Note
Go to the end to download the full example code
Quantile Regression#
scikit-learn does not have a quantile regression. mlinsights implements a version of it.
Simple example#
We first generate some dummy data.
import numpy
import matplotlib.pyplot as plt
from pandas import DataFrame
from sklearn.linear_model import LinearRegression
from mlinsights.mlmodel import QuantileLinearRegression
X = numpy.random.random(1000)
eps1 = (numpy.random.random(900) - 0.5) * 0.1
eps2 = (numpy.random.random(100)) * 10
eps = numpy.hstack([eps1, eps2])
X = X.reshape((1000, 1))
Y = X.ravel() * 3.4 + 5.6 + eps
clr = LinearRegression()
clr.fit(X, Y)
fig, ax = plt.subplots(1, 1, figsize=(10, 4))
choice = numpy.random.choice(X.shape[0] - 1, size=100)
xx = X.ravel()[choice]
yy = Y[choice]
ax.plot(xx, yy, ".", label="data")
xx = numpy.array([[0], [1]])
y1 = clr.predict(xx)
y2 = clq.predict(xx)
ax.plot(xx, y1, "--", label="L2")
ax.plot(xx, y2, "--", label="L1")
ax.set_title("Quantile (L1) vs Square (L2)")
ax.legend()
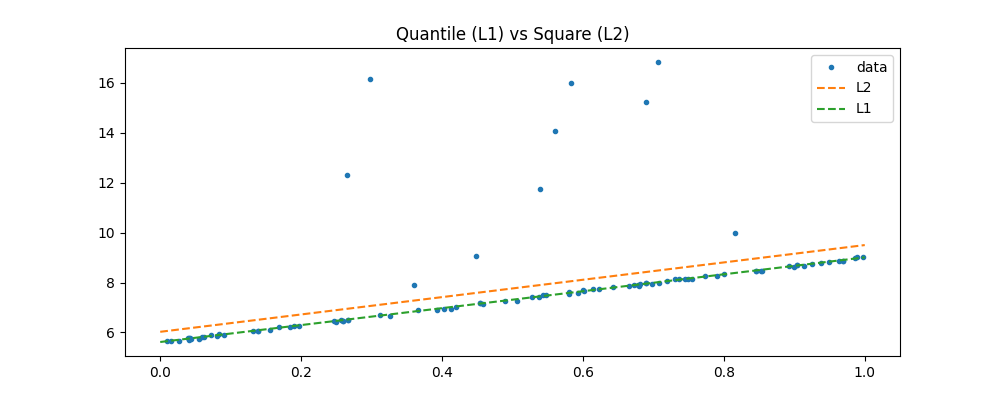
<matplotlib.legend.Legend object at 0x7fee76db9300>
The L1 is clearly less sensible to extremas. The optimization algorithm is based on Iteratively reweighted least squares. It estimates a linear regression with error L2 then reweights each oberservation with the inverse of the error L1.
clq = QuantileLinearRegression(verbose=True, max_iter=20)
clq.fit(X, Y)
[QuantileLinearRegression.fit] iter=1 error=835.7433085753823
[QuantileLinearRegression.fit] iter=2 error=541.6462544523913
[QuantileLinearRegression.fit] iter=3 error=490.12149584807094
[QuantileLinearRegression.fit] iter=4 error=488.38545568231723
[QuantileLinearRegression.fit] iter=5 error=487.73403374126303
[QuantileLinearRegression.fit] iter=6 error=487.2399890513258
[QuantileLinearRegression.fit] iter=7 error=486.87120486499697
[QuantileLinearRegression.fit] iter=8 error=486.56688076275424
[QuantileLinearRegression.fit] iter=9 error=486.1633998393787
[QuantileLinearRegression.fit] iter=10 error=485.9433740268136
[QuantileLinearRegression.fit] iter=11 error=485.82860372441843
[QuantileLinearRegression.fit] iter=12 error=485.76584592891413
[QuantileLinearRegression.fit] iter=13 error=485.7262314864904
[QuantileLinearRegression.fit] iter=14 error=485.6949812700002
[QuantileLinearRegression.fit] iter=15 error=485.66430562971664
[QuantileLinearRegression.fit] iter=16 error=485.6410981708784
[QuantileLinearRegression.fit] iter=17 error=485.6283769087981
[QuantileLinearRegression.fit] iter=18 error=485.61807358943935
[QuantileLinearRegression.fit] iter=19 error=485.6059412673913
[QuantileLinearRegression.fit] iter=20 error=485.5963091132484
0.48559630911324847
Regression with various quantiles#
X = numpy.random.random(1200)
eps1 = (numpy.random.random(900) - 0.5) * 0.5
eps2 = (numpy.random.random(300)) * 2
eps = numpy.hstack([eps1, eps2])
X = X.reshape((1200, 1))
Y = X.ravel() * 3.4 + 5.6 + eps + X.ravel() * X.ravel() * 8
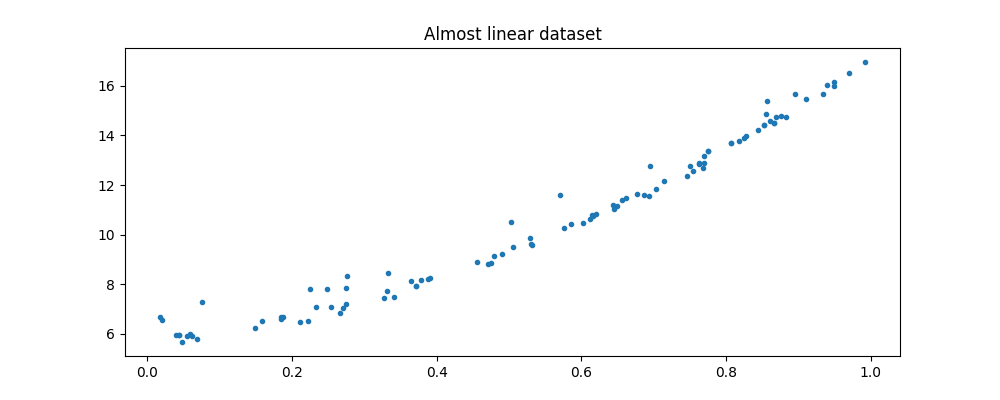
Text(0.5, 1.0, 'Almost linear dataset')
fig, ax = plt.subplots(1, 1, figsize=(10, 4))
choice = numpy.random.choice(X.shape[0] - 1, size=100)
xx = X.ravel()[choice]
yy = Y[choice]
ax.plot(xx, yy, ".", label="data")
xx = numpy.array([[0], [1]])
for qu in sorted(clqs):
y = clqs[qu].predict(xx)
ax.plot(xx, y, "--", label=qu)
ax.set_title("Various quantiles")
ax.legend()
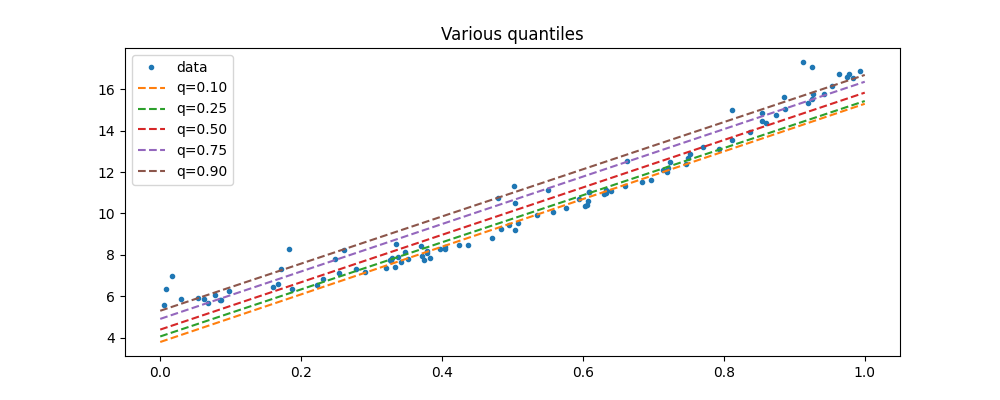
<matplotlib.legend.Legend object at 0x7feea84352d0>
Total running time of the script: (0 minutes 0.382 seconds)