Note
Go to the end to download the full example code
Measuring CPU performance with a parallelized vector sum#
The example compares the time spend in computing the sum of all coefficients of a matrix when the function walks through the coefficients by rows or by columns when the computation is parallelized.
Vector Sum#
from tqdm import tqdm
import numpy
import matplotlib.pyplot as plt
from pandas import DataFrame
from onnx_extended.ext_test_case import measure_time, unit_test_going
from onnx_extended.validation.cpu._validation import (
vector_sum_array as vector_sum,
vector_sum_array_parallel as vector_sum_parallel,
)
obs = []
dims = [500, 700, 800, 900, 1000, 1100, 1200, 1300, 1400, 1500, 1600, 1700, 1800, 2000]
if unit_test_going():
dims = dims[:2]
for dim in tqdm(dims):
values = numpy.ones((dim, dim), dtype=numpy.float32).ravel()
diff = abs(vector_sum(dim, values, True) - dim**2)
res = measure_time(lambda: vector_sum(dim, values, True), max_time=0.5)
obs.append(
dict(
dim=dim,
size=values.size,
time=res["average"],
direction="rows",
time_per_element=res["average"] / dim**2,
diff=diff,
)
)
res = measure_time(lambda: vector_sum_parallel(dim, values, True), max_time=0.5)
obs.append(
dict(
dim=dim,
size=values.size,
time=res["average"],
direction="rows//",
time_per_element=res["average"] / dim**2,
diff=diff,
)
)
diff = abs(vector_sum(dim, values, False) - dim**2)
res = measure_time(lambda: vector_sum_parallel(dim, values, False), max_time=0.5)
obs.append(
dict(
dim=dim,
size=values.size,
time=res["average"],
direction="cols//",
time_per_element=res["average"] / dim**2,
diff=diff,
)
)
df = DataFrame(obs)
piv = df.pivot(index="dim", columns="direction", values="time_per_element")
print(piv)
0%| | 0/14 [00:00<?, ?it/s]
7%|7 | 1/14 [00:01<00:22, 1.73s/it]
14%|#4 | 2/14 [00:03<00:20, 1.72s/it]
21%|##1 | 3/14 [00:05<00:22, 2.01s/it]
29%|##8 | 4/14 [00:07<00:18, 1.89s/it]
36%|###5 | 5/14 [00:09<00:16, 1.87s/it]
43%|####2 | 6/14 [00:11<00:15, 1.89s/it]
50%|##### | 7/14 [00:13<00:12, 1.85s/it]
57%|#####7 | 8/14 [00:14<00:11, 1.84s/it]
64%|######4 | 9/14 [00:16<00:08, 1.78s/it]
71%|#######1 | 10/14 [00:18<00:07, 1.75s/it]
79%|#######8 | 11/14 [00:19<00:05, 1.73s/it]
86%|########5 | 12/14 [00:21<00:03, 1.72s/it]
93%|#########2| 13/14 [00:23<00:01, 1.72s/it]
100%|##########| 14/14 [00:25<00:00, 1.72s/it]
100%|##########| 14/14 [00:25<00:00, 1.79s/it]
direction cols// rows rows//
dim
500 4.097443e-10 1.049476e-09 2.827302e-10
700 3.478543e-10 1.047094e-09 2.688798e-10
800 4.044373e-10 1.049253e-09 7.343808e-10
900 3.783477e-10 1.044797e-09 2.676229e-10
1000 1.194984e-09 1.051420e-09 2.672745e-10
1100 1.822083e-09 1.069530e-09 2.943174e-10
1200 1.728993e-09 1.065641e-09 2.699241e-10
1300 1.936429e-09 1.077499e-09 3.466560e-10
1400 1.768032e-09 1.082127e-09 2.844029e-10
1500 2.001714e-09 1.092231e-09 2.857570e-10
1600 1.872902e-09 1.108881e-09 2.757862e-10
1700 1.936484e-09 1.106547e-09 2.789415e-10
1800 1.864029e-09 1.125567e-09 2.766655e-10
2000 2.013057e-09 1.103585e-09 2.933698e-10
Plots#
piv_diff = df.pivot(index="dim", columns="direction", values="diff")
piv_time = df.pivot(index="dim", columns="direction", values="time")
fig, ax = plt.subplots(1, 3, figsize=(12, 6))
piv.plot(ax=ax[0], logx=True, title="Comparison between two summation")
piv_diff.plot(ax=ax[1], logx=True, logy=True, title="Summation errors")
piv_time.plot(ax=ax[2], logx=True, logy=True, title="Total time")
fig.savefig("plot_bench_cpu_vector_sum_parallel.png")
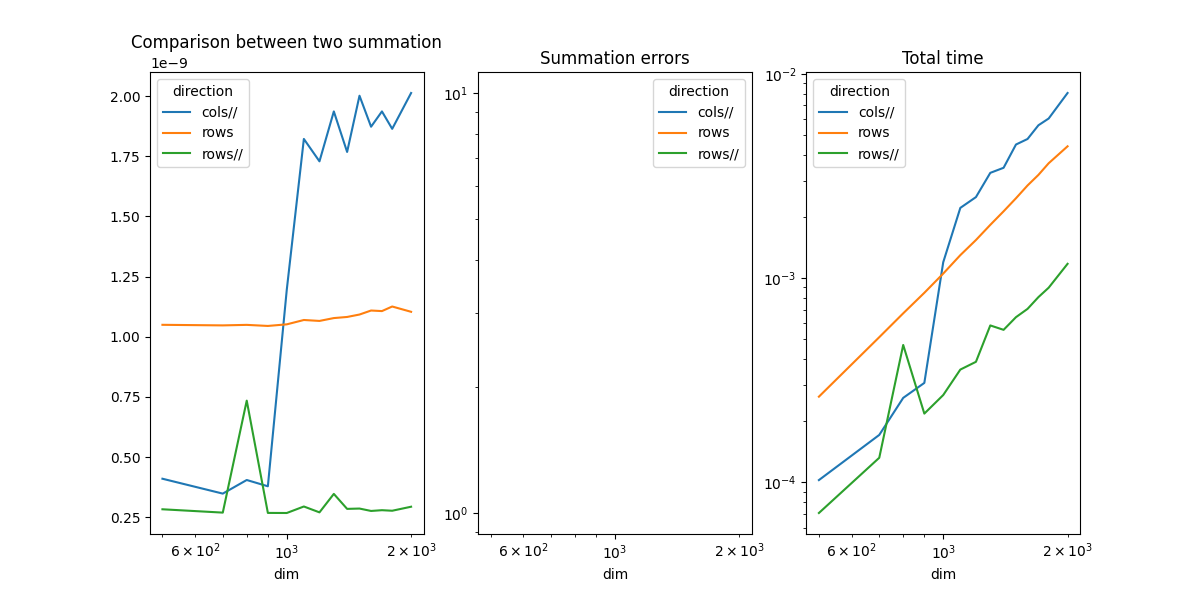
~/.local/lib/python3.10/site-packages/pandas/plotting/_matplotlib/core.py:744: UserWarning: Data has no positive values, and therefore cannot be log-scaled.
labels = axis.get_majorticklabels() + axis.get_minorticklabels()
The summation by rows is much faster as expected. That explains why it is usually more efficient to transpose the first matrix before a matrix multiplication. Parallelization is faster.
Total running time of the script: ( 0 minutes 26.322 seconds)