Note
Go to the end to download the full example code.
Parallelization of a dot product with processes (concurrent.futures)¶
Uses processes to parallelize a dot product is not a very solution because processes do not share memory, they need to exchange data. This parallelisation is efficient if the ratio exchanged data / computation time is low. This example uses concurrent.futures. The cost of creating new processes is also significant.
import numpy
from tqdm import tqdm
from pandas import DataFrame
import matplotlib.pyplot as plt
import concurrent.futures as cf
from teachcompute.ext_test_case import measure_time
def parallel_numpy_dot(va, vb, max_workers=2):
if max_workers == 2:
with cf.ThreadPoolExecutor(max_workers=max_workers) as e:
m = va.shape[0] // 2
f1 = e.submit(numpy.dot, va[:m], vb[:m])
f2 = e.submit(numpy.dot, va[m:], vb[m:])
return f1.result() + f2.result()
elif max_workers == 3:
with cf.ThreadPoolExecutor(max_workers=max_workers) as e:
m = va.shape[0] // 3
m2 = va.shape[0] * 2 // 3
f1 = e.submit(numpy.dot, va[:m], vb[:m])
f2 = e.submit(numpy.dot, va[m:m2], vb[m:m2])
f3 = e.submit(numpy.dot, va[m2:], vb[m2:])
return f1.result() + f2.result() + f3.result()
else:
raise NotImplementedError()
We check that it returns the same values.
va = numpy.random.randn(100).astype(numpy.float64)
vb = numpy.random.randn(100).astype(numpy.float64)
print(parallel_numpy_dot(va, vb), numpy.dot(va, vb))
-0.8619366999916398 -0.8619366999916395
Let’s benchmark.
res = []
for n in tqdm([100000, 1000000, 10000000, 100000000]):
va = numpy.random.randn(n).astype(numpy.float64)
vb = numpy.random.randn(n).astype(numpy.float64)
m1 = measure_time("dot(va, vb, 2)", dict(va=va, vb=vb, dot=parallel_numpy_dot))
m2 = measure_time("dot(va, vb)", dict(va=va, vb=vb, dot=numpy.dot))
res.append({"N": n, "numpy.dot": m2["average"], "futures": m1["average"]})
df = DataFrame(res).set_index("N")
print(df)
df.plot(logy=True, logx=True)
plt.title("Parallel / numpy dot")
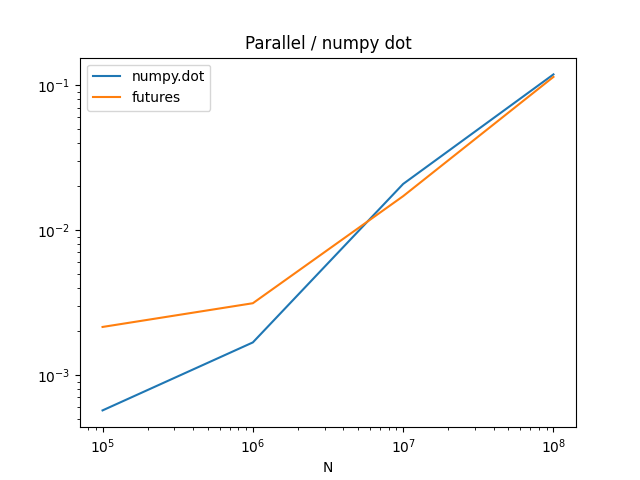
0%| | 0/4 [00:00<?, ?it/s]
25%|██▌ | 1/4 [00:01<00:05, 1.74s/it]
50%|█████ | 2/4 [00:03<00:04, 2.02s/it]
75%|███████▌ | 3/4 [00:09<00:03, 3.73s/it]
100%|██████████| 4/4 [00:54<00:00, 19.99s/it]
100%|██████████| 4/4 [00:54<00:00, 13.66s/it]
numpy.dot futures
N
100000 0.001159 0.002256
1000000 0.001509 0.002778
10000000 0.004062 0.006457
100000000 0.046675 0.039240
Text(0.5, 1.0, 'Parallel / numpy dot')
The parallelisation is inefficient unless the vectors are big.
Total running time of the script: (0 minutes 54.830 seconds)