Note
Go to the end to download the full example code
Associativity and matrix multiplication¶
The matrix multiplication m1 @ m2 @ m3 can be done in two different ways: (m1 @ m2) @ m3 or m1 @ (m2 @ m3). Are these two orders equivalent or is there a better order?
import pprint
import numpy
import matplotlib.pyplot as plt
from pandas import DataFrame
from tqdm import tqdm
from teachcompute.ext_test_case import measure_time
First try¶
m1 = numpy.random.rand(100, 100)
m2 = numpy.random.rand(100, 10)
m3 = numpy.random.rand(10, 100)
m = m1 @ m2 @ m3
print(m.shape)
mm1 = (m1 @ m2) @ m3
mm2 = m1 @ (m2 @ m3)
print(mm1.shape, mm2.shape)
t1 = measure_time(lambda: (m1 @ m2) @ m3, context={}, number=50, repeat=50)
pprint.pprint(t1)
t2 = measure_time(lambda: m1 @ (m2 @ m3), context={}, number=50, repeat=50)
pprint.pprint(t2)
(100, 100)
(100, 100) (100, 100)
{'average': 7.918572000035058e-05,
'context_size': 64,
'deviation': 7.472517220805028e-05,
'max_exec': 0.0002890740000020742,
'min_exec': 2.662199999576842e-05,
'number': 50,
'repeat': 50,
'ttime': 0.003959286000017529,
'warmup_time': 0.00012929999957123073}
{'average': 0.003669156359999761,
'context_size': 64,
'deviation': 0.0058324362524603416,
'max_exec': 0.021446986000000834,
'min_exec': 7.507799999984854e-05,
'number': 50,
'repeat': 50,
'ttime': 0.18345781799998806,
'warmup_time': 0.025857000000087282}
With different sizes¶
obs = []
for i in tqdm([50, 100, 125, 150, 175, 200]):
m1 = numpy.random.rand(i, i)
m2 = numpy.random.rand(i, 10)
m3 = numpy.random.rand(10, i)
t1 = measure_time(lambda: (m1 @ m2) @ m3, context={}, number=50, repeat=50)
t1["formula"] = "(m1 @ m2) @ m3"
t1["size"] = i
obs.append(t1)
t2 = measure_time(lambda: m1 @ (m2 @ m3), context={}, number=50, repeat=50)
t2["formula"] = "m1 @ (m2 @ m3)"
t2["size"] = i
obs.append(t2)
df = DataFrame(obs)
piv = df.pivot(index="size", columns="formula", values="average")
piv
0%| | 0/6 [00:00<?, ?it/s]
17%|█▋ | 1/6 [00:00<00:00, 5.72it/s]
33%|███▎ | 2/6 [00:02<00:04, 1.16s/it]
50%|█████ | 3/6 [00:02<00:02, 1.12it/s]
67%|██████▋ | 4/6 [00:04<00:02, 1.10s/it]
83%|████████▎ | 5/6 [00:04<00:01, 1.04s/it]
100%|██████████| 6/6 [00:06<00:00, 1.26s/it]
100%|██████████| 6/6 [00:06<00:00, 1.11s/it]
Graph¶
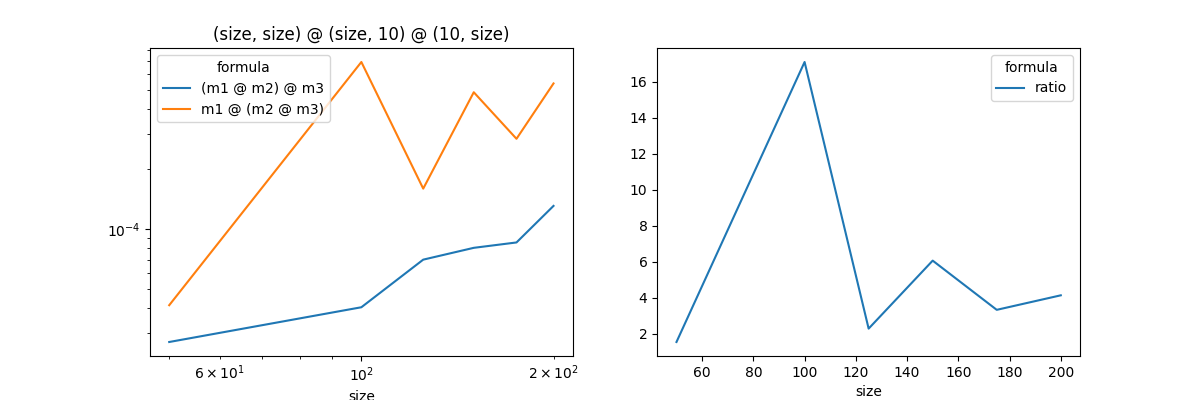
<Axes: xlabel='size'>
Total running time of the script: (0 minutes 16.443 seconds)