Note
Go to the end to download the full example code
Close leaves in a decision trees#
A decision tree computes a partition of the feature space. We can wonder which leave is close to another one even though the predict the same value (or class). Do they share a border?
A simple tree#
import matplotlib.pyplot as plt
import numpy
from mlinsights.mltree import predict_leaves, tree_leave_index, tree_leave_neighbors
from sklearn.datasets import load_iris
from sklearn.tree import DecisionTreeClassifier
X = numpy.array(
[[10, 0], [10, 1], [10, 2], [11, 0], [11, 1], [11, 2], [12, 0], [12, 1], [12, 2]]
)
y = list(range(X.shape[0]))
# In[5]:
fig, ax = plt.subplots(1, 1)
for i in range(X.shape[0]):
ax.plot([X[i, 0]], [X[i, 1]], "o", ms=19, label="y=%d" % y[i])
ax.legend()
ax.set_title("Simple grid")
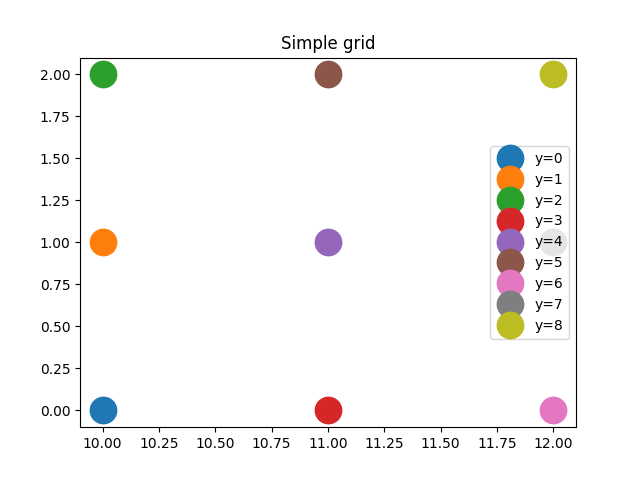
Text(0.5, 1.0, 'Simple grid')
clr = DecisionTreeClassifier(max_depth=5)
clr.fit(X, y)
The contains the following list of leaves.
tree_leave_index(clr)
[2, 4, 5, 8, 9, 12, 13, 15, 16]
Let’s compute the neighbors for each leave.
{(2, 8): [(0, (10.0, 0.0), (11.0, 0.0))], (2, 4): [(1, (10.0, 0.0), (10.0, 1.0))], (4, 12): [(0, (10.0, 1.0), (11.0, 1.0))], (4, 5): [(1, (10.0, 1.0), (10.0, 2.0))], (5, 13): [(0, (10.0, 2.0), (11.0, 2.0))], (8, 9): [(0, (11.0, 0.0), (12.0, 0.0))], (8, 12): [(1, (11.0, 0.0), (11.0, 1.0))], (12, 15): [(0, (11.0, 1.0), (12.0, 1.0))], (12, 13): [(1, (11.0, 1.0), (11.0, 2.0))], (13, 16): [(0, (11.0, 2.0), (12.0, 2.0))], (9, 15): [(1, (12.0, 0.0), (12.0, 1.0))], (15, 16): [(1, (12.0, 1.0), (12.0, 2.0))]}
And let’s explain the results by drawing the segments [x1, x2]
.
leaves = predict_leaves(clr, X)
fig, ax = plt.subplots(1, 2, figsize=(14, 4))
for i in range(X.shape[0]):
ax[0].plot([X[i, 0]], [X[i, 1]], "o", ms=19)
ax[1].plot([X[i, 0]], [X[i, 1]], "o", ms=19)
ax[0].text(X[i, 0] + 0.1, X[i, 1] - 0.1, "y=%d\nl=%d" % (y[i], leaves[i]))
for edge, segments in neighbors.items():
for segment in segments:
# leaves l1, l2 are neighbors
l1, l2 = edge
# the common border is [x1, x2]
x1 = segment[1]
x2 = segment[2]
ax[1].plot([x1[0], x2[0]], [x1[1], x2[1]], "b--")
ax[1].text((x1[0] + x2[0]) / 2, (x1[1] + x2[1]) / 2, "%d->%d" % edge)
ax[0].set_title("Classes and leaves")
ax[1].set_title("Segments")
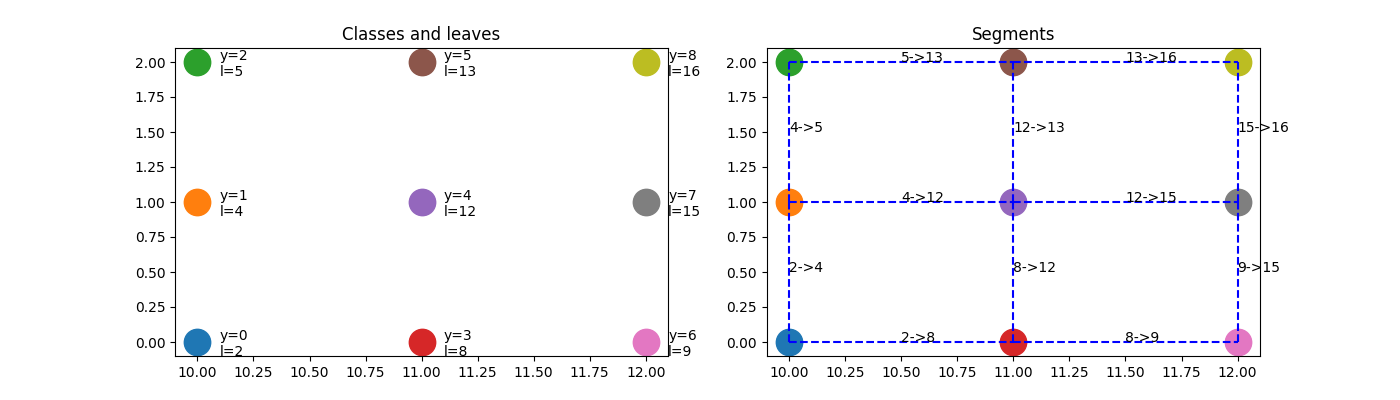
Text(0.5, 1.0, 'Segments')
On Iris#
X = iris.data[:, :2]
y = iris.target
clr = DecisionTreeClassifier(max_depth=3)
clr.fit(X, y)
def draw_border(
clr, X, y, fct=None, incx=1, incy=1, figsize=None, border=True, ax=None
):
# see https://sashat.me/2017/01/11/list-of-20-simple-distinct-colors/
# https://matplotlib.org/examples/color/colormaps_reference.html
h = 0.02 # step size in the mesh
# Plot the decision boundary. For that, we will assign a color to each
# point in the mesh [x_min, x_max]x[y_min, y_max].
x_min, x_max = X[:, 0].min() - incx, X[:, 0].max() + incx
y_min, y_max = X[:, 1].min() - incy, X[:, 1].max() + incy
xx, yy = numpy.meshgrid(
numpy.arange(x_min, x_max, h), numpy.arange(y_min, y_max, h)
)
if fct is None:
Z = clr.predict(numpy.c_[xx.ravel(), yy.ravel()])
else:
Z = fct(clr, numpy.c_[xx.ravel(), yy.ravel()])
# Put the result into a color plot
cmap = plt.cm.tab20
Z = Z.reshape(xx.shape)
if ax is None:
fig, ax = plt.subplots(1, 1, figsize=figsize or (4, 3))
ax.pcolormesh(xx, yy, Z, cmap=cmap)
# Plot also the training points
ax.scatter(X[:, 0], X[:, 1], c=y, edgecolors="k", cmap=cmap)
ax.set_xlabel("Sepal length")
ax.set_ylabel("Sepal width")
ax.set_xlim(xx.min(), xx.max())
ax.set_ylim(yy.min(), yy.max())
return ax
fig, ax = plt.subplots(1, 2, figsize=(14, 4))
draw_border(clr, X, y, border=False, ax=ax[0])
ax[0].set_title("Iris")
draw_border(clr, X, y, border=False, ax=ax[1], fct=lambda m, x: predict_leaves(m, x))
ax[1].set_title("Leaves")
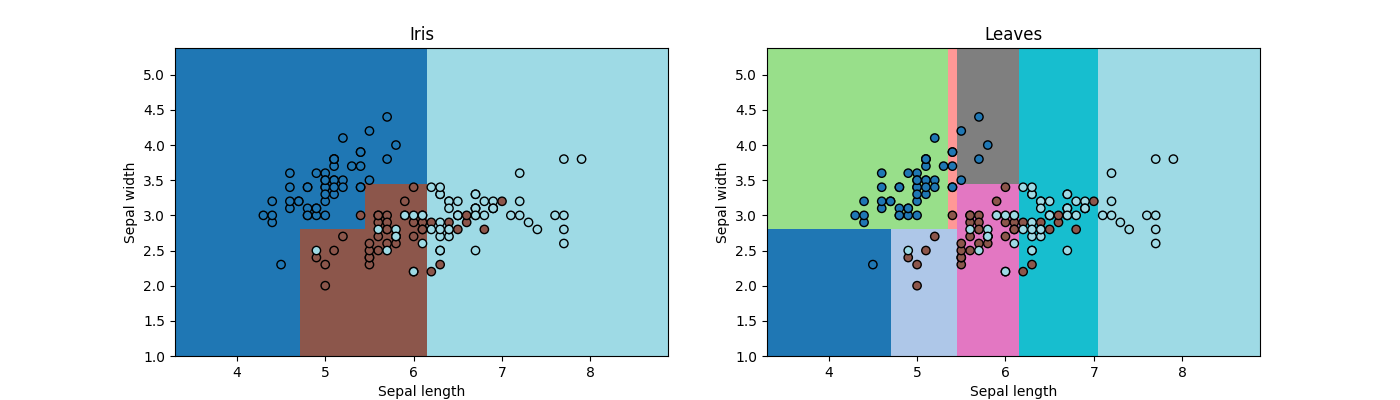
Text(0.5, 1.0, 'Leaves')
[((3, 4), [(0, (4.650000095367432, 2.4750000834465027), (5.025000095367432, 2.4750000834465027))]), ((3, 6), [(1, (4.650000095367432, 2.4750000834465027), (4.650000095367432, 3.1250000596046448))])]
fig, ax = plt.subplots(1, 1, figsize=(8, 8))
draw_border(
clr,
X,
y,
incx=1,
incy=1,
figsize=(6, 4),
border=False,
ax=ax,
fct=lambda m, x: predict_leaves(m, x),
)
for edge, segments in neighbors.items():
for segment in segments:
# leaves l1, l2 are neighbors
l1, l2 = edge
# the common border is [x1, x2]
x1 = segment[1]
x2 = segment[2]
ax.plot([x1[0], x2[0]], [x1[1], x2[1]], "b--")
ax.text((x1[0] + x2[0]) / 2, (x1[1] + x2[1]) / 2, "%d->%d" % edge)
ax.set_title("Leaves and segments")
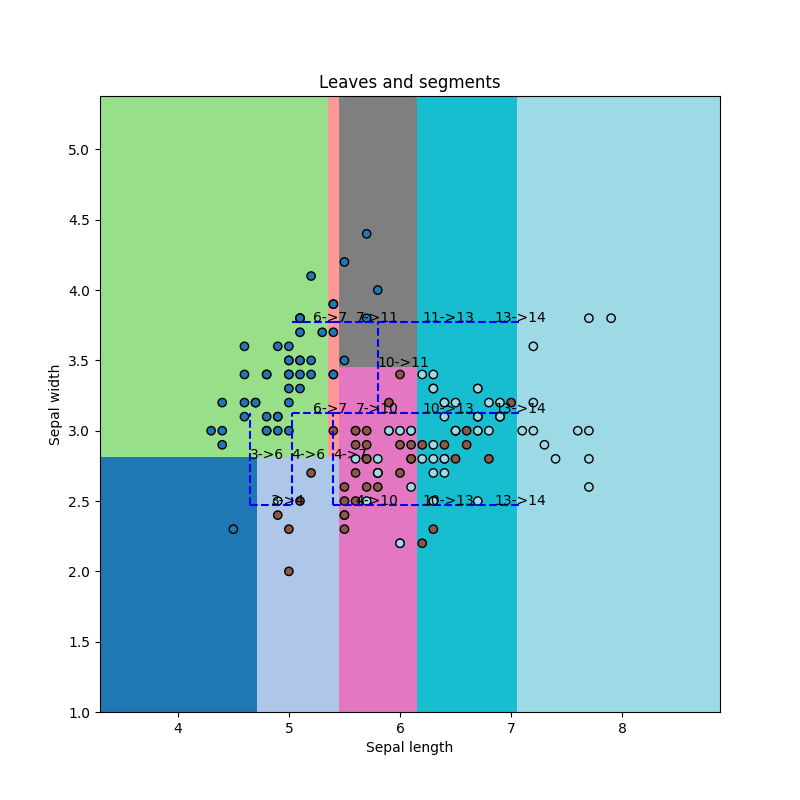
Text(0.5, 1.0, 'Leaves and segments')
Total running time of the script: (0 minutes 1.237 seconds)