkv_proj: Linear <<<
<<<
>>> mlp: Phi3MLP
>>> gate_up_proj: Linear <<<
>>> down_proj: Linear <<<
>>> activation_fn: SiLU <<<
<<<
>>> input_layernorm: Phi3RMSNorm <<<
>>> post_attention_layernorm: Phi3RMSNorm <<<
>>> resid_attn_dropout: Dropout <<<
>>> resid_mlp_dropout: Dropout <<<
<<<
>>> layers[1]: Phi3DecoderLayer
>>> self_attn: Phi3Attention
>>> o_proj: Linear <<<
>>> qkv_proj: Linear <<<
<<<
>>> mlp: Phi3MLP
>>> gate_up_proj: Linear <<<
>>> down_proj: Linear <<<
>>> activation_fn: SiLU <<<
<<<
>>> input_layernorm: Phi3RMSNorm <<<
>>> post_attention_layernorm: Phi3RMSNorm <<<
>>> resid_attn_dropout: Dropout <<<
>>> resid_mlp_dropout: Dropout <<<
<<<
>>> norm: Phi3RMSNorm <<<
>>> rotary_emb: Phi3RotaryEmbedding <<<
<<<
>>> lm_head: Linear <<<
<<<
The we try to export to see the submodule failing the whole model. We can pickle the failing model and restore it to speedup the refactoring to make it work.
print("----------------------")
ep = diag.try_export(
exporter="fx",
use_dynamic_shapes=True,
exporter_kwargs=dict(strict=False),
verbose=1,
)
----------------------
def forward(self, arg0_1: "f32[32064, 3072]", arg1_1: "f32[3072, 3072]", arg2_1: "f32[9216, 3072]", arg3_1: "f32[16384, 3072]", arg4_1: "f32[3072, 8192]", arg5_1: "f32[3072]", arg6_1: "f32[3072]", arg7_1: "f32[3072, 3072]", arg8_1: "f32[9216, 3072]", arg9_1: "f32[16384, 3072]", arg10_1: "f32[3072, 8192]", arg11_1: "f32[3072]", arg12_1: "f32[3072]", arg13_1: "f32[3072]", arg14_1: "f32[32064, 3072]", arg15_1: "f32[48]", arg16_1: "i64[s0, s1]", arg17_1: "i64[s0, s3]", arg18_1: "f32[2, 32, s5, 96]", arg19_1: "f32[s6, 32, s7, 96]", arg20_1: "f32[s8, 32, s9, 96]", arg21_1: "f32[s10, 32, s11, 96]"):
# File: /home/xadupre/vv/this312/lib/python3.12/site-packages/torch/nn/modules/sparse.py:190 in forward, code: return F.embedding(
embedding: "f32[s0, s1, 3072]" = torch.ops.aten.embedding.default(arg0_1, arg16_1, 32000); arg0_1 = embedding = None
# File: /home/xadupre/vv/this312/lib/python3.12/site-packages/transformers/models/phi3/modeling_phi3.py:598 in forward, code: past_seen_tokens, past_seen_tokens + inputs_embeds.shape[1], device=inputs_embeds.device
sym_size_int: "Sym(s5)" = torch.ops.aten.sym_size.int(arg18_1, 2); arg18_1 = None
sym_size_int_1: "Sym(s1)" = torch.ops.aten.sym_size.int(arg16_1, 1)
add: "Sym(s1 + s5)" = sym_size_int + sym_size_int_1
# File: /home/xadupre/vv/this312/lib/python3.12/site-packages/transformers/models/phi3/modeling_phi3.py:597 in forward, code: cache_position = torch.arange(
arange: "i64[s1]" = torch.ops.aten.arange.start(sym_size_int, add, device = device(type='cpu'), pin_memory = False); add = None
# File: /home/xadupre/vv/this312/lib/python3.12/site-packages/transformers/models/phi3/modeling_phi3.py:602 in forward, code: position_ids = cache_position.unsqueeze(0)
unsqueeze: "i64[1, s1]" = torch.ops.aten.unsqueeze.default(arange, 0)
# File: /home/xadupre/vv/this312/lib/python3.12/site-packages/transformers/models/phi3/modeling_phi3.py:604 in forward, code: causal_mask = self._update_causal_mask(
add_1: "Sym(s1 + s5)" = sym_size_int_1 + sym_size_int; sym_size_int = None
lt: "Sym(s1 + s5 < 262144)" = add_1 < 262144; add_1 = lt = None
sym_size_int_2: "Sym(s3)" = torch.ops.aten.sym_size.int(arg17_1, 1)
full: "f32[s1, s3]" = torch.ops.aten.full.default([sym_size_int_1, sym_size_int_2], -3.4028234663852886e+38, dtype = torch.float32, device = device(type='cpu'), pin_memory = False)
arange_1: "i64[s3]" = torch.ops.aten.arange.default(sym_size_int_2, device = device(type='cpu'), pin_memory = False)
reshape: "i64[s1, 1]" = torch.ops.aten.reshape.default(arange, [-1, 1])
gt: "b8[s1, s3]" = torch.ops.aten.gt.Tensor(arange_1, reshape); arange_1 = reshape = None
arange_2: "i64[s3]" = torch.ops.aten.arange.default(sym_size_int_2, device = device(type='cpu'), pin_memory = False)
reshape_1: "i64[s1, 1]" = torch.ops.aten.reshape.default(arange, [-1, 1]); arange = None
sub: "i64[s1, 1]" = torch.ops.aten.sub.Tensor(reshape_1, 262144); reshape_1 = None
le: "b8[s1, s3]" = torch.ops.aten.le.Tensor(arange_2, sub); arange_2 = sub = None
bitwise_or_: "b8[s1, s3]" = torch.ops.aten.bitwise_or_.Tensor(gt, le); gt = le = None
mul_: "f32[s1, s3]" = torch.ops.aten.mul_.Tensor(full, bitwise_or_); full = bitwise_or_ = None
unsqueeze_1: "f32[1, s1, s3]" = torch.ops.aten.unsqueeze.default(mul_, 0); mul_ = None
unsqueeze_2: "f32[1, 1, s1, s3]" = torch.ops.aten.unsqueeze.default(unsqueeze_1, 1); unsqueeze_1 = None
eq: "Sym(Eq(s1, 9223372036854775807))" = sym_size_int_1 == 9223372036854775807; sym_size_int_1 = eq = None
slice_1: "f32[1, 1, s1, s3]" = torch.ops.aten.slice.Tensor(unsqueeze_2, 2, 0, 9223372036854775807); unsqueeze_2 = None
eq_1: "Sym(Eq(s3, 9223372036854775807))" = sym_size_int_2 == 9223372036854775807; eq_1 = None
slice_2: "f32[1, 1, s1, s3]" = torch.ops.aten.slice.Tensor(slice_1, 3, 0, 9223372036854775807)
sym_size_int_3: "Sym(s0)" = torch.ops.aten.sym_size.int(arg16_1, 0); arg16_1 = None
expand: "f32[s0, 1, s1, s3]" = torch.ops.aten.expand.default(slice_2, [sym_size_int_3, 1, -1, -1])
clone: "f32[s0, 1, s1, s3]" = torch.ops.aten.clone.default(expand); expand = None
gt_1: "Sym(False)" = sym_size_int_2 > sym_size_int_2; gt_1 = None
eq_2: "Sym(Eq(s0, 9223372036854775807))" = sym_size_int_3 == 9223372036854775807; eq_2 = None
slice_3: "f32[s0, 1, s1, s3]" = torch.ops.aten.slice.Tensor(clone, 0, 0, 9223372036854775807)
slice_4: "f32[s0, 1, s1, s3]" = torch.ops.aten.slice.Tensor(slice_3, 1, 0, 9223372036854775807)
sym_size_int_4: "Sym(s1)" = torch.ops.aten.sym_size.int(slice_1, 2); slice_1 = None
eq_3: "Sym(Eq(s1, 9223372036854775807))" = sym_size_int_4 == 9223372036854775807; eq_3 = None
slice_5: "f32[s0, 1, s1, s3]" = torch.ops.aten.slice.Tensor(slice_4, 2, 0, 9223372036854775807); slice_4 = None
sym_size_int_5: "Sym(s3)" = torch.ops.aten.sym_size.int(slice_2, 3); slice_2 = None
eq_4: "Sym(True)" = sym_size_int_5 == sym_size_int_2; eq_4 = None
sym_size_int_6: "Sym(s0)" = torch.ops.aten.sym_size.int(arg17_1, 0)
eq_5: "Sym(Eq(s0, 9223372036854775807))" = sym_size_int_6 == 9223372036854775807; sym_size_int_6 = eq_5 = None
slice_6: "i64[s0, s3]" = torch.ops.aten.slice.Tensor(arg17_1, 0, 0, 9223372036854775807); arg17_1 = None
unsqueeze_3: "i64[s0, 1, s3]" = torch.ops.aten.unsqueeze.default(slice_6, 1); slice_6 = None
unsqueeze_4: "i64[s0, 1, 1, s3]" = torch.ops.aten.unsqueeze.default(unsqueeze_3, 2); unsqueeze_3 = None
eq_6: "Sym(Eq(s3, 9223372036854775807))" = sym_size_int_2 == 9223372036854775807; eq_6 = None
slice_7: "i64[s0, 1, 1, s3]" = torch.ops.aten.slice.Tensor(unsqueeze_4, 3, 0, 9223372036854775807); unsqueeze_4 = None
add_2: "f32[s0, 1, s1, s3]" = torch.ops.aten.add.Tensor(slice_5, slice_7); slice_7 = None
eq_7: "b8[s0, 1, s1, s3]" = torch.ops.aten.eq.Scalar(add_2, 0); add_2 = None
eq_8: "Sym(Eq(s0, 9223372036854775807))" = sym_size_int_3 == 9223372036854775807; eq_8 = None
slice_8: "f32[s0, 1, s1, s3]" = torch.ops.aten.slice.Tensor(clone, 0, 0, 9223372036854775807)
slice_9: "f32[s0, 1, s1, s3]" = torch.ops.aten.slice.Tensor(slice_8, 1, 0, 9223372036854775807); slice_8 = None
eq_9: "Sym(Eq(s1, 9223372036854775807))" = sym_size_int_4 == 9223372036854775807; eq_9 = None
slice_10: "f32[s0, 1, s1, s3]" = torch.ops.aten.slice.Tensor(slice_9, 2, 0, 9223372036854775807); slice_9 = None
eq_10: "Sym(True)" = sym_size_int_5 == sym_size_int_2; eq_10 = None
masked_fill: "f32[s0, 1, s1, s3]" = torch.ops.aten.masked_fill.Scalar(slice_10, eq_7, -3.4028234663852886e+38); slice_10 = eq_7 = None
eq_11: "Sym(Eq(s0, 9223372036854775807))" = sym_size_int_3 == 9223372036854775807; sym_size_int_3 = eq_11 = None
slice_11: "f32[s0, 1, s1, s3]" = torch.ops.aten.slice.Tensor(clone, 0, 0, 9223372036854775807); clone = None
slice_12: "f32[s0, 1, s1, s3]" = torch.ops.aten.slice.Tensor(slice_11, 1, 0, 9223372036854775807)
eq_12: "Sym(Eq(s1, 9223372036854775807))" = sym_size_int_4 == 9223372036854775807; sym_size_int_4 = eq_12 = None
slice_13: "f32[s0, 1, s1, s3]" = torch.ops.aten.slice.Tensor(slice_12, 2, 0, 9223372036854775807); slice_12 = None
eq_13: "Sym(True)" = sym_size_int_5 == sym_size_int_2; sym_size_int_2 = eq_13 = None
sym_size_int_7: "Sym(s0)" = torch.ops.aten.sym_size.int(slice_11, 0); slice_11 = None
sym_size_int_8: "Sym(s0)" = torch.ops.aten.sym_size.int(slice_3, 0); slice_3 = None
eq_14: "Sym(True)" = sym_size_int_7 == sym_size_int_8; sym_size_int_7 = sym_size_int_8 = eq_14 = None
sym_size_int_9: "Sym(s1)" = torch.ops.aten.sym_size.int(slice_13, 2)
sym_size_int_10: "Sym(s1)" = torch.ops.aten.sym_size.int(slice_5, 2); slice_5 = None
eq_15: "Sym(True)" = sym_size_int_9 == sym_size_int_10; sym_size_int_9 = sym_size_int_10 = eq_15 = None
eq_16: "Sym(True)" = sym_size_int_5 == sym_size_int_5; sym_size_int_5 = eq_16 = None
copy_: "f32[s0, 1, s1, s3]" = torch.ops.aten.copy_.default(slice_13, masked_fill); slice_13 = masked_fill = copy_ = None
# File: /home/xadupre/vv/this312/lib/python3.12/site-packages/transformers/models/phi3/modeling_phi3.py:611 in forward, code: position_embeddings = self.rotary_emb(hidden_states, position_ids)
_set_grad_enabled = torch._C._set_grad_enabled(False); _set_grad_enabled = None
# File: /home/xadupre/vv/this312/lib/python3.12/site-packages/transformers/models/phi3/modeling_phi3.py:362 in forward, code: self._longrope_frequency_update(position_ids, device=x.device)
max_1: "i64[]" = torch.ops.aten.max.default(unsqueeze); unsqueeze = None
add_3: "i64[]" = torch.ops.aten.add.Tensor(max_1, 1); max_1 = None
gt_2: "b8[]" = torch.ops.aten.gt.Scalar(add_3, 4096); add_3 = None
ne: "b8[]" = torch.ops.aten.ne.Scalar(gt_2, 0); gt_2 = None
item: "Sym(Eq(u0, 1))" = torch.ops.aten.item.default(ne); ne = item = None
# File: /home/xadupre/vv/this312/lib/python3.12/site-packages/transformers/models/phi3/modeling_phi3.py:611 in forward, code: position_embeddings = self.rotary_emb(hidden_states, position_ids)
_set_grad_enabled_1 = torch._C._set_grad_enabled(True); _set_grad_enabled_1 = None
/home/xadupre/vv/this312/lib/python3.12/site-packages/torch/backends/mkldnn/__init__.py:78: UserWarning: TF32 acceleration on top of oneDNN is available for Intel GPUs. The current Torch version does not have Intel GPU Support. (Triggered internally at /pytorch/aten/src/ATen/Context.cpp:148.)
torch._C._set_onednn_allow_tf32(_allow_tf32)
[try_export-FX] M:__main__-Phi3ForCausalLM --- FAIL, step=EXPORT, reason=Could not guard on data-dependent expression Eq(u0, 1) (unhinted: Eq(u0, 1)). (Size-like symbols: none)
def forward(self, arg0_1: "f32[32064, 3072]", arg1_1: "f32[3072, 3072]", arg2_1: "f32[9216, 3072]", arg3_1: "f32[16384, 3072]", arg4_1: "f32[3072, 8192]", arg5_1: "f32[3072]", arg6_1: "f32[3072]", arg7_1: "f32[3072, 3072]", arg8_1: "f32[9216, 3072]", arg9_1: "f32[16384, 3072]", arg10_1: "f32[3072, 8192]", arg11_1: "f32[3072]", arg12_1: "f32[3072]", arg13_1: "f32[3072]", arg14_1: "f32[48]", arg15_1: "i64[s0, s1]", arg16_1: "i64[s0, s3]", arg17_1, arg18_1: "f32[2, 32, s5, 96]", arg19_1: "f32[s6, 32, s7, 96]", arg20_1: "f32[s8, 32, s9, 96]", arg21_1: "f32[s10, 32, s11, 96]", arg22_1, arg23_1, arg24_1, arg25_1, arg26_1, arg27_1):
# File: /home/xadupre/vv/this312/lib/python3.12/site-packages/torch/nn/modules/sparse.py:190 in forward, code: return F.embedding(
embedding: "f32[s0, s1, 3072]" = torch.ops.aten.embedding.default(arg0_1, arg15_1, 32000); arg0_1 = embedding = None
# File: /home/xadupre/vv/this312/lib/python3.12/site-packages/transformers/models/phi3/modeling_phi3.py:598 in forward, code: past_seen_tokens, past_seen_tokens + inputs_embeds.shape[1], device=inputs_embeds.device
sym_size_int: "Sym(s5)" = torch.ops.aten.sym_size.int(arg18_1, 2); arg18_1 = None
sym_size_int_1: "Sym(s1)" = torch.ops.aten.sym_size.int(arg15_1, 1)
add: "Sym(s1 + s5)" = sym_size_int + sym_size_int_1
# File: /home/xadupre/vv/this312/lib/python3.12/site-packages/transformers/models/phi3/modeling_phi3.py:597 in forward, code: cache_position = torch.arange(
arange: "i64[s1]" = torch.ops.aten.arange.start(sym_size_int, add, device = device(type='cpu'), pin_memory = False); add = None
# File: /home/xadupre/vv/this312/lib/python3.12/site-packages/transformers/models/phi3/modeling_phi3.py:602 in forward, code: position_ids = cache_position.unsqueeze(0)
unsqueeze: "i64[1, s1]" = torch.ops.aten.unsqueeze.default(arange, 0)
# File: /home/xadupre/vv/this312/lib/python3.12/site-packages/transformers/models/phi3/modeling_phi3.py:604 in forward, code: causal_mask = self._update_causal_mask(
add_1: "Sym(s1 + s5)" = sym_size_int_1 + sym_size_int; sym_size_int = None
lt: "Sym(s1 + s5 < 262144)" = add_1 < 262144; add_1 = lt = None
sym_size_int_2: "Sym(s3)" = torch.ops.aten.sym_size.int(arg16_1, 1)
full: "f32[s1, s3]" = torch.ops.aten.full.default([sym_size_int_1, sym_size_int_2], -3.4028234663852886e+38, dtype = torch.float32, device = device(type='cpu'), pin_memory = False)
arange_1: "i64[s3]" = torch.ops.aten.arange.default(sym_size_int_2, device = device(type='cpu'), pin_memory = False)
reshape: "i64[s1, 1]" = torch.ops.aten.reshape.default(arange, [-1, 1])
gt: "b8[s1, s3]" = torch.ops.aten.gt.Tensor(arange_1, reshape); arange_1 = reshape = None
arange_2: "i64[s3]" = torch.ops.aten.arange.default(sym_size_int_2, device = device(type='cpu'), pin_memory = False)
reshape_1: "i64[s1, 1]" = torch.ops.aten.reshape.default(arange, [-1, 1]); arange = None
sub: "i64[s1, 1]" = torch.ops.aten.sub.Tensor(reshape_1, 262144); reshape_1 = None
le: "b8[s1, s3]" = torch.ops.aten.le.Tensor(arange_2, sub); arange_2 = sub = None
bitwise_or_: "b8[s1, s3]" = torch.ops.aten.bitwise_or_.Tensor(gt, le); gt = le = None
mul_: "f32[s1, s3]" = torch.ops.aten.mul_.Tensor(full, bitwise_or_); full = bitwise_or_ = None
unsqueeze_1: "f32[1, s1, s3]" = torch.ops.aten.unsqueeze.default(mul_, 0); mul_ = None
unsqueeze_2: "f32[1, 1, s1, s3]" = torch.ops.aten.unsqueeze.default(unsqueeze_1, 1); unsqueeze_1 = None
eq: "Sym(Eq(s1, 9223372036854775807))" = sym_size_int_1 == 9223372036854775807; sym_size_int_1 = eq = None
slice_1: "f32[1, 1, s1, s3]" = torch.ops.aten.slice.Tensor(unsqueeze_2, 2, 0, 9223372036854775807); unsqueeze_2 = None
eq_1: "Sym(Eq(s3, 9223372036854775807))" = sym_size_int_2 == 9223372036854775807; eq_1 = None
slice_2: "f32[1, 1, s1, s3]" = torch.ops.aten.slice.Tensor(slice_1, 3, 0, 9223372036854775807)
sym_size_int_3: "Sym(s0)" = torch.ops.aten.sym_size.int(arg15_1, 0); arg15_1 = None
expand: "f32[s0, 1, s1, s3]" = torch.ops.aten.expand.default(slice_2, [sym_size_int_3, 1, -1, -1])
clone: "f32[s0, 1, s1, s3]" = torch.ops.aten.clone.default(expand); expand = None
gt_1: "Sym(False)" = sym_size_int_2 > sym_size_int_2; gt_1 = None
eq_2: "Sym(Eq(s0, 9223372036854775807))" = sym_size_int_3 == 9223372036854775807; eq_2 = None
slice_3: "f32[s0, 1, s1, s3]" = torch.ops.aten.slice.Tensor(clone, 0, 0, 9223372036854775807)
slice_4: "f32[s0, 1, s1, s3]" = torch.ops.aten.slice.Tensor(slice_3, 1, 0, 9223372036854775807)
sym_size_int_4: "Sym(s1)" = torch.ops.aten.sym_size.int(slice_1, 2); slice_1 = None
eq_3: "Sym(Eq(s1, 9223372036854775807))" = sym_size_int_4 == 9223372036854775807; eq_3 = None
slice_5: "f32[s0, 1, s1, s3]" = torch.ops.aten.slice.Tensor(slice_4, 2, 0, 9223372036854775807); slice_4 = None
sym_size_int_5: "Sym(s3)" = torch.ops.aten.sym_size.int(slice_2, 3); slice_2 = None
eq_4: "Sym(True)" = sym_size_int_5 == sym_size_int_2; eq_4 = None
sym_size_int_6: "Sym(s0)" = torch.ops.aten.sym_size.int(arg16_1, 0)
eq_5: "Sym(Eq(s0, 9223372036854775807))" = sym_size_int_6 == 9223372036854775807; sym_size_int_6 = eq_5 = None
slice_6: "i64[s0, s3]" = torch.ops.aten.slice.Tensor(arg16_1, 0, 0, 9223372036854775807); arg16_1 = None
unsqueeze_3: "i64[s0, 1, s3]" = torch.ops.aten.unsqueeze.default(slice_6, 1); slice_6 = None
unsqueeze_4: "i64[s0, 1, 1, s3]" = torch.ops.aten.unsqueeze.default(unsqueeze_3, 2); unsqueeze_3 = None
eq_6: "Sym(Eq(s3, 9223372036854775807))" = sym_size_int_2 == 9223372036854775807; eq_6 = None
slice_7: "i64[s0, 1, 1, s3]" = torch.ops.aten.slice.Tensor(unsqueeze_4, 3, 0, 9223372036854775807); unsqueeze_4 = None
add_2: "f32[s0, 1, s1, s3]" = torch.ops.aten.add.Tensor(slice_5, slice_7); slice_7 = None
eq_7: "b8[s0, 1, s1, s3]" = torch.ops.aten.eq.Scalar(add_2, 0); add_2 = None
eq_8: "Sym(Eq(s0, 9223372036854775807))" = sym_size_int_3 == 9223372036854775807; eq_8 = None
slice_8: "f32[s0, 1, s1, s3]" = torch.ops.aten.slice.Tensor(clone, 0, 0, 9223372036854775807)
slice_9: "f32[s0, 1, s1, s3]" = torch.ops.aten.slice.Tensor(slice_8, 1, 0, 9223372036854775807); slice_8 = None
eq_9: "Sym(Eq(s1, 9223372036854775807))" = sym_size_int_4 == 9223372036854775807; eq_9 = None
slice_10: "f32[s0, 1, s1, s3]" = torch.ops.aten.slice.Tensor(slice_9, 2, 0, 9223372036854775807); slice_9 = None
eq_10: "Sym(True)" = sym_size_int_5 == sym_size_int_2; eq_10 = None
masked_fill: "f32[s0, 1, s1, s3]" = torch.ops.aten.masked_fill.Scalar(slice_10, eq_7, -3.4028234663852886e+38); slice_10 = eq_7 = None
eq_11: "Sym(Eq(s0, 9223372036854775807))" = sym_size_int_3 == 9223372036854775807; sym_size_int_3 = eq_11 = None
slice_11: "f32[s0, 1, s1, s3]" = torch.ops.aten.slice.Tensor(clone, 0, 0, 9223372036854775807); clone = None
slice_12: "f32[s0, 1, s1, s3]" = torch.ops.aten.slice.Tensor(slice_11, 1, 0, 9223372036854775807)
eq_12: "Sym(Eq(s1, 9223372036854775807))" = sym_size_int_4 == 9223372036854775807; sym_size_int_4 = eq_12 = None
slice_13: "f32[s0, 1, s1, s3]" = torch.ops.aten.slice.Tensor(slice_12, 2, 0, 9223372036854775807); slice_12 = None
eq_13: "Sym(True)" = sym_size_int_5 == sym_size_int_2; sym_size_int_2 = eq_13 = None
sym_size_int_7: "Sym(s0)" = torch.ops.aten.sym_size.int(slice_11, 0); slice_11 = None
sym_size_int_8: "Sym(s0)" = torch.ops.aten.sym_size.int(slice_3, 0); slice_3 = None
eq_14: "Sym(True)" = sym_size_int_7 == sym_size_int_8; sym_size_int_7 = sym_size_int_8 = eq_14 = None
sym_size_int_9: "Sym(s1)" = torch.ops.aten.sym_size.int(slice_13, 2)
sym_size_int_10: "Sym(s1)" = torch.ops.aten.sym_size.int(slice_5, 2); slice_5 = None
eq_15: "Sym(True)" = sym_size_int_9 == sym_size_int_10; sym_size_int_9 = sym_size_int_10 = eq_15 = None
eq_16: "Sym(True)" = sym_size_int_5 == sym_size_int_5; sym_size_int_5 = eq_16 = None
copy_: "f32[s0, 1, s1, s3]" = torch.ops.aten.copy_.default(slice_13, masked_fill); slice_13 = masked_fill = copy_ = None
# File: /home/xadupre/vv/this312/lib/python3.12/site-packages/transformers/models/phi3/modeling_phi3.py:611 in forward, code: position_embeddings = self.rotary_emb(hidden_states, position_ids)
_set_grad_enabled = torch._C._set_grad_enabled(False); _set_grad_enabled = None
# File: /home/xadupre/vv/this312/lib/python3.12/site-packages/transformers/models/phi3/modeling_phi3.py:362 in forward, code: self._longrope_frequency_update(position_ids, device=x.device)
max_1: "i64[]" = torch.ops.aten.max.default(unsqueeze); unsqueeze = None
add_3: "i64[]" = torch.ops.aten.add.Tensor(max_1, 1); max_1 = None
gt_2: "b8[]" = torch.ops.aten.gt.Scalar(add_3, 4096); add_3 = None
ne: "b8[]" = torch.ops.aten.ne.Scalar(gt_2, 0); gt_2 = None
item: "Sym(Eq(u0, 1))" = torch.ops.aten.item.default(ne); ne = item = None
# File: /home/xadupre/vv/this312/lib/python3.12/site-packages/transformers/models/phi3/modeling_phi3.py:611 in forward, code: position_embeddings = self.rotary_emb(hidden_states, position_ids)
_set_grad_enabled_1 = torch._C._set_grad_enabled(True); _set_grad_enabled_1 = None
/home/xadupre/vv/this312/lib/python3.12/site-packages/torch/backends/mkldnn/__init__.py:78: UserWarning: TF32 acceleration on top of oneDNN is available for Intel GPUs. The current Torch version does not have Intel GPU Support. (Triggered internally at /pytorch/aten/src/ATen/Context.cpp:148.)
torch._C._set_onednn_allow_tf32(_allow_tf32)
[try_export-FX] .. M:model-Phi3Model --- FAIL, step=EXPORT, reason=Could not guard on data-dependent expression Eq(u0, 1) (unhinted: Eq(u0, 1)). (Size-like symbols: none)
/home/xadupre/vv/this312/lib/python3.12/site-packages/torch/backends/mkldnn/__init__.py:78: UserWarning: TF32 acceleration on top of oneDNN is available for Intel GPUs. The current Torch version does not have Intel GPU Support. (Triggered internally at /pytorch/aten/src/ATen/Context.cpp:148.)
torch._C._set_onednn_allow_tf32(_allow_tf32)
[try_export-FX] .... M:embed_tokens-Embedding --- OK:
/home/xadupre/vv/this312/lib/python3.12/site-packages/torch/backends/mkldnn/__init__.py:78: UserWarning: TF32 acceleration on top of oneDNN is available for Intel GPUs. The current Torch version does not have Intel GPU Support. (Triggered internally at /pytorch/aten/src/ATen/Context.cpp:148.)
torch._C._set_onednn_allow_tf32(_allow_tf32)
/home/xadupre/vv/this312/lib/python3.12/site-packages/torch/backends/mkldnn/__init__.py:78: UserWarning: TF32 acceleration on top of oneDNN is available for Intel GPUs. The current Torch version does not have Intel GPU Support. (Triggered internally at /pytorch/aten/src/ATen/Context.cpp:148.)
torch._C._set_onednn_allow_tf32(_allow_tf32)
/home/xadupre/vv/this312/lib/python3.12/site-packages/torch/backends/mkldnn/__init__.py:78: UserWarning: TF32 acceleration on top of oneDNN is available for Intel GPUs. The current Torch version does not have Intel GPU Support. (Triggered internally at /pytorch/aten/src/ATen/Context.cpp:148.)
torch._C._set_onednn_allow_tf32(_allow_tf32)
[try_export-FX] ........ M:o_proj-Linear --- OK:
/home/xadupre/vv/this312/lib/python3.12/site-packages/torch/backends/mkldnn/__init__.py:78: UserWarning: TF32 acceleration on top of oneDNN is available for Intel GPUs. The current Torch version does not have Intel GPU Support. (Triggered internally at /pytorch/aten/src/ATen/Context.cpp:148.)
torch._C._set_onednn_allow_tf32(_allow_tf32)
[try_export-FX] ........ M:qkv_proj-Linear --- OK:
/home/xadupre/vv/this312/lib/python3.12/site-packages/torch/backends/mkldnn/__init__.py:78: UserWarning: TF32 acceleration on top of oneDNN is available for Intel GPUs. The current Torch version does not have Intel GPU Support. (Triggered internally at /pytorch/aten/src/ATen/Context.cpp:148.)
torch._C._set_onednn_allow_tf32(_allow_tf32)
[try_export-FX] ...... M:mlp-Phi3MLP --- OK:
/home/xadupre/vv/this312/lib/python3.12/site-packages/torch/backends/mkldnn/__init__.py:78: UserWarning: TF32 acceleration on top of oneDNN is available for Intel GPUs. The current Torch version does not have Intel GPU Support. (Triggered internally at /pytorch/aten/src/ATen/Context.cpp:148.)
torch._C._set_onednn_allow_tf32(_allow_tf32)
[try_export-FX] ...... M:input_layernorm-Phi3RMSNorm --- OK:
/home/xadupre/vv/this312/lib/python3.12/site-packages/torch/backends/mkldnn/__init__.py:78: UserWarning: TF32 acceleration on top of oneDNN is available for Intel GPUs. The current Torch version does not have Intel GPU Support. (Triggered internally at /pytorch/aten/src/ATen/Context.cpp:148.)
torch._C._set_onednn_allow_tf32(_allow_tf32)
[try_export-FX] ...... M:post_attention_layernorm-Phi3RMSNorm --- OK:
/home/xadupre/vv/this312/lib/python3.12/site-packages/torch/backends/mkldnn/__init__.py:78: UserWarning: TF32 acceleration on top of oneDNN is available for Intel GPUs. The current Torch version does not have Intel GPU Support. (Triggered internally at /pytorch/aten/src/ATen/Context.cpp:148.)
torch._C._set_onednn_allow_tf32(_allow_tf32)
[try_export-FX] ...... M:resid_attn_dropout-Dropout --- OK:
/home/xadupre/vv/this312/lib/python3.12/site-packages/torch/backends/mkldnn/__init__.py:78: UserWarning: TF32 acceleration on top of oneDNN is available for Intel GPUs. The current Torch version does not have Intel GPU Support. (Triggered internally at /pytorch/aten/src/ATen/Context.cpp:148.)
torch._C._set_onednn_allow_tf32(_allow_tf32)
[try_export-FX] ...... M:resid_mlp_dropout-Dropout --- OK:
/home/xadupre/vv/this312/lib/python3.12/site-packages/torch/backends/mkldnn/__init__.py:78: UserWarning: TF32 acceleration on top of oneDNN is available for Intel GPUs. The current Torch version does not have Intel GPU Support. (Triggered internally at /pytorch/aten/src/ATen/Context.cpp:148.)
torch._C._set_onednn_allow_tf32(_allow_tf32)
/home/xadupre/vv/this312/lib/python3.12/site-packages/torch/backends/mkldnn/__init__.py:78: UserWarning: TF32 acceleration on top of oneDNN is available for Intel GPUs. The current Torch version does not have Intel GPU Support. (Triggered internally at /pytorch/aten/src/ATen/Context.cpp:148.)
torch._C._set_onednn_allow_tf32(_allow_tf32)
/home/xadupre/vv/this312/lib/python3.12/site-packages/torch/backends/mkldnn/__init__.py:78: UserWarning: TF32 acceleration on top of oneDNN is available for Intel GPUs. The current Torch version does not have Intel GPU Support. (Triggered internally at /pytorch/aten/src/ATen/Context.cpp:148.)
torch._C._set_onednn_allow_tf32(_allow_tf32)
[try_export-FX] ........ M:o_proj-Linear --- OK:
/home/xadupre/vv/this312/lib/python3.12/site-packages/torch/backends/mkldnn/__init__.py:78: UserWarning: TF32 acceleration on top of oneDNN is available for Intel GPUs. The current Torch version does not have Intel GPU Support. (Triggered internally at /pytorch/aten/src/ATen/Context.cpp:148.)
torch._C._set_onednn_allow_tf32(_allow_tf32)
[try_export-FX] ........ M:qkv_proj-Linear --- OK:
/home/xadupre/vv/this312/lib/python3.12/site-packages/torch/backends/mkldnn/__init__.py:78: UserWarning: TF32 acceleration on top of oneDNN is available for Intel GPUs. The current Torch version does not have Intel GPU Support. (Triggered internally at /pytorch/aten/src/ATen/Context.cpp:148.)
torch._C._set_onednn_allow_tf32(_allow_tf32)
[try_export-FX] ...... M:mlp-Phi3MLP --- OK:
/home/xadupre/vv/this312/lib/python3.12/site-packages/torch/backends/mkldnn/__init__.py:78: UserWarning: TF32 acceleration on top of oneDNN is available for Intel GPUs. The current Torch version does not have Intel GPU Support. (Triggered internally at /pytorch/aten/src/ATen/Context.cpp:148.)
torch._C._set_onednn_allow_tf32(_allow_tf32)
[try_export-FX] ...... M:input_layernorm-Phi3RMSNorm --- OK:
/home/xadupre/vv/this312/lib/python3.12/site-packages/torch/backends/mkldnn/__init__.py:78: UserWarning: TF32 acceleration on top of oneDNN is available for Intel GPUs. The current Torch version does not have Intel GPU Support. (Triggered internally at /pytorch/aten/src/ATen/Context.cpp:148.)
torch._C._set_onednn_allow_tf32(_allow_tf32)
[try_export-FX] ...... M:post_attention_layernorm-Phi3RMSNorm --- OK:
/home/xadupre/vv/this312/lib/python3.12/site-packages/torch/backends/mkldnn/__init__.py:78: UserWarning: TF32 acceleration on top of oneDNN is available for Intel GPUs. The current Torch version does not have Intel GPU Support. (Triggered internally at /pytorch/aten/src/ATen/Context.cpp:148.)
torch._C._set_onednn_allow_tf32(_allow_tf32)
[try_export-FX] ...... M:resid_attn_dropout-Dropout --- OK:
/home/xadupre/vv/this312/lib/python3.12/site-packages/torch/backends/mkldnn/__init__.py:78: UserWarning: TF32 acceleration on top of oneDNN is available for Intel GPUs. The current Torch version does not have Intel GPU Support. (Triggered internally at /pytorch/aten/src/ATen/Context.cpp:148.)
torch._C._set_onednn_allow_tf32(_allow_tf32)
[try_export-FX] ...... M:resid_mlp_dropout-Dropout --- OK:
/home/xadupre/vv/this312/lib/python3.12/site-packages/torch/backends/mkldnn/__init__.py:78: UserWarning: TF32 acceleration on top of oneDNN is available for Intel GPUs. The current Torch version does not have Intel GPU Support. (Triggered internally at /pytorch/aten/src/ATen/Context.cpp:148.)
torch._C._set_onednn_allow_tf32(_allow_tf32)
[try_export-FX] .... M:norm-Phi3RMSNorm --- OK:
def forward(self, arg0_1: "f32[48]", arg1_1: "f32[s0, s1, 3072]", arg2_1: "i64[1, s2]"):
# No stacktrace found for following nodes
_set_grad_enabled = torch._C._set_grad_enabled(False); _set_grad_enabled = None
# File: /home/xadupre/vv/this312/lib/python3.12/site-packages/transformers/models/phi3/modeling_phi3.py:362 in forward, code: self._longrope_frequency_update(position_ids, device=x.device)
max_1: "i64[]" = torch.ops.aten.max.default(arg2_1); arg2_1 = None
add: "i64[]" = torch.ops.aten.add.Tensor(max_1, 1); max_1 = None
gt: "b8[]" = torch.ops.aten.gt.Scalar(add, 4096); add = None
ne: "b8[]" = torch.ops.aten.ne.Scalar(gt, 0); gt = None
item: "Sym(Eq(u0, 1))" = torch.ops.aten.item.default(ne); ne = item = None
# No stacktrace found for following nodes
_set_grad_enabled_1 = torch._C._set_grad_enabled(True); _set_grad_enabled_1 = None
/home/xadupre/vv/this312/lib/python3.12/site-packages/torch/backends/mkldnn/__init__.py:78: UserWarning: TF32 acceleration on top of oneDNN is available for Intel GPUs. The current Torch version does not have Intel GPU Support. (Triggered internally at /pytorch/aten/src/ATen/Context.cpp:148.)
torch._C._set_onednn_allow_tf32(_allow_tf32)
[try_export-FX] .... M:rotary_emb-Phi3RotaryEmbedding --- FAIL, step=EXPORT, reason=Could not guard on data-dependent expression Eq(u0, 1) (unhinted: Eq(u0, 1)). (Size-like symbols: none)
[try_export-FX] .... M:rotary_emb-Phi3RotaryEmbedding --- FAIL: Could not guard on data-depend...
/home/xadupre/vv/this312/lib/python3.12/site-packages/torch/backends/mkldnn/__init__.py:78: UserWarning: TF32 acceleration on top of oneDNN is available for Intel GPUs. The current Torch version does not have Intel GPU Support. (Triggered internally at /pytorch/aten/src/ATen/Context.cpp:148.)
torch._C._set_onednn_allow_tf32(_allow_tf32)
[try_export-FX] .. M:lm_head-Linear --- OK:
Let’s display a report.
print(f"success: {ep.status}")
print(diag.get_export_report())
success: 2
__main__ Phi3ForCausalLM FAIL -- step=EXPORT, reason='Could not guard on data-dependent expression Eq(u0, 1) (unhinted: Eq(u0, 1)). (Size-like symbols: n...'
..model Phi3Model FAIL -- step=EXPORT, reason='Could not guard on data-dependent expression Eq(u0, 1) (unhinted: Eq(u0, 1)). (Size-like symbols: n...'
....embed_tokens Embedding OK -- ExportedProgram
....layers[0] Phi3DecoderLayer FAIL -- step=, reason='mat1 and mat2 shapes cannot be multiplied (8x4608 and 3072x3072)'
......self_attn Phi3Attention FAIL -- step=, reason='mat1 and mat2 shapes cannot be multiplied (8x4608 and 3072x3072)'
........o_proj Linear OK -- ExportedProgram
........qkv_proj Linear OK -- ExportedProgram
......mlp Phi3MLP OK -- ExportedProgram
........gate_up_proj Linear <OK-2i>
........down_proj Linear <OK-2i>
........activation_fn SiLU <OK-2i>
......input_layernorm Phi3RMSNorm OK -- ExportedProgram
......post_attention_layernorm Phi3RMSNorm OK -- ExportedProgram
......resid_attn_dropout Dropout OK -- ExportedProgram
......resid_mlp_dropout Dropout OK -- ExportedProgram
....layers[1] Phi3DecoderLayer FAIL -- step=, reason='mat1 and mat2 shapes cannot be multiplied (8x4608 and 3072x3072)'
......self_attn Phi3Attention FAIL -- step=, reason='mat1 and mat2 shapes cannot be multiplied (8x4608 and 3072x3072)'
........o_proj Linear OK -- ExportedProgram
........qkv_proj Linear OK -- ExportedProgram
......mlp Phi3MLP OK -- ExportedProgram
........gate_up_proj Linear <OK-2i>
........down_proj Linear <OK-2i>
........activation_fn SiLU <OK-2i>
......input_layernorm Phi3RMSNorm OK -- ExportedProgram
......post_attention_layernorm Phi3RMSNorm OK -- ExportedProgram
......resid_attn_dropout Dropout OK -- ExportedProgram
......resid_mlp_dropout Dropout OK -- ExportedProgram
....norm Phi3RMSNorm OK -- ExportedProgram
....rotary_emb Phi3RotaryEmbedding FAIL -- step=EXPORT, reason='Could not guard on data-dependent expression Eq(u0, 1) (unhinted: Eq(u0, 1)). (Size-like symbols: n...'
..lm_head Linear OK -- ExportedProgram
Replace the failing module by a custom op¶
The main module is not exportable because one piece cannot be exported. But maybe if we assume it works, maybe everything else is working. So let’s try to replace this class by a custom op. This will be something for another example.
Total running time of the script: (0 minutes 13.644 seconds)
Related examples
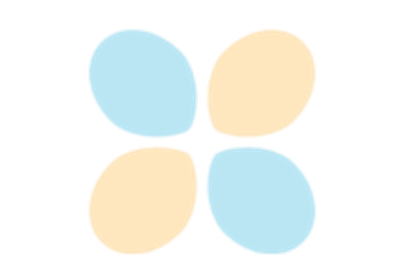
Export Phi-3.5-mini-instruct with report_exportability