Note
Go to the end to download the full example code.
Export a LLAMA model into ONNX¶
This script does not export a full llama model but a shorter one to be able to fast iterate on improvments. See LlamaConfig. The model is then converted into ONNX. It can be seen with Netron which can be also used through a VS Code Extension.
The model¶
import os
import random
def ids_tensor(shape, vocab_size, rng=None, name=None):
# Creates a random int32 tensor of the shape within the vocab size
import torch
if rng is None:
rng = random.Random()
total_dims = 1
for dim in shape:
total_dims *= dim
values = []
for _ in range(total_dims):
values.append(rng.randint(0, vocab_size - 1))
return torch.tensor(data=values, dtype=torch.long).view(shape).contiguous()
def get_llama_model(
input_dims=[(2, 1024)], # noqa: B006
hidden_size=1024, # 4096,
num_hidden_layers=1,
vocab_size=32000,
intermediate_size=11008,
max_position_embeddings=2048,
num_attention_heads=4, # 32,
_attn_implementation="eager",
with_mask: bool = True,
):
import torch
from transformers import LlamaConfig
from transformers.models.llama.modeling_llama import LlamaModel
config = LlamaConfig(
num_hidden_layers=num_hidden_layers,
vocab_size=vocab_size,
hidden_size=hidden_size,
intermediate_size=intermediate_size,
max_position_embeddings=max_position_embeddings,
num_attention_heads=num_attention_heads,
)
if _attn_implementation:
config._attn_implementation = _attn_implementation
class LlamaModelWrapper(torch.nn.Module):
def __init__(self, config):
super().__init__()
self.model = LlamaModel(config)
def forward(self, input_ids, attention_mask):
model_output = self.model(input_ids, attention_mask=attention_mask)
return model_output.to_tuple()
def generate_example_inputs(batch: int, seq: int, vocab_size: int):
input_ids = ids_tensor([batch, seq], vocab_size)
input_mask = torch.tril(torch.ones(batch, seq, dtype=torch.float32))
assert input_mask.dtype == torch.float32
return input_ids, input_mask
example_args_collection = []
for b, s in input_dims:
example_args_collection.append(generate_example_inputs(b, s, vocab_size))
return LlamaModelWrapper(config), example_args_collection
print("creation of the model.")
model, example_args_collection = get_llama_model()
print("done.")
creation of the model.
done.
The conversion to ONNX¶
def export(model, args, filename, dynamic_shapes):
from experimental_experiment.torch_interpreter import to_onnx, ExportOptions
from onnx_diagnostic.torch_export_patches import bypass_export_some_errors
with bypass_export_some_errors(patch_transformers=True):
to_onnx(
model,
args,
filename=filename,
target_opset=18,
dynamic_shapes=dynamic_shapes,
export_options=ExportOptions(strict=False),
)
filename = "dump_llama.onnx"
print(f"conversion to ONNX in file {filename!r}")
export(
model,
example_args_collection[0],
filename,
dynamic_shapes=({0: "batch", 1: "seq_length"}, {0: "batch", 1: "seq_length"}),
)
print("done.")
print(f"model size {os.stat(filename).st_size / 2**20} Mb.")
conversion to ONNX in file 'dump_llama.onnx'
done.
model size 270.04535579681396 Mb.
This gives the following in Netron:
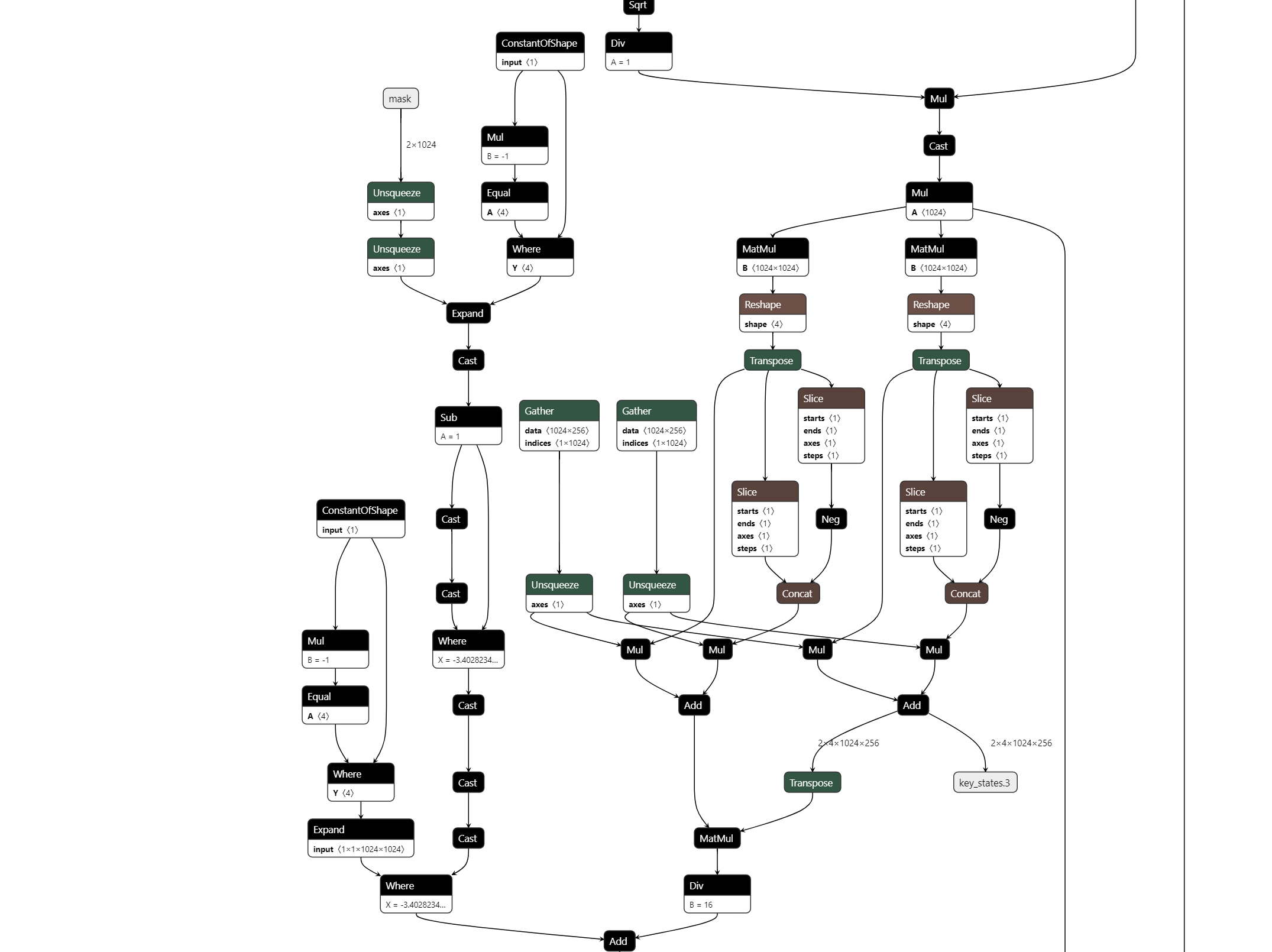
Total running time of the script: (0 minutes 6.892 seconds)