Note
Go to the end to download the full example code.
to_onnx and a custom operator inplace¶
This example shows how to convert a custom operator as defined in the tutorial Python Custom Operators.
Inplace modification are not supported by onnx.
A model with a custom ops¶
from typing import Any, Dict, List, Optional
import numpy as np
import torch
from onnx_array_api.plotting.graphviz_helper import plot_dot
from experimental_experiment.xbuilder import GraphBuilder
from experimental_experiment.helpers import pretty_onnx
from experimental_experiment.torch_interpreter import to_onnx, Dispatcher, ExportOptions
We define a model with a custom operator.
@torch.library.custom_op("mylib::numpy_sin", mutates_args={"output"}, device_types="cpu")
def numpy_sin(x: torch.Tensor, output: torch.Tensor) -> None:
assert x.device == output.device
assert x.device.type == "cpu"
x_np = x.numpy()
output_np = output.numpy()
np.sin(x_np, out=output_np)
class ModuleWithACustomOperator(torch.nn.Module):
def forward(self, x):
out = torch.zeros(x.shape)
numpy_sin(x, out)
return out
model = ModuleWithACustomOperator()
Let’s check it runs.
x = torch.randn(1, 3)
model(x)
tensor([[ 0.2549, -0.7778, -0.6156]])
As expected, it does not export.
try:
torch.export.export(model, (x,))
raise AssertionError("This export should failed unless pytorch now supports this model.")
except Exception as e:
print(e)
This export should failed unless pytorch now supports this model.
The exporter fails with the same eror as it expects torch.export.export to work.
Registration¶
The exporter how to convert the new exporter into ONNX. This must be defined. The first piece is to tell the exporter that the shape of the output is the same as x. input names must be the same.
@numpy_sin.register_fake
def numpy_sin_shape(x, output):
pass
Let’s see what the fx graph looks like.
print(torch.export.export(model, (x,)).graph)
graph():
%x : [num_users=1] = placeholder[target=x]
%zeros : [num_users=2] = call_function[target=torch.ops.aten.zeros.default](args = ([1, 3],), kwargs = {device: cpu, pin_memory: False})
%numpy_sin : [num_users=0] = call_function[target=torch.ops.mylib.numpy_sin.default](args = (%x, %zeros), kwargs = {})
return (zeros,)
Next is the conversion to onnx.
T = str # a tensor name
def numpy_sin_to_onnx(
g: GraphBuilder,
sts: Dict[str, Any],
outputs: List[str],
x: T,
output: Optional[T] = None,
name: str = "mylib.numpy_sin",
) -> T:
# name= ... lets the user know when the node comes from
# o is not used, we could check the shape are equal.
# outputs contains unexpectedly two outputs
g.op.Sin(x, name=name, outputs=outputs[1:])
return outputs
We create a Dispatcher
.
dispatcher = Dispatcher({"mylib::numpy_sin": numpy_sin_to_onnx})
And we convert again.
onx = to_onnx(
model,
(x,),
dispatcher=dispatcher,
optimize=False,
export_options=ExportOptions(decomposition_table="default"),
)
print(pretty_onnx(onx))
opset: domain='' version=18
input: name='x' type=dtype('float32') shape=[1, 3]
init: name='init7_s2_1_3' type=int64 shape=(2,) -- array([1, 3]) -- Opset.make_node.1/Shape
ConstantOfShape(init7_s2_1_3, value=[nan]) -> zeros
Sin(x) -> auto_functionalized#1
Identity(auto_functionalized#1) -> getitem_1
Identity(getitem_1) -> output_0
output: name='output_0' type=dtype('float32') shape=[1, 3]
And we convert again with optimization this time.
onx = to_onnx(
model,
(x,),
dispatcher=dispatcher,
optimize=True,
export_options=ExportOptions(decomposition_table="default"),
)
print(pretty_onnx(onx))
opset: domain='' version=18
input: name='x' type=dtype('float32') shape=[1, 3]
Sin(x) -> output_0
output: name='output_0' type=dtype('float32') shape=[1, 3]
And visually.
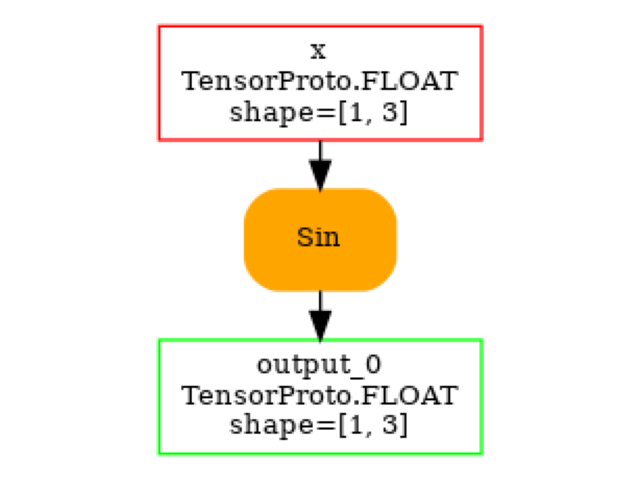
Total running time of the script: (0 minutes 4.229 seconds)
Related examples
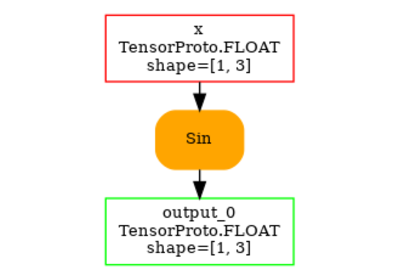
to_onnx and a custom operator registered with a function