Note
Go to the end to download the full example code.
201: Evaluate DORT¶
It compares DORT to eager mode and onnxrt backend.
To run the script:
python _doc/examples/plot_torch_dort --help
Some helpers¶
import warnings
try:
with warnings.catch_warnings():
warnings.simplefilter("ignore")
import onnxruntime
has_cuda = "CUDAExecutionProvider" in onnxruntime.get_available_providers()
except ImportError:
print("onnxruntime not available.")
import sys
sys.exit(0)
import torch._dynamo
import contextlib
import itertools
import gc
import platform
# import pickle
import pprint
import multiprocessing
import time
import io
import logging
import numpy as np
import matplotlib.pyplot as plt
import pandas
import torch
from torch import nn
import torch.nn.functional as F
from experimental_experiment.plotting.memory import memory_peak_plot
from experimental_experiment.ext_test_case import measure_time, get_figure
from experimental_experiment.args import get_parsed_args
from experimental_experiment.memory_peak import start_spying_on
from experimental_experiment.torch_models.training_helper import make_aot_ort
from tqdm import tqdm
has_cuda = has_cuda and torch.cuda.device_count() > 0
logging.disable(logging.ERROR)
def system_info():
obs = {}
obs["processor"] = platform.processor()
obs["cores"] = multiprocessing.cpu_count()
try:
obs["cuda"] = 1 if torch.cuda.device_count() > 0 else 0
obs["cuda_count"] = torch.cuda.device_count()
obs["cuda_name"] = torch.cuda.get_device_name()
obs["cuda_capa"] = torch.cuda.get_device_capability()
except (RuntimeError, AssertionError):
# no cuda
pass
return obs
pprint.pprint(system_info())
{'cores': 20,
'cuda': 1,
'cuda_capa': (8, 9),
'cuda_count': 1,
'cuda_name': 'NVIDIA GeForce RTX 4060 Laptop GPU',
'processor': 'x86_64'}
Scripts arguments
script_args = get_parsed_args(
"plot_torch_dort",
description=__doc__,
scenarios={
"small": "small model to test",
"middle": "55Mb model",
"large": "1Gb model",
},
warmup=5,
repeat=5,
repeat1=(1, "repeat for the first iteration"),
maxtime=(
2,
"maximum time to run a model to measure the computation time, "
"it is 0.1 when scenario is small",
),
expose="scenarios,repeat,repeat1,warmup",
)
if script_args.scenario in (None, "small"):
script_args.maxtime = 0.1
print(f"scenario={script_args.scenario or 'small'}")
print(f"warmup={script_args.warmup}")
print(f"repeat={script_args.repeat}")
print(f"repeat1={script_args.repeat1}")
print(f"maxtime={script_args.maxtime}")
scenario=small
warmup=5
repeat=5
repeat1=1
maxtime=0.1
The model¶
A simple model to convert.
class MyModelClass(nn.Module):
def __init__(self, scenario=script_args.scenario):
super().__init__()
if scenario == "middle":
self.large = False
self.conv1 = nn.Conv2d(1, 32, 5)
# self.conv2 = nn.Conv2d(128, 16, 5)
self.fc1 = nn.Linear(30752, 1024)
self.fcs = []
self.fc2 = nn.Linear(1024, 128)
self.fc3 = nn.Linear(128, 10)
elif scenario in (None, "small"):
self.large = False
self.conv1 = nn.Conv2d(1, 16, 5)
# self.conv2 = nn.Conv2d(16, 16, 5)
self.fc1 = nn.Linear(144, 512)
self.fcs = []
self.fc2 = nn.Linear(512, 128)
self.fc3 = nn.Linear(128, 10)
elif scenario in (None, "large"):
self.large = True
self.conv1 = nn.Conv2d(1, 32, 5)
# self.conv2 = nn.Conv2d(128, 16, 5)
self.fc1 = nn.Linear(30752, 4096)
# torch script does not support loops.
self.fca = nn.Linear(4096, 4096)
self.fcb = nn.Linear(4096, 4096)
self.fcc = nn.Linear(4096, 4096)
self.fcd = nn.Linear(4096, 4096)
self.fce = nn.Linear(4096, 4096)
self.fcf = nn.Linear(4096, 4096)
self.fcg = nn.Linear(4096, 4096)
self.fch = nn.Linear(4096, 4096)
self.fci = nn.Linear(4096, 4096)
# end of the unfolded loop.
self.fc2 = nn.Linear(4096, 128)
self.fc3 = nn.Linear(128, 10)
else:
raise ValueError(f"Unsupported scenario={scenario!r}.")
def forward(self, x):
x = F.max_pool2d(F.relu(self.conv1(x)), (4, 4))
# x = F.max_pool2d(F.relu(self.conv2(x)), 2)
x = torch.flatten(x, 1)
x = F.relu(self.fc1(x))
if self.large:
# loop
x = F.relu(self.fca(x))
x = F.relu(self.fcb(x))
x = F.relu(self.fcc(x))
x = F.relu(self.fcd(x))
x = F.relu(self.fce(x))
x = F.relu(self.fcf(x))
x = F.relu(self.fcg(x))
x = F.relu(self.fch(x))
x = F.relu(self.fci(x))
# end of the loop
x = F.relu(self.fc2(x))
y = self.fc3(x)
return y
def create_model_and_input(scenario=script_args.scenario):
if scenario == "middle":
shape = [1, 1, 128, 128]
elif scenario in (None, "small"):
shape = [1, 1, 16, 16]
elif scenario == "large":
shape = [1, 1, 128, 128]
else:
raise ValueError(f"Unsupported scenario={scenario!r}.")
input_tensor = torch.rand(*shape).to(torch.float32)
model = MyModelClass(scenario=scenario)
assert model(input_tensor) is not None
return model, input_tensor
def torch_model_size(model):
size_model = 0
for param in model.parameters():
size = param.numel() * torch.finfo(param.data.dtype).bits / 8
size_model += size
return size_model
model, input_tensor = create_model_and_input()
model_size = torch_model_size(model)
print(f"model size={model_size / 2 ** 20} Mb")
model size=0.5401992797851562 Mb
Backends¶
def get_torch_eager(model, *args):
def my_compiler(gm, example_inputs):
return gm.forward
with contextlib.redirect_stdout(io.StringIO()):
with warnings.catch_warnings():
warnings.simplefilter("ignore")
optimized_mod = torch.compile(model, fullgraph=True, backend=my_compiler)
optimized_mod(*args)
return optimized_mod
def get_torch_default(model, *args):
with contextlib.redirect_stdout(io.StringIO()):
with warnings.catch_warnings():
warnings.simplefilter("ignore")
optimized_mod = torch.compile(model, fullgraph=True, mode="reduce-overhead")
optimized_mod(*args)
return optimized_mod
def get_torch_dort(model, *args):
with contextlib.redirect_stdout(io.StringIO()):
with warnings.catch_warnings():
warnings.simplefilter("ignore")
local_aot_ort, _ = make_aot_ort(dynamic=True, rewrite=True)
optimized_mod = torch.compile(model, backend=local_aot_ort, fullgraph=True)
optimized_mod(*args)
return optimized_mod
def get_torch_opti(model, *args):
with contextlib.redirect_stdout(io.StringIO()):
with warnings.catch_warnings():
warnings.simplefilter("ignore")
local_aot_ort, _ = make_aot_ort(dynamic=True, rewrite=True)
optimized_mod = torch.compile(model, backend=local_aot_ort, fullgraph=True)
optimized_mod(*args)
return optimized_mod
Let’s check they are working.
export_functions = [
get_torch_eager,
get_torch_default,
get_torch_dort,
# get_torch_opti,
]
exporters = {f.__name__.replace("get_", ""): f for f in export_functions}
supported_exporters = {}
for k, v in exporters.items():
print(f"run function {k}")
filename = f"plot_torch_dort_{k}.onnx"
torch._dynamo.reset()
model, input_tensor = create_model_and_input()
try:
v(model, input_tensor)
except Exception as e:
print(f"skipped due to {str(e)[:1000]}")
continue
supported_exporters[k] = v
del model
gc.collect()
time.sleep(1)
run function torch_eager
run function torch_default
run function torch_dort
Compile and Memory¶
def flatten(ps):
obs = ps["cpu"].to_dict(unit=2**20)
if "gpus" in ps:
for i, g in enumerate(ps["gpus"]):
for k, v in g.to_dict(unit=2**20).items():
obs[f"gpu{i}_{k}"] = v
return obs
data = []
for k, v in supported_exporters.items():
print(f"run compile for memory {k} on cpu")
filename = f"plot_torch_dort_{k}.onnx"
if has_cuda:
torch.cuda.set_device(0)
torch._dynamo.reset()
# CPU
model, input_tensor = create_model_and_input()
stat = start_spying_on(cuda=1 if has_cuda else 0)
v(model, input_tensor)
obs = flatten(stat.stop())
print("done.")
obs.update(dict(export=k, p="cpu"))
data.append(obs)
del model
gc.collect()
time.sleep(1)
if not has_cuda:
continue
if k in {"torch_default"}:
print(f"skip compile for memory {k} on cuda")
continue
torch._dynamo.reset()
# CUDA
model, input_tensor = create_model_and_input()
model = model.cuda()
input_tensor = input_tensor.cuda()
print(f"run compile for memory {k} on cuda")
stat = start_spying_on(cuda=1 if has_cuda else 0)
v(model, input_tensor)
obs = flatten(stat.stop())
print("done.")
obs.update(dict(export=k, p="cuda"))
data.append(obs)
del model
gc.collect()
time.sleep(1)
run compile for memory torch_eager on cpu
done.
run compile for memory torch_eager on cuda
done.
run compile for memory torch_default on cpu
done.
skip compile for memory torch_default on cuda
run compile for memory torch_dort on cpu
done.
run compile for memory torch_dort on cuda
done.
The result.
df1 = pandas.DataFrame(data)
df1.to_csv("plot_torch_dort_1_memory.csv", index=False)
df1.to_excel("plot_torch_dort_1_memory.xlsx", index=False)
print(df1)
for p in ["cpu", "cuda"]:
if not has_cuda and p == "cuda":
continue
ax = memory_peak_plot(
df1[df1["p"] == p],
key=("export",),
bars=[model_size * i / 2**20 for i in range(1, 5)],
suptitle=f"Memory Consumption of the Compilation on {p}\n"
f"model size={model_size / 2**20:1.0f} Mb",
)
get_figure(ax).savefig(f"plot_torch_dort_1_memory_{p}.png")
peak mean n begin end gpu0_peak gpu0_mean gpu0_n gpu0_begin gpu0_end export p
0 1625.191406 1623.914258 20 1623.210938 1625.191406 535.617188 535.617188 20 535.617188 535.617188 torch_eager cpu
1 1722.074219 1648.712054 49 1621.183594 1722.074219 535.617188 535.617188 49 535.617188 535.617188 torch_eager cuda
2 1727.671875 1725.367053 58 1724.757812 1727.671875 535.617188 535.617188 58 535.617188 535.617188 torch_default cpu
3 1730.277344 1727.735665 112 1727.671875 1730.277344 535.617188 535.617188 112 535.617188 535.617188 torch_dort cpu
4 1780.621094 1732.271099 152 1728.304688 1780.621094 543.617188 536.932977 152 535.617188 543.617188 torch_dort cuda
dort first iteration speed¶
data = []
for k, v in supported_exporters.items():
print(f"run dort cpu {k}: {script_args.repeat1}")
times = []
for _ in range(int(script_args.repeat1)):
model, input_tensor = create_model_and_input()
torch._dynamo.reset()
begin = time.perf_counter()
v(model, input_tensor)
duration = time.perf_counter() - begin
times.append(duration)
del model
gc.collect()
time.sleep(1)
print(f"done: {times[-1]}")
data.append(
dict(
export=k,
time=np.mean(times),
min=min(times),
max=max(times),
first=times[0],
last=times[-1],
std=np.std(times),
p="cpu",
)
)
if not has_cuda:
continue
if k in {"torch_dort", "torch_default"}:
print(f"skip dort cuda {k}: {script_args.repeat1}")
continue
print(f"run dort cuda {k}: {script_args.repeat1}")
times = []
for _ in range(int(script_args.repeat1)):
model, input_tensor = create_model_and_input()
model = model.cuda()
input_tensor = input_tensor.cuda()
torch._dynamo.reset()
begin = time.perf_counter()
v(model, input_tensor)
duration = time.perf_counter() - begin
times.append(duration)
del model
gc.collect()
time.sleep(1)
print(f"done: {times[-1]}")
data.append(
dict(
export=k,
time=np.mean(times),
min=min(times),
max=max(times),
first=times[0],
last=times[-1],
std=np.std(times),
p="cuda",
)
)
run dort cpu torch_eager: 1
done: 0.061221789000228455
run dort cuda torch_eager: 1
done: 0.08717411099951278
run dort cpu torch_default: 1
done: 0.7109125839997432
skip dort cuda torch_default: 1
run dort cpu torch_dort: 1
done: 0.9112194059998728
skip dort cuda torch_dort: 1
The result.
df1 = pandas.DataFrame(data)
df1.to_csv("plot_torch_dort_1_time.csv", index=False)
df1.to_excel("plot_torch_dort_1_time.xlsx", index=False)
print(df1)
fig, ax = plt.subplots(1, 1)
dfi = df1[["export", "p", "time", "std"]].set_index(["export", "p"])
dfi["time"].plot.bar(ax=ax, title="Compilation time", yerr=dfi["std"], rot=30)
fig.tight_layout()
fig.savefig("plot_torch_dort_1_time.png")
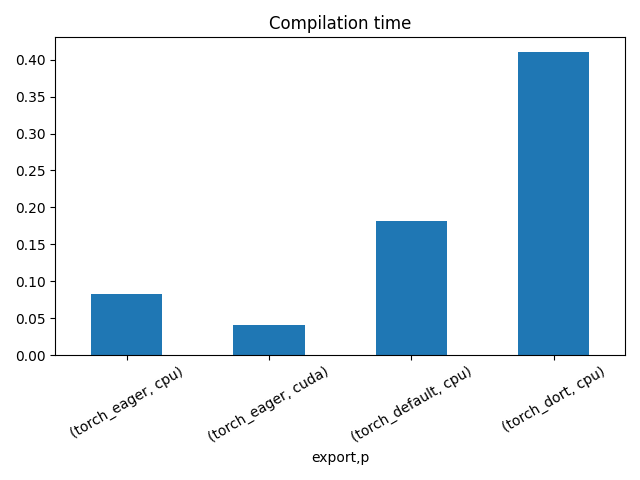
export time min max first last std p
0 torch_eager 0.061222 0.061222 0.061222 0.061222 0.061222 0.0 cpu
1 torch_eager 0.087174 0.087174 0.087174 0.087174 0.087174 0.0 cuda
2 torch_default 0.710913 0.710913 0.710913 0.710913 0.710913 0.0 cpu
3 torch_dort 0.911219 0.911219 0.911219 0.911219 0.911219 0.0 cpu
Benchmark exported models with ORT¶
def benchmark(shape):
data = []
data_mem_first_run = []
data_mem_run = []
confs = list(
itertools.product(
export_functions,
["CPU", "CUDA"],
)
)
loop = tqdm(confs)
print(f"number of experiments: {len(loop)}")
for export_fct, p in loop:
name = export_fct.__name__.replace("get_torch_", "")
obs = {} # system_info()
obs["name"] = name
obs["compute"] = p
obs["export"] = name
model, input_tensor = create_model_and_input()
if p == "CUDA":
if not has_cuda:
continue
model = model.cuda()
input_tensor = input_tensor.cuda()
try:
exported_model = export_fct(model, input_tensor)
except torch._dynamo.exc.BackendCompilerFailed as e:
# Triton only supports devices of CUDA Capability >= 7.0,
# but your device is of CUDA capability 6.1
obs["error"] = str(e)
data.append(obs)
continue
def call_model(
export_fct=export_fct,
exported_model=exported_model,
input_tensor=input_tensor,
):
res = exported_model(input_tensor).sum()
return res
stat = start_spying_on(cuda=1 if has_cuda else 0)
try:
call_model()
except Exception as e:
loop.set_description(f"ERROR-run: {name} {e}")
obs.update({"error": e, "step": "load"})
data.append(obs)
stat.stop()
continue
memobs = flatten(stat.stop())
memobs.update(obs)
data_mem_first_run.append(memobs)
# memory consumption
stat = start_spying_on(cuda=1 if has_cuda else 0)
for _ in range(0, script_args.warmup):
call_model()
memobs = flatten(stat.stop())
memobs.update(obs)
data_mem_run.append(memobs)
obs.update(
measure_time(
call_model,
max_time=script_args.maxtime,
repeat=script_args.repeat,
number=1,
)
)
loop.set_description(f"{obs['average']} {name} {p}")
data.append(obs)
del model
del exported_model
gc.collect()
time.sleep(1)
df = pandas.DataFrame(data)
df.to_csv("plot_torch_dort_ort_time.csv", index=False)
df.to_excel("plot_torch_dort_ort_time.xlsx", index=False)
dfmemr = pandas.DataFrame(data_mem_run)
dfmemr.to_csv("plot_torch_dort_ort_run_mem.csv", index=False)
dfmemr.to_excel("plot_torch_dort_ort_run_mem.xlsx", index=False)
dfmemfr = pandas.DataFrame(data_mem_first_run)
dfmemfr.to_csv("plot_torch_dort_ort_first_run_mem.csv", index=False)
dfmemfr.to_excel("plot_torch_dort_ort_first_run_mem.xlsx", index=False)
return df, dfmemfr, dfmemr
df, dfmemfr, dfmemr = benchmark(list(input_tensor.shape))
print(df)
0%| | 0/6 [00:00<?, ?it/s]number of experiments: 6
0.003072431313704132 eager CPU: 0%| | 0/6 [00:00<?, ?it/s]
0.003072431313704132 eager CPU: 17%|█▋ | 1/6 [00:02<00:11, 2.20s/it]
0.0007839491542784215 eager CUDA: 17%|█▋ | 1/6 [00:02<00:11, 2.20s/it]
0.0007839491542784215 eager CUDA: 33%|███▎ | 2/6 [00:04<00:08, 2.02s/it]
0.02322134633323003 default CPU: 33%|███▎ | 2/6 [00:05<00:08, 2.02s/it]
0.02322134633323003 default CPU: 50%|█████ | 3/6 [00:06<00:07, 2.42s/it]
0.0007147062171055184 default CUDA: 50%|█████ | 3/6 [00:13<00:07, 2.42s/it]
0.0007147062171055184 default CUDA: 67%|██████▋ | 4/6 [00:14<00:09, 4.59s/it]
0.0010182768778681296 dort CPU: 67%|██████▋ | 4/6 [00:16<00:09, 4.59s/it]
0.0010182768778681296 dort CPU: 83%|████████▎ | 5/6 [00:17<00:04, 4.03s/it]
0.0012504478888926607 dort CUDA: 83%|████████▎ | 5/6 [00:19<00:04, 4.03s/it]
0.0012504478888926607 dort CUDA: 100%|██████████| 6/6 [00:21<00:00, 3.76s/it]
0.0012504478888926607 dort CUDA: 100%|██████████| 6/6 [00:21<00:00, 3.53s/it]
name compute export average deviation min_exec max_exec repeat number ttime context_size warmup_time
0 eager CPU eager 0.003072 0.001466 0.001455 0.005237 1 51.0 0.156694 64 0.003151
1 eager CUDA eager 0.000784 0.000100 0.000682 0.000892 1 175.0 0.137191 64 0.001366
2 default CPU default 0.023221 0.010695 0.008224 0.037393 1 6.0 0.139328 64 0.003639
3 default CUDA default 0.000715 0.000257 0.000459 0.001733 1 152.0 0.108635 64 0.002136
4 dort CPU dort 0.001018 0.000071 0.000767 0.001430 1 131.0 0.133394 64 0.001701
5 dort CUDA dort 0.001250 0.000297 0.001061 0.002966 1 81.0 0.101286 64 0.002375
Other view
def view_time(df, title, suffix="time"):
piv = pandas.pivot_table(df, index="export", columns=["compute"], values="average")
print(piv)
piv.to_csv(f"plot_torch_dort_{suffix}_compute.csv")
piv.to_excel(f"plot_torch_dort_{suffix}_compute.xlsx")
piv_cpu = pandas.pivot_table(
df[df.compute == "CPU"],
index="export",
columns=["compute"],
values="average",
)
fig, ax = plt.subplots(1, 2, figsize=(12, 4))
fig.suptitle(title)
piv_cpu.plot.barh(ax=ax[0], title="CPU", logx=True)
if has_cuda:
piv_gpu = pandas.pivot_table(
df[df.compute == "CUDA"],
index="export",
columns=["compute"],
values="average",
)
piv_gpu.plot.barh(ax=ax[1], title="CUDA", logx=True)
fig.tight_layout()
fig.savefig(f"plot_torch_dort_{suffix}.png")
return ax
view_time(df, "Compares processing time on backends")
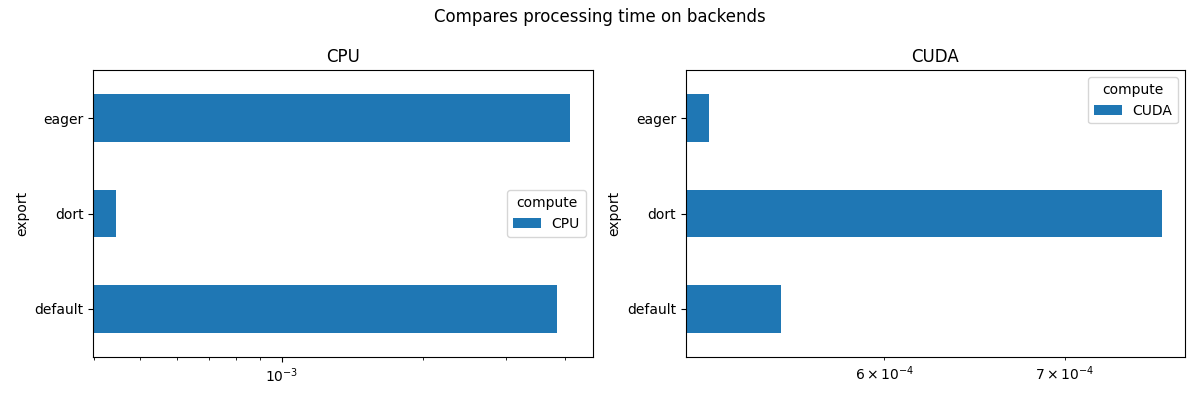
compute CPU CUDA
export
default 0.023221 0.000715
dort 0.001018 0.001250
eager 0.003072 0.000784
array([<Axes: title={'center': 'CPU'}, ylabel='export'>,
<Axes: title={'center': 'CUDA'}, ylabel='export'>], dtype=object)
Memory First Running Time (ORT)¶
for compute in ["CPU", "CUDA"]:
if not has_cuda and compute == "CUDA":
continue
ax = memory_peak_plot(
dfmemfr[dfmemfr.compute == compute],
("export",),
suptitle=f"Memory Consumption of backend, first running time"
f"\nrunning on {compute}",
bars=[model_size * i / 2**20 for i in range(1, 3)],
figsize=(18, 6),
)
get_figure(ax).savefig(f"plot_torch_dort_first_run_mem_{compute}.png")
Memory Running Time (ORT)¶
for compute in ["CPU", "CUDA"]:
if not has_cuda and compute == "CUDA":
continue
ax = memory_peak_plot(
dfmemr[dfmemr.compute == compute],
("export",),
suptitle=f"Memory Consumption of backens, running time\nrunning on {compute}",
bars=[model_size * i / 2**20 for i in range(1, 3)],
figsize=(18, 6),
)
get_figure(ax).savefig(f"plot_torch_dort_run_mem_{compute}.png")
Total running time of the script: (1 minutes 11.561 seconds)
Related examples
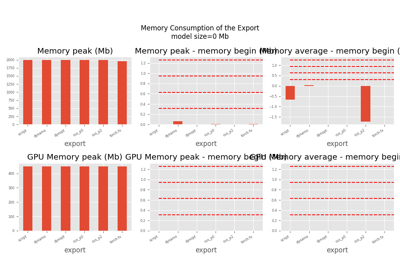
201: Evaluate different ways to export a torch model to ONNX
201: Evaluate different ways to export a torch model to ONNX