Note
Go to the end to download the full example code.
Quantile Regression¶
scikit-learn does not have a quantile regression. mlinsights implements a version of it.
Simple example¶
We first generate some dummy data.
import numpy
import matplotlib.pyplot as plt
from pandas import DataFrame
from sklearn.linear_model import LinearRegression
from mlinsights.mlmodel import QuantileLinearRegression
X = numpy.random.random(1000)
eps1 = (numpy.random.random(900) - 0.5) * 0.1
eps2 = (numpy.random.random(100)) * 10
eps = numpy.hstack([eps1, eps2])
X = X.reshape((1000, 1))
Y = X.ravel() * 3.4 + 5.6 + eps
clr = LinearRegression()
clr.fit(X, Y)
fig, ax = plt.subplots(1, 1, figsize=(10, 4))
choice = numpy.random.choice(X.shape[0] - 1, size=100)
xx = X.ravel()[choice]
yy = Y[choice]
ax.plot(xx, yy, ".", label="data")
xx = numpy.array([[0], [1]])
y1 = clr.predict(xx)
y2 = clq.predict(xx)
ax.plot(xx, y1, "--", label="L2")
ax.plot(xx, y2, "--", label="L1")
ax.set_title("Quantile (L1) vs Square (L2)")
ax.legend()
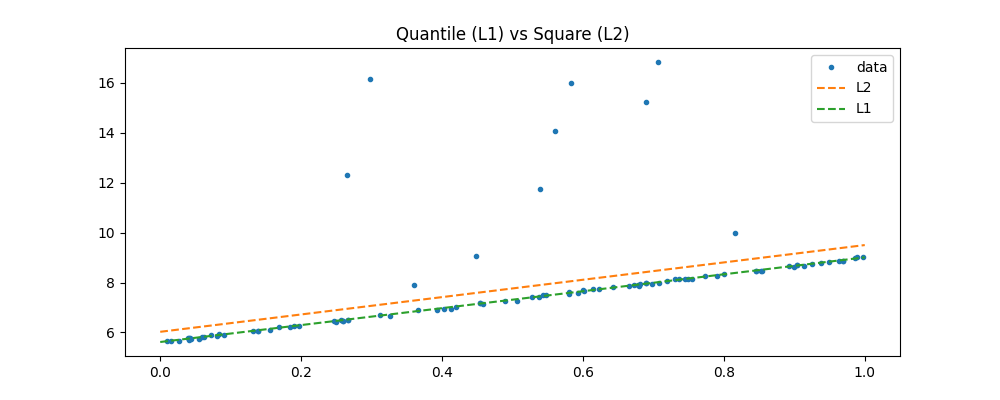
<matplotlib.legend.Legend object at 0x7f7557173820>
The L1 is clearly less sensible to extremas. The optimization algorithm is based on Iteratively reweighted least squares. It estimates a linear regression with error L2 then reweights each oberservation with the inverse of the error L1.
clq = QuantileLinearRegression(verbose=True, max_iter=20)
clq.fit(X, Y)
[QuantileLinearRegression.fit] iter=1 error=887.3974799042942
[QuantileLinearRegression.fit] iter=2 error=584.418315445887
[QuantileLinearRegression.fit] iter=3 error=515.6852922462667
[QuantileLinearRegression.fit] iter=4 error=515.2680053173664
[QuantileLinearRegression.fit] iter=5 error=514.9246380365536
[QuantileLinearRegression.fit] iter=6 error=514.5965386047486
[QuantileLinearRegression.fit] iter=7 error=514.3901850422749
[QuantileLinearRegression.fit] iter=8 error=514.2057153574946
[QuantileLinearRegression.fit] iter=9 error=514.0821374889089
[QuantileLinearRegression.fit] iter=10 error=513.9987881952429
[QuantileLinearRegression.fit] iter=11 error=513.9358925448369
[QuantileLinearRegression.fit] iter=12 error=513.890761901876
[QuantileLinearRegression.fit] iter=13 error=513.852366694661
[QuantileLinearRegression.fit] iter=14 error=513.8206482671766
[QuantileLinearRegression.fit] iter=15 error=513.8016564994344
[QuantileLinearRegression.fit] iter=16 error=513.7883817254528
[QuantileLinearRegression.fit] iter=17 error=513.778283428184
[QuantileLinearRegression.fit] iter=18 error=513.7700595409715
[QuantileLinearRegression.fit] iter=19 error=513.7648371043631
[QuantileLinearRegression.fit] iter=20 error=513.7603803736176
0.5137603803736176
Regression with various quantiles¶
X = numpy.random.random(1200)
eps1 = (numpy.random.random(900) - 0.5) * 0.5
eps2 = (numpy.random.random(300)) * 2
eps = numpy.hstack([eps1, eps2])
X = X.reshape((1200, 1))
Y = X.ravel() * 3.4 + 5.6 + eps + X.ravel() * X.ravel() * 8
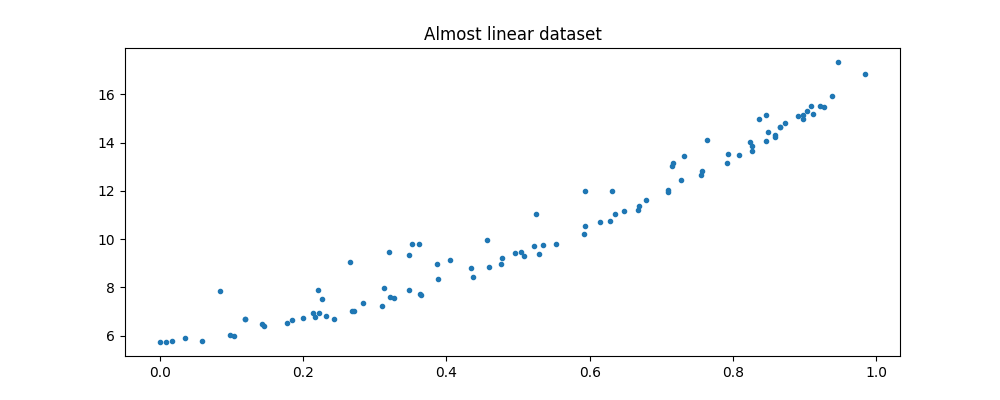
Text(0.5, 1.0, 'Almost linear dataset')
fig, ax = plt.subplots(1, 1, figsize=(10, 4))
choice = numpy.random.choice(X.shape[0] - 1, size=100)
xx = X.ravel()[choice]
yy = Y[choice]
ax.plot(xx, yy, ".", label="data")
xx = numpy.array([[0], [1]])
for qu in sorted(clqs):
y = clqs[qu].predict(xx)
ax.plot(xx, y, "--", label=qu)
ax.set_title("Various quantiles")
ax.legend()
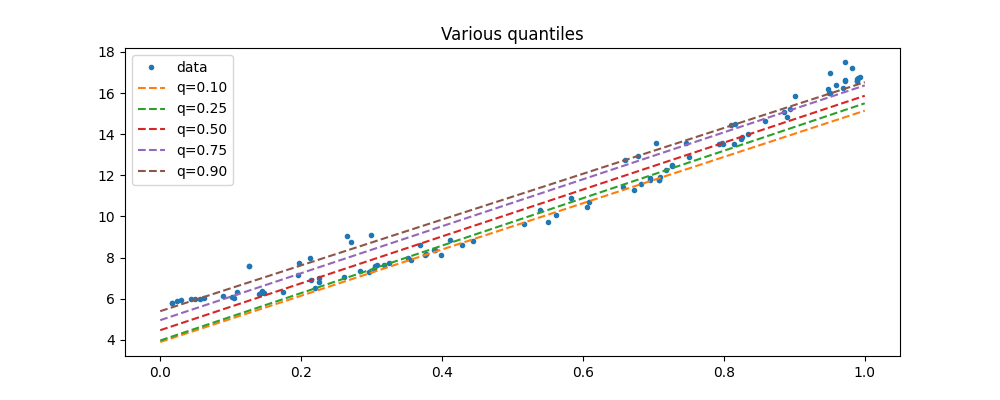
<matplotlib.legend.Legend object at 0x7f7556241180>
Total running time of the script: (0 minutes 0.210 seconds)