Note
Go to the end to download the full example code
Compares filtering implementations (numpy, cython)¶
The benchmark looks into different ways to implement
thresholding: every value of a vector superior to mx
is replaced by mx (numpy.clip()
).
It compares several implementation to numpy.
import pprint
import numpy
import matplotlib.pyplot as plt
from pandas import DataFrame
from teachcompute.validation.cython.experiment_cython import (
pyfilter_dmax,
filter_dmax_cython,
filter_dmax_cython_optim,
cyfilter_dmax,
cfilter_dmax,
cfilter_dmax2,
cfilter_dmax16,
cfilter_dmax4,
)
from teachcompute.ext_test_case import measure_time_dim
def get_vectors(fct, n, h=200, dtype=numpy.float64):
ctxs = [
dict(
va=numpy.random.randn(n).astype(dtype),
fil=fct,
mx=numpy.float64(0),
x_name=n,
)
for n in range(10, n, h)
]
return ctxs
def numpy_filter(va, mx):
va[va > mx] = mx
all_res = []
for fct in [
numpy_filter,
pyfilter_dmax,
filter_dmax_cython,
filter_dmax_cython_optim,
cyfilter_dmax,
cfilter_dmax,
cfilter_dmax2,
cfilter_dmax16,
cfilter_dmax4,
]:
print(fct)
ctxs = get_vectors(fct, 1000 if fct == pyfilter_dmax else 40000)
res = list(measure_time_dim("fil(va, mx)", ctxs, verbose=1))
for r in res:
r["fct"] = fct.__name__
all_res.extend(res)
pprint.pprint(all_res[:2])
<function numpy_filter at 0x7ff2e6763b50>
0%| | 0/200 [00:00<?, ?it/s]
30%|███ | 60/200 [00:00<00:00, 590.09it/s]
60%|██████ | 120/200 [00:00<00:00, 406.31it/s]
82%|████████▏ | 164/200 [00:00<00:00, 309.15it/s]
100%|█████████▉| 199/200 [00:00<00:00, 217.43it/s]
100%|██████████| 200/200 [00:00<00:00, 263.15it/s]
<cyfunction pyfilter_dmax at 0x7ff32e47fc60>
0%| | 0/5 [00:00<?, ?it/s]
100%|██████████| 5/5 [00:00<00:00, 39.54it/s]
100%|██████████| 5/5 [00:00<00:00, 39.44it/s]
<cyfunction filter_dmax_cython at 0x7ff2fd0928e0>
0%| | 0/200 [00:00<?, ?it/s]
40%|███▉ | 79/200 [00:00<00:00, 789.85it/s]
79%|███████▉ | 158/200 [00:00<00:00, 403.12it/s]
100%|██████████| 200/200 [00:00<00:00, 364.30it/s]
<cyfunction filter_dmax_cython_optim at 0x7ff2fd092400>
0%| | 0/200 [00:00<?, ?it/s]
43%|████▎ | 86/200 [00:00<00:00, 843.32it/s]
86%|████████▌ | 171/200 [00:00<00:00, 367.13it/s]
100%|██████████| 200/200 [00:00<00:00, 340.45it/s]
<cyfunction cyfilter_dmax at 0x7ff2fd091be0>
0%| | 0/200 [00:00<?, ?it/s]
26%|██▌ | 51/200 [00:00<00:00, 509.43it/s]
51%|█████ | 102/200 [00:00<00:00, 490.98it/s]
76%|███████▌ | 152/200 [00:00<00:00, 379.40it/s]
96%|█████████▋| 193/200 [00:00<00:00, 311.63it/s]
100%|██████████| 200/200 [00:00<00:00, 340.26it/s]
<cyfunction cfilter_dmax at 0x7ff2e846ee90>
0%| | 0/200 [00:00<?, ?it/s]
30%|███ | 60/200 [00:00<00:00, 589.64it/s]
60%|█████▉ | 119/200 [00:00<00:00, 377.84it/s]
80%|████████ | 161/200 [00:00<00:00, 269.06it/s]
96%|█████████▋| 193/200 [00:00<00:00, 240.61it/s]
100%|██████████| 200/200 [00:00<00:00, 272.39it/s]
<cyfunction cfilter_dmax2 at 0x7ff2e846f850>
0%| | 0/200 [00:00<?, ?it/s]
46%|████▌ | 91/200 [00:00<00:00, 899.44it/s]
90%|█████████ | 181/200 [00:00<00:00, 480.37it/s]
100%|██████████| 200/200 [00:00<00:00, 457.30it/s]
<cyfunction cfilter_dmax16 at 0x7ff2e846f2a0>
0%| | 0/200 [00:00<?, ?it/s]
24%|██▍ | 49/200 [00:00<00:00, 485.73it/s]
49%|████▉ | 98/200 [00:00<00:00, 238.28it/s]
64%|██████▍ | 129/200 [00:00<00:00, 186.34it/s]
76%|███████▌ | 152/200 [00:00<00:00, 151.77it/s]
85%|████████▌ | 170/200 [00:01<00:00, 131.02it/s]
92%|█████████▎| 185/200 [00:01<00:00, 95.66it/s]
98%|█████████▊| 197/200 [00:01<00:00, 76.03it/s]
100%|██████████| 200/200 [00:01<00:00, 118.11it/s]
<cyfunction cfilter_dmax4 at 0x7ff2e846f370>
0%| | 0/200 [00:00<?, ?it/s]
25%|██▌ | 50/200 [00:00<00:00, 491.18it/s]
50%|█████ | 100/200 [00:00<00:00, 252.00it/s]
66%|██████▌ | 132/200 [00:00<00:00, 197.74it/s]
78%|███████▊ | 156/200 [00:00<00:00, 163.05it/s]
88%|████████▊ | 175/200 [00:01<00:00, 140.04it/s]
96%|█████████▌| 191/200 [00:01<00:00, 102.01it/s]
100%|██████████| 200/200 [00:01<00:00, 133.75it/s]
[{'average': 1.1421999952290207e-06,
'context_size': 232,
'deviation': 1.2789058602498825e-08,
'fct': 'numpy_filter',
'max_exec': 1.1799999992945231e-06,
'min_exec': 1.1359999916749075e-06,
'number': 50,
'repeat': 10,
'ttime': 1.1421999952290207e-05,
'warmup_time': 2.9200000426499173e-05,
'x_name': 10},
{'average': 1.1563999978534411e-06,
'context_size': 232,
'deviation': 7.578910748483153e-09,
'fct': 'numpy_filter',
'max_exec': 1.1779999840655364e-06,
'min_exec': 1.1500000255182385e-06,
'number': 50,
'repeat': 10,
'ttime': 1.1563999978534411e-05,
'warmup_time': 1.089999932446517e-05,
'x_name': 210}]
Let’s display the results¶
cc = DataFrame(all_res)
cc["N"] = cc["x_name"]
fig, ax = plt.subplots(2, 2, figsize=(10, 10))
cc[cc.N <= 1100].pivot(index="N", columns="fct", values="average").plot(
logy=True, ax=ax[0, 0]
)
cc[cc.fct != "pyfilter_dmax"].pivot(index="N", columns="fct", values="average").plot(
logy=True, ax=ax[0, 1]
)
cc[cc.fct != "pyfilter_dmax"].pivot(index="N", columns="fct", values="average").plot(
logy=True, logx=True, ax=ax[1, 1]
)
cc[(cc.fct.str.contains("cfilter") | cc.fct.str.contains("numpy"))].pivot(
index="N", columns="fct", values="average"
).plot(logy=True, ax=ax[1, 0])
ax[0, 0].set_title("Comparison of filter implementations")
ax[0, 1].set_title("Comparison of filter implementations\n" "without pyfilter_dmax")
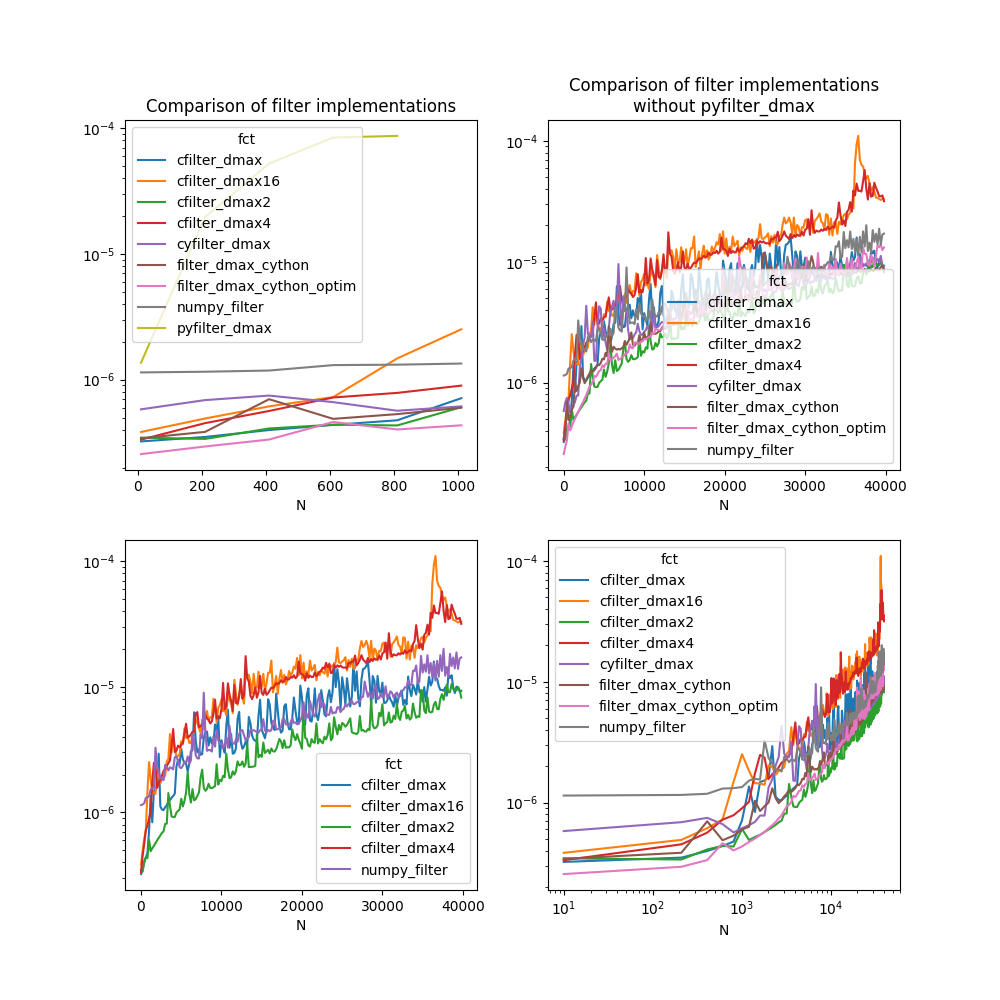
Text(0.5, 1.0, 'Comparison of filter implementations\nwithout pyfilter_dmax')
The results depends on the machine, its number of cores, the compilation settings of numpy or this module.
Total running time of the script: (0 minutes 9.877 seconds)