Note
Go to the end to download the full example code.
LogisticRegression and Clustering¶
A logistic regression implements a convex partition of the features spaces. A clustering algorithm applied before the trainer modifies the feature space in way the partition is not necessarily convex in the initial features. Let’s see how.
A dummy datasets and not convex¶
import numpy
import pandas
import matplotlib.pyplot as plt
from sklearn.linear_model import LogisticRegression
from sklearn.ensemble import RandomForestClassifier
from mlinsights.mlmodel import ClassifierAfterKMeans
Xs = []
Ys = []
n = 20
for i in range(5):
for j in range(4):
x1 = numpy.random.rand(n) + i * 1.1
x2 = numpy.random.rand(n) + j * 1.1
Xs.append(numpy.vstack([x1, x2]).T)
cl = numpy.random.randint(0, 4)
Ys.extend([cl for i in range(n)])
X = numpy.vstack(Xs)
Y = numpy.array(Ys)
X.shape, Y.shape, set(Y)
((400, 2), (400,), {np.int64(0), np.int64(1), np.int64(2), np.int64(3)})
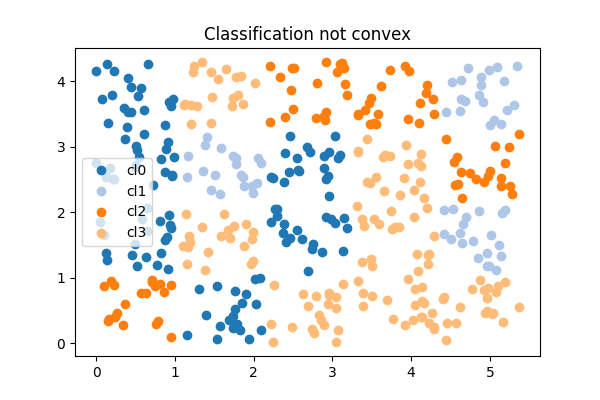
Text(0.5, 1.0, 'Classification not convex')
One function to plot classification in 2D¶
def draw_border(
clr,
X,
y,
fct=None,
incx=1,
incy=1,
figsize=None,
border=True,
clusters=None,
ax=None,
):
# see https://sashat.me/2017/01/11/list-of-20-simple-distinct-colors/
# https://matplotlib.org/examples/color/colormaps_reference.html
h = 0.02 # step size in the mesh
# Plot the decision boundary. For that, we will assign a color to each
# point in the mesh [x_min, x_max]x[y_min, y_max].
x_min, x_max = X[:, 0].min() - incx, X[:, 0].max() + incx
y_min, y_max = X[:, 1].min() - incy, X[:, 1].max() + incy
xx, yy = numpy.meshgrid(
numpy.arange(x_min, x_max, h), numpy.arange(y_min, y_max, h)
)
if fct is None:
Z = clr.predict(numpy.c_[xx.ravel(), yy.ravel()])
else:
Z = fct(clr, numpy.c_[xx.ravel(), yy.ravel()])
# Put the result into a color plot
cmap = plt.cm.tab20
Z = Z.reshape(xx.shape)
if ax is None:
fig, ax = plt.subplots(1, 1, figsize=figsize or (4, 3))
ax.pcolormesh(xx, yy, Z, cmap=cmap)
# Plot also the training points
ax.scatter(X[:, 0], X[:, 1], c=y, edgecolors="k", cmap=cmap)
ax.set_xlabel("Sepal length")
ax.set_ylabel("Sepal width")
ax.set_xlim(xx.min(), xx.max())
ax.set_ylim(yy.min(), yy.max())
# Plot clusters
if clusters is not None:
mat = []
ym = []
for k, v in clusters.items():
mat.append(v.cluster_centers_)
ym.extend(k for i in range(v.cluster_centers_.shape[0]))
cx = numpy.vstack(mat)
ym = numpy.array(ym)
ax.scatter(cx[:, 0], cx[:, 1], c=ym, edgecolors="y", cmap=cmap, s=300)
return ax
Logistic Regression¶
clr = LogisticRegression(solver="lbfgs", multi_class="multinomial")
clr.fit(X, Y)
/home/xadupre/github/scikit-learn/sklearn/linear_model/_logistic.py:1242: FutureWarning: 'multi_class' was deprecated in version 1.5 and will be removed in 1.7. From then on, it will always use 'multinomial'. Leave it to its default value to avoid this warning.
warnings.warn(
ax = draw_border(clr, X, Y, incx=1, incy=1, figsize=(6, 4), border=False)
ax.set_title("Logistic Regression")
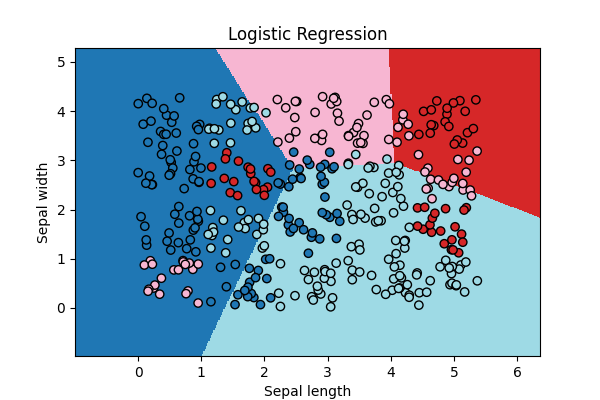
Text(0.5, 1.0, 'Logistic Regression')
Not quite close!
Logistic Regression and k-means¶
clk = ClassifierAfterKMeans(e_solver="lbfgs", e_multi_class="multinomial")
clk.fit(X, Y)
/home/xadupre/github/scikit-learn/sklearn/linear_model/_logistic.py:1242: FutureWarning: 'multi_class' was deprecated in version 1.5 and will be removed in 1.7. From then on, it will always use 'multinomial'. Leave it to its default value to avoid this warning.
warnings.warn(
/home/xadupre/github/scikit-learn/sklearn/linear_model/_logistic.py:464: ConvergenceWarning: lbfgs failed to converge (status=1):
STOP: TOTAL NO. of ITERATIONS REACHED LIMIT.
Increase the number of iterations (max_iter) or scale the data as shown in:
https://scikit-learn.org/stable/modules/preprocessing.html
Please also refer to the documentation for alternative solver options:
https://scikit-learn.org/stable/modules/linear_model.html#logistic-regression
n_iter_i = _check_optimize_result(
The centers of the first k-means:
clk.clus_[0].cluster_centers_
array([[0.54550994, 2.73039463],
[2.34472491, 1.6361495 ]])
ax = draw_border(
clk, X, Y, incx=1, incy=1, figsize=(6, 4), border=False, clusters=clk.clus_
)
ax.set_title("Logistic Regression and K-Means - 2 clusters per class")
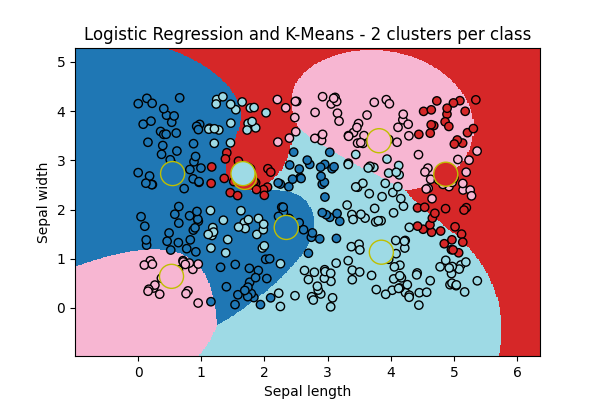
Text(0.5, 1.0, 'Logistic Regression and K-Means - 2 clusters per class')
The big cricles are the centers of the k-means fitted for each class. It look better!
Variation¶
/home/xadupre/github/scikit-learn/sklearn/linear_model/_logistic.py:1242: FutureWarning: 'multi_class' was deprecated in version 1.5 and will be removed in 1.7. From then on, it will always use 'multinomial'. Leave it to its default value to avoid this warning.
warnings.warn(
/home/xadupre/github/scikit-learn/sklearn/linear_model/_logistic.py:1242: FutureWarning: 'multi_class' was deprecated in version 1.5 and will be removed in 1.7. From then on, it will always use 'multinomial'. Leave it to its default value to avoid this warning.
warnings.warn(
/home/xadupre/github/scikit-learn/sklearn/linear_model/_logistic.py:1242: FutureWarning: 'multi_class' was deprecated in version 1.5 and will be removed in 1.7. From then on, it will always use 'multinomial'. Leave it to its default value to avoid this warning.
warnings.warn(
/home/xadupre/github/scikit-learn/sklearn/linear_model/_logistic.py:1242: FutureWarning: 'multi_class' was deprecated in version 1.5 and will be removed in 1.7. From then on, it will always use 'multinomial'. Leave it to its default value to avoid this warning.
warnings.warn(
/home/xadupre/github/scikit-learn/sklearn/linear_model/_logistic.py:1242: FutureWarning: 'multi_class' was deprecated in version 1.5 and will be removed in 1.7. From then on, it will always use 'multinomial'. Leave it to its default value to avoid this warning.
warnings.warn(
ax = draw_border(
clk, X, Y, incx=1, incy=1, figsize=(6, 4), border=False, clusters=clk.clus_
)
ax.set_title("Logistic Regression and K-Means - 8 clusters per class")
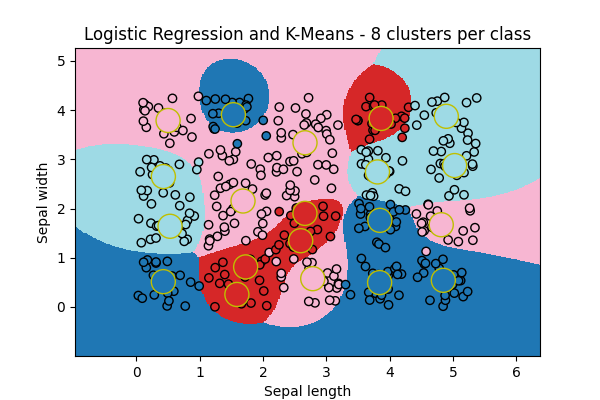
Text(0.5, 1.0, 'Logistic Regression and K-Means - 8 clusters per class')
Random Forest¶
# The random forest works without any clustering as expected.
rf = RandomForestClassifier(n_estimators=20)
rf.fit(X, Y)
ax = draw_border(rf, X, Y, incx=1, incy=1, figsize=(6, 4), border=False)
ax.set_title("Random Forest")
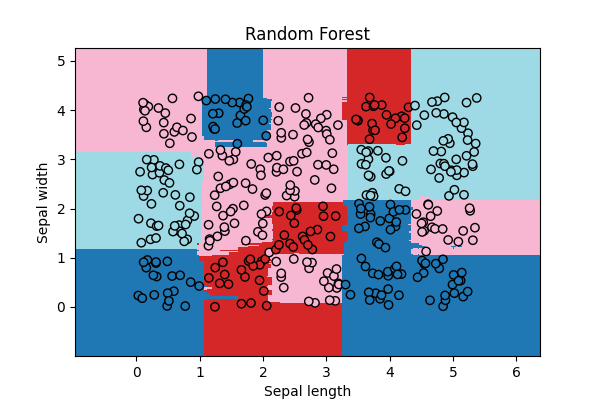
Text(0.5, 1.0, 'Random Forest')
Total running time of the script: (0 minutes 1.093 seconds)