Note
Go to the end to download the full example code
Fusing multiplication operators on CUDA¶
The examples compare the performaance of two fused operators Mul with the unfused sequence.
Cache Performance¶
from onnx_extended.args import get_parsed_args
script_args = get_parsed_args(
"plot_op_mul_cuda",
description=__doc__,
config=(
"small",
"small, short optimization (default), "
"medium for medium sizes, "
"large for big sizes",
),
warmup=3,
repeat=5,
itype=(1, "1 or 10 for float or float16"),
expose="config,itype,warmup,repeat",
)
itype = script_args.itype
config = script_args.config
print(f"config={config}")
print(f"itype={itype}")
if config == "small":
sizes = (256, 512, 1024)
elif config == "medium":
sizes = (512, 1024, 2048)
elif config == "large":
sizes = (1024, 2048, 4096, 8192)
else:
try:
sizes = list(map(int, config.split(",")))
except (ValueError, TypeError) as e:
raise AssertionError(f"Unexpected config value {config!r}.") from e
import time
import numpy as np
import onnx.helper as oh
from tqdm import tqdm
from pandas import DataFrame
from onnxruntime import InferenceSession, SessionOptions, get_available_providers
from onnx_array_api.plotting.text_plot import onnx_simple_text_plot
from onnx_extended.ortops.optim.cuda import get_ort_ext_libs
def get_model1(itype):
return oh.make_model(
oh.make_graph(
[
oh.make_node("Mul", ["X", "Y"], ["xy"]),
oh.make_node("Mul", ["xy", "Z"], ["xyz"]),
oh.make_node("Mul", ["Y", "X"], ["yx"]),
oh.make_node("Mul", ["xyz", "yx"], ["final"]),
],
"nd",
[
oh.make_tensor_value_info("X", itype, [None, None]),
oh.make_tensor_value_info("Y", itype, [None, None]),
oh.make_tensor_value_info("Z", itype, [None, None]),
],
[oh.make_tensor_value_info("final", itype, [None, None])],
),
opset_imports=[oh.make_opsetid("", 18)],
ir_version=9,
)
print(onnx_simple_text_plot(get_model1(itype)))
config=small
itype=1
opset: domain='' version=18
input: name='X' type=dtype('float32') shape=['', '']
input: name='Y' type=dtype('float32') shape=['', '']
input: name='Z' type=dtype('float32') shape=['', '']
Mul(X, Y) -> xy
Mul(xy, Z) -> xyz
Mul(Y, X) -> yx
Mul(xyz, yx) -> final
output: name='final' type=dtype('float32') shape=['', '']
And the other model
def get_model2(itype):
return oh.make_model(
oh.make_graph(
[
oh.make_node(
"MulMul",
["X", "Y", "Z"],
["xyz"],
domain="onnx_extended.ortops.optim.cuda",
),
oh.make_node(
"MulMul",
["Y", "X", "xyz"],
["final"],
domain="onnx_extended.ortops.optim.cuda",
),
],
"nd",
[
oh.make_tensor_value_info("X", itype, [None, None]),
oh.make_tensor_value_info("Y", itype, [None, None]),
oh.make_tensor_value_info("Z", itype, [None, None]),
],
[oh.make_tensor_value_info("final", itype, [None, None])],
),
opset_imports=[
oh.make_opsetid("", 18),
oh.make_opsetid("onnx_extended.ortops.optim.cuda", 1),
],
ir_version=9,
)
print(onnx_simple_text_plot(get_model2(itype)))
opset: domain='' version=18
opset: domain='onnx_extended.ortops.optim.cuda' version=1
input: name='X' type=dtype('float32') shape=['', '']
input: name='Y' type=dtype('float32') shape=['', '']
input: name='Z' type=dtype('float32') shape=['', '']
MulMul[onnx_extended.ortops.optim.cuda](X, Y, Z) -> xyz
MulMul[onnx_extended.ortops.optim.cuda](Y, X, xyz) -> final
output: name='final' type=dtype('float32') shape=['', '']
InferenceSession¶
has_cuda = "CUDAExecutionProvider" in get_available_providers()
if has_cuda:
dtype = np.float32 if itype == 1 else np.float16
x = np.random.randn(16, 16).astype(dtype)
y = np.random.randn(16, 16).astype(dtype)
z = np.random.randn(16, 16).astype(dtype)
feeds = dict(X=x, Y=y, Z=z)
sess1 = InferenceSession(
get_model1(itype).SerializeToString(), providers=["CUDAExecutionProvider"]
)
expected = sess1.run(None, feeds)[0]
The other model.
Discrepancies
diff=0.0
Benchmark¶
some code to avoid measuring copying the data from host to device
def move_inputs(sess, feeds):
from onnxruntime.capi._pybind_state import (
SessionIOBinding,
OrtDevice as C_OrtDevice,
OrtValue as C_OrtValue,
)
input_names = [i.name for i in sess.get_inputs()]
ort_device = C_OrtDevice(C_OrtDevice.cuda(), C_OrtDevice.default_memory(), 0)
feed_ort_value = [
(name, C_OrtValue.ortvalue_from_numpy(feeds[name], ort_device))
for name in input_names
]
bind = SessionIOBinding(sess._sess)
for name, value in feed_ort_value:
bind.bind_input(
name, ort_device, feeds[name].dtype, value.shape(), value.data_ptr()
)
for o in sess.get_outputs():
bind.bind_output(o.name, ort_device)
return bind, feed_ort_value
Benchmark function
def benchmark(sess, sizes, label):
data = []
for size in tqdm(sizes):
x = np.random.randn(size, size).astype(dtype)
y = np.random.randn(size, size).astype(dtype)
z = np.random.randn(size, size).astype(dtype)
feeds = dict(X=x, Y=y, Z=z)
bind, cuda_feeds = move_inputs(sess, feeds)
begin = time.perf_counter()
for i in range(script_args.warmup):
# sess.run(None, feeds)
sess._sess.run_with_iobinding(bind, None)
warmup = time.perf_counter() - begin
times = []
for i in range(script_args.repeat):
begin = time.perf_counter()
# sess.run(None, feeds)
sess._sess.run_with_iobinding(bind, None)
times.append(time.perf_counter() - begin)
npt = np.array(times)
obs = dict(
warmup=warmup,
time=npt.mean(),
std=npt.std(),
min=npt.min(),
max=npt.max(),
repeat=script_args.repeat,
size=size,
label=label,
)
data.append(obs)
return data
Not Fused.
sizes=(256, 512, 1024)
0%| | 0/3 [00:00<?, ?it/s]
100%|██████████| 3/3 [00:00<00:00, 12.00it/s]
100%|██████████| 3/3 [00:00<00:00, 11.98it/s]
Fused.
if has_cuda:
data_mulmul = benchmark(sess2, sizes, "Fused")
0%| | 0/3 [00:00<?, ?it/s]
100%|██████████| 3/3 [00:00<00:00, 18.85it/s]
100%|██████████| 3/3 [00:00<00:00, 18.81it/s]
Data¶
warmup time std min max repeat size label
0 0.001041 0.000263 0.000037 0.000231 0.000331 5 256 Not Fused
1 0.006591 0.001112 0.000071 0.000994 0.001203 5 512 Not Fused
2 0.013479 0.003823 0.000384 0.003556 0.004579 5 1024 Not Fused
3 0.001064 0.000250 0.000064 0.000195 0.000364 5 256 Fused
4 0.002817 0.000724 0.000097 0.000646 0.000894 5 512 Fused
Pivot.
if has_cuda:
pivot = df.pivot(index="size", columns="label", values="time")
pivot["ratio"] = pivot["Fused"] / pivot["Not Fused"]
print(pivot)
ax = pivot[["Not Fused", "Fused"]].plot(
logx=True,
logy=True,
title=f"Fused/Unfused element wise multiplication on CUDA\nitype={itype}",
)
ax.get_figure().savefig("plot_op_mul_cuda.png")
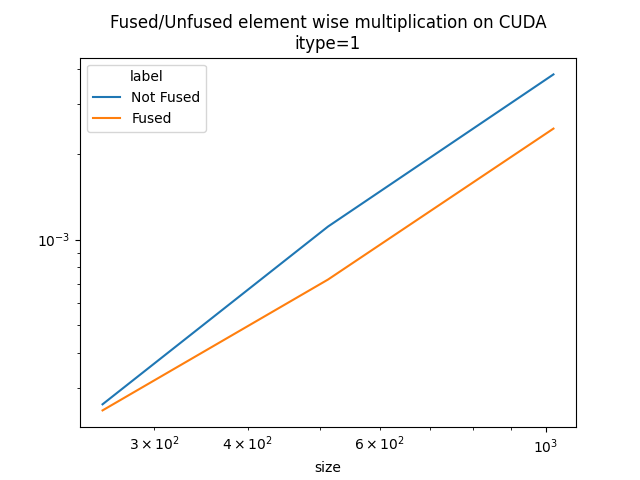
label Fused Not Fused ratio
size
256 0.000250 0.000263 0.951791
512 0.000724 0.001112 0.650576
1024 0.002463 0.003823 0.644207
It seems the fused operator is 33% faster.
Total running time of the script: (0 minutes 15.456 seconds)