Note
Go to the end to download the full example code.
Benchmark of TreeEnsemble implementation¶
The following example compares the inference time between
onnxruntime and sklearn.ensemble.RandomForestRegressor
,
fow different number of estimators, max depth, and parallelization.
It does it for a fixed number of rows and features.
import and registration of necessary converters¶
import pickle
import os
import time
from itertools import product
import matplotlib.pyplot as plt
import numpy
import pandas
from lightgbm import LGBMRegressor
from onnxruntime import InferenceSession, SessionOptions
from psutil import cpu_count
from sphinx_runpython.runpython import run_cmd
from skl2onnx import to_onnx, update_registered_converter
from skl2onnx.common.shape_calculator import calculate_linear_regressor_output_shapes
from sklearn import set_config
from sklearn.ensemble import RandomForestRegressor
from tqdm import tqdm
from xgboost import XGBRegressor
from onnxmltools.convert.xgboost.operator_converters.XGBoost import convert_xgboost
def skl2onnx_convert_lightgbm(scope, operator, container):
from onnxmltools.convert.lightgbm.operator_converters.LightGbm import (
convert_lightgbm,
)
options = scope.get_options(operator.raw_operator)
operator.split = options.get("split", None)
convert_lightgbm(scope, operator, container)
update_registered_converter(
LGBMRegressor,
"LightGbmLGBMRegressor",
calculate_linear_regressor_output_shapes,
skl2onnx_convert_lightgbm,
options={"split": None},
)
update_registered_converter(
XGBRegressor,
"XGBoostXGBRegressor",
calculate_linear_regressor_output_shapes,
convert_xgboost,
)
# The following instruction reduces the time spent by scikit-learn
# to validate the data.
set_config(assume_finite=True)
Machine details¶
print(f"Number of cores: {cpu_count()}")
Number of cores: 20
But this information is not usually enough. Let’s extract the cache information.
<Popen: returncode: None args: ['lscpu']>
Or with the following command.
<Popen: returncode: None args: ['cat', '/proc/cpuinfo']>
Fonction to measure inference time¶
def measure_inference(fct, X, repeat, max_time=5, quantile=1):
"""
Run *repeat* times the same function on data *X*.
:param fct: fonction to run
:param X: data
:param repeat: number of times to run
:param max_time: maximum time to use to measure the inference
:return: number of runs, sum of the time, average, median
"""
times = []
for _n in range(repeat):
perf = time.perf_counter()
fct(X)
delta = time.perf_counter() - perf
times.append(delta)
if len(times) < 3:
continue
if max_time is not None and sum(times) >= max_time:
break
times.sort()
quantile = 0 if (len(times) - quantile * 2) < 3 else quantile
if quantile == 0:
tt = times
else:
tt = times[quantile:-quantile]
return (len(times), sum(times), sum(tt) / len(tt), times[len(times) // 2])
Benchmark¶
The following script benchmarks the inference for the same model for a random forest and onnxruntime after it was converted into ONNX and for the following configurations.
small = cpu_count() < 12
if small:
N = 1000
n_features = 10
n_jobs = [1, cpu_count() // 2, cpu_count()]
n_ests = [10, 20, 30]
depth = [4, 6, 8, 10]
Regressor = RandomForestRegressor
else:
N = 100000
n_features = 50
n_jobs = [cpu_count(), cpu_count() // 2, 1]
n_ests = [100, 200, 400]
depth = [6, 8, 10, 12, 14]
Regressor = RandomForestRegressor
legend = f"parallel-nf-{n_features}-"
# avoid duplicates on machine with 1 or 2 cores.
n_jobs = list(sorted(set(n_jobs), reverse=True))
Benchmark parameters
Data
X = numpy.random.randn(N, n_features).astype(numpy.float32)
noise = (numpy.random.randn(X.shape[0]) / (n_features // 5)).astype(numpy.float32)
y = X.mean(axis=1) + noise
n_train = min(N, N // 3)
data = []
couples = list(product(n_jobs, depth, n_ests))
bar = tqdm(couples)
cache_dir = "_cache"
if not os.path.exists(cache_dir):
os.mkdir(cache_dir)
for n_j, max_depth, n_estimators in bar:
if n_j == 1 and n_estimators > n_ests[0]:
# skipping
continue
# parallelization
cache_name = os.path.join(
cache_dir, f"nf-{X.shape[1]}-rf-J-{n_j}-E-{n_estimators}-D-{max_depth}.pkl"
)
if os.path.exists(cache_name):
with open(cache_name, "rb") as f:
rf = pickle.load(f)
else:
bar.set_description(f"J={n_j} E={n_estimators} D={max_depth} train rf")
if n_j == 1 and issubclass(Regressor, RandomForestRegressor):
rf = Regressor(max_depth=max_depth, n_estimators=n_estimators, n_jobs=-1)
rf.fit(X[:n_train], y[:n_train])
rf.n_jobs = 1
else:
rf = Regressor(max_depth=max_depth, n_estimators=n_estimators, n_jobs=n_j)
rf.fit(X[:n_train], y[:n_train])
with open(cache_name, "wb") as f:
pickle.dump(rf, f)
bar.set_description(f"J={n_j} E={n_estimators} D={max_depth} ISession")
so = SessionOptions()
so.intra_op_num_threads = n_j
cache_name = os.path.join(
cache_dir, f"nf-{X.shape[1]}-rf-J-{n_j}-E-{n_estimators}-D-{max_depth}.onnx"
)
if os.path.exists(cache_name):
sess = InferenceSession(cache_name, so, providers=["CPUExecutionProvider"])
else:
bar.set_description(f"J={n_j} E={n_estimators} D={max_depth} cvt onnx")
onx = to_onnx(rf, X[:1])
with open(cache_name, "wb") as f:
f.write(onx.SerializeToString())
sess = InferenceSession(cache_name, so, providers=["CPUExecutionProvider"])
onx_size = os.stat(cache_name).st_size
# run once to avoid counting the first run
bar.set_description(f"J={n_j} E={n_estimators} D={max_depth} predict1")
rf.predict(X)
sess.run(None, {"X": X})
# fixed data
obs = dict(
n_jobs=n_j,
max_depth=max_depth,
n_estimators=n_estimators,
repeat=repeat,
max_time=max_time,
name=rf.__class__.__name__,
n_rows=X.shape[0],
n_features=X.shape[1],
onnx_size=onx_size,
)
# baseline
bar.set_description(f"J={n_j} E={n_estimators} D={max_depth} predictB")
r, t, mean, med = measure_inference(rf.predict, X, repeat=repeat, max_time=max_time)
o1 = obs.copy()
o1.update(dict(avg=mean, med=med, n_runs=r, ttime=t, name="base"))
data.append(o1)
# onnxruntime
bar.set_description(f"J={n_j} E={n_estimators} D={max_depth} predictO")
r, t, mean, med = measure_inference(
lambda x, sess=sess: sess.run(None, {"X": x}),
X,
repeat=repeat,
max_time=max_time,
)
o2 = obs.copy()
o2.update(dict(avg=mean, med=med, n_runs=r, ttime=t, name="ort_"))
data.append(o2)
0%| | 0/45 [00:00<?, ?it/s]~/vv/this312/lib/python3.12/site-packages/sklearn/base.py:380: InconsistentVersionWarning: Trying to unpickle estimator DecisionTreeRegressor from version 1.6.dev0 when using version 1.6.1. This might lead to breaking code or invalid results. Use at your own risk. For more info please refer to:
https://scikit-learn.org/stable/model_persistence.html#security-maintainability-limitations
warnings.warn(
~/vv/this312/lib/python3.12/site-packages/sklearn/base.py:380: InconsistentVersionWarning: Trying to unpickle estimator RandomForestRegressor from version 1.6.dev0 when using version 1.6.1. This might lead to breaking code or invalid results. Use at your own risk. For more info please refer to:
https://scikit-learn.org/stable/model_persistence.html#security-maintainability-limitations
warnings.warn(
J=20 E=100 D=6 ISession: 0%| | 0/45 [00:00<?, ?it/s]
J=20 E=100 D=6 predict1: 0%| | 0/45 [00:00<?, ?it/s]
J=20 E=100 D=6 predictB: 0%| | 0/45 [00:00<?, ?it/s]
J=20 E=100 D=6 predictO: 0%| | 0/45 [00:01<?, ?it/s]
J=20 E=100 D=6 predictO: 2%|▏ | 1/45 [00:03<02:35, 3.54s/it]~/vv/this312/lib/python3.12/site-packages/sklearn/base.py:380: InconsistentVersionWarning: Trying to unpickle estimator DecisionTreeRegressor from version 1.6.dev0 when using version 1.6.1. This might lead to breaking code or invalid results. Use at your own risk. For more info please refer to:
https://scikit-learn.org/stable/model_persistence.html#security-maintainability-limitations
warnings.warn(
~/vv/this312/lib/python3.12/site-packages/sklearn/base.py:380: InconsistentVersionWarning: Trying to unpickle estimator RandomForestRegressor from version 1.6.dev0 when using version 1.6.1. This might lead to breaking code or invalid results. Use at your own risk. For more info please refer to:
https://scikit-learn.org/stable/model_persistence.html#security-maintainability-limitations
warnings.warn(
J=20 E=200 D=6 ISession: 2%|▏ | 1/45 [00:03<02:35, 3.54s/it]
J=20 E=200 D=6 predict1: 2%|▏ | 1/45 [00:03<02:35, 3.54s/it]
J=20 E=200 D=6 predictB: 2%|▏ | 1/45 [00:04<02:35, 3.54s/it]
J=20 E=200 D=6 predictO: 2%|▏ | 1/45 [00:06<02:35, 3.54s/it]
J=20 E=200 D=6 predictO: 4%|▍ | 2/45 [00:09<03:35, 5.00s/it]~/vv/this312/lib/python3.12/site-packages/sklearn/base.py:380: InconsistentVersionWarning: Trying to unpickle estimator DecisionTreeRegressor from version 1.6.dev0 when using version 1.6.1. This might lead to breaking code or invalid results. Use at your own risk. For more info please refer to:
https://scikit-learn.org/stable/model_persistence.html#security-maintainability-limitations
warnings.warn(
~/vv/this312/lib/python3.12/site-packages/sklearn/base.py:380: InconsistentVersionWarning: Trying to unpickle estimator RandomForestRegressor from version 1.6.dev0 when using version 1.6.1. This might lead to breaking code or invalid results. Use at your own risk. For more info please refer to:
https://scikit-learn.org/stable/model_persistence.html#security-maintainability-limitations
warnings.warn(
J=20 E=400 D=6 ISession: 4%|▍ | 2/45 [00:09<03:35, 5.00s/it]
J=20 E=400 D=6 predict1: 4%|▍ | 2/45 [00:09<03:35, 5.00s/it]
J=20 E=400 D=6 predictB: 4%|▍ | 2/45 [00:10<03:35, 5.00s/it]
J=20 E=400 D=6 predictO: 4%|▍ | 2/45 [00:13<03:35, 5.00s/it]
J=20 E=400 D=6 predictO: 7%|▋ | 3/45 [00:18<04:40, 6.68s/it]~/vv/this312/lib/python3.12/site-packages/sklearn/base.py:380: InconsistentVersionWarning: Trying to unpickle estimator DecisionTreeRegressor from version 1.6.dev0 when using version 1.6.1. This might lead to breaking code or invalid results. Use at your own risk. For more info please refer to:
https://scikit-learn.org/stable/model_persistence.html#security-maintainability-limitations
warnings.warn(
~/vv/this312/lib/python3.12/site-packages/sklearn/base.py:380: InconsistentVersionWarning: Trying to unpickle estimator RandomForestRegressor from version 1.6.dev0 when using version 1.6.1. This might lead to breaking code or invalid results. Use at your own risk. For more info please refer to:
https://scikit-learn.org/stable/model_persistence.html#security-maintainability-limitations
warnings.warn(
J=20 E=100 D=8 ISession: 7%|▋ | 3/45 [00:18<04:40, 6.68s/it]
J=20 E=100 D=8 predict1: 7%|▋ | 3/45 [00:18<04:40, 6.68s/it]
J=20 E=100 D=8 predictB: 7%|▋ | 3/45 [00:18<04:40, 6.68s/it]
J=20 E=100 D=8 predictO: 7%|▋ | 3/45 [00:19<04:40, 6.68s/it]
J=20 E=100 D=8 predictO: 9%|▉ | 4/45 [00:21<03:37, 5.30s/it]~/vv/this312/lib/python3.12/site-packages/sklearn/base.py:380: InconsistentVersionWarning: Trying to unpickle estimator DecisionTreeRegressor from version 1.6.dev0 when using version 1.6.1. This might lead to breaking code or invalid results. Use at your own risk. For more info please refer to:
https://scikit-learn.org/stable/model_persistence.html#security-maintainability-limitations
warnings.warn(
~/vv/this312/lib/python3.12/site-packages/sklearn/base.py:380: InconsistentVersionWarning: Trying to unpickle estimator RandomForestRegressor from version 1.6.dev0 when using version 1.6.1. This might lead to breaking code or invalid results. Use at your own risk. For more info please refer to:
https://scikit-learn.org/stable/model_persistence.html#security-maintainability-limitations
warnings.warn(
J=20 E=200 D=8 ISession: 9%|▉ | 4/45 [00:21<03:37, 5.30s/it]
J=20 E=200 D=8 predict1: 9%|▉ | 4/45 [00:21<03:37, 5.30s/it]
J=20 E=200 D=8 predictB: 9%|▉ | 4/45 [00:22<03:37, 5.30s/it]
J=20 E=200 D=8 predictO: 9%|▉ | 4/45 [00:24<03:37, 5.30s/it]
J=20 E=200 D=8 predictO: 11%|█ | 5/45 [00:26<03:34, 5.37s/it]~/vv/this312/lib/python3.12/site-packages/sklearn/base.py:380: InconsistentVersionWarning: Trying to unpickle estimator DecisionTreeRegressor from version 1.6.dev0 when using version 1.6.1. This might lead to breaking code or invalid results. Use at your own risk. For more info please refer to:
https://scikit-learn.org/stable/model_persistence.html#security-maintainability-limitations
warnings.warn(
~/vv/this312/lib/python3.12/site-packages/sklearn/base.py:380: InconsistentVersionWarning: Trying to unpickle estimator RandomForestRegressor from version 1.6.dev0 when using version 1.6.1. This might lead to breaking code or invalid results. Use at your own risk. For more info please refer to:
https://scikit-learn.org/stable/model_persistence.html#security-maintainability-limitations
warnings.warn(
J=20 E=400 D=8 ISession: 11%|█ | 5/45 [00:26<03:34, 5.37s/it]
J=20 E=400 D=8 predict1: 11%|█ | 5/45 [00:27<03:34, 5.37s/it]
J=20 E=400 D=8 predictB: 11%|█ | 5/45 [00:27<03:34, 5.37s/it]
J=20 E=400 D=8 predictO: 11%|█ | 5/45 [00:30<03:34, 5.37s/it]
J=20 E=400 D=8 predictO: 13%|█▎ | 6/45 [00:35<04:05, 6.30s/it]~/vv/this312/lib/python3.12/site-packages/sklearn/base.py:380: InconsistentVersionWarning: Trying to unpickle estimator DecisionTreeRegressor from version 1.6.dev0 when using version 1.6.1. This might lead to breaking code or invalid results. Use at your own risk. For more info please refer to:
https://scikit-learn.org/stable/model_persistence.html#security-maintainability-limitations
warnings.warn(
~/vv/this312/lib/python3.12/site-packages/sklearn/base.py:380: InconsistentVersionWarning: Trying to unpickle estimator RandomForestRegressor from version 1.6.dev0 when using version 1.6.1. This might lead to breaking code or invalid results. Use at your own risk. For more info please refer to:
https://scikit-learn.org/stable/model_persistence.html#security-maintainability-limitations
warnings.warn(
J=20 E=100 D=10 ISession: 13%|█▎ | 6/45 [00:35<04:05, 6.30s/it]
J=20 E=100 D=10 predict1: 13%|█▎ | 6/45 [00:35<04:05, 6.30s/it]
J=20 E=100 D=10 predictB: 13%|█▎ | 6/45 [00:35<04:05, 6.30s/it]
J=20 E=100 D=10 predictO: 13%|█▎ | 6/45 [00:36<04:05, 6.30s/it]
J=20 E=100 D=10 predictO: 16%|█▌ | 7/45 [00:37<03:17, 5.21s/it]~/vv/this312/lib/python3.12/site-packages/sklearn/base.py:380: InconsistentVersionWarning: Trying to unpickle estimator DecisionTreeRegressor from version 1.6.dev0 when using version 1.6.1. This might lead to breaking code or invalid results. Use at your own risk. For more info please refer to:
https://scikit-learn.org/stable/model_persistence.html#security-maintainability-limitations
warnings.warn(
~/vv/this312/lib/python3.12/site-packages/sklearn/base.py:380: InconsistentVersionWarning: Trying to unpickle estimator RandomForestRegressor from version 1.6.dev0 when using version 1.6.1. This might lead to breaking code or invalid results. Use at your own risk. For more info please refer to:
https://scikit-learn.org/stable/model_persistence.html#security-maintainability-limitations
warnings.warn(
J=20 E=200 D=10 ISession: 16%|█▌ | 7/45 [00:38<03:17, 5.21s/it]
J=20 E=200 D=10 predict1: 16%|█▌ | 7/45 [00:38<03:17, 5.21s/it]
J=20 E=200 D=10 predictB: 16%|█▌ | 7/45 [00:38<03:17, 5.21s/it]
J=20 E=200 D=10 predictO: 16%|█▌ | 7/45 [00:40<03:17, 5.21s/it]
J=20 E=200 D=10 predictO: 18%|█▊ | 8/45 [00:42<03:05, 5.01s/it]~/vv/this312/lib/python3.12/site-packages/sklearn/base.py:380: InconsistentVersionWarning: Trying to unpickle estimator DecisionTreeRegressor from version 1.6.dev0 when using version 1.6.1. This might lead to breaking code or invalid results. Use at your own risk. For more info please refer to:
https://scikit-learn.org/stable/model_persistence.html#security-maintainability-limitations
warnings.warn(
~/vv/this312/lib/python3.12/site-packages/sklearn/base.py:380: InconsistentVersionWarning: Trying to unpickle estimator RandomForestRegressor from version 1.6.dev0 when using version 1.6.1. This might lead to breaking code or invalid results. Use at your own risk. For more info please refer to:
https://scikit-learn.org/stable/model_persistence.html#security-maintainability-limitations
warnings.warn(
J=20 E=400 D=10 ISession: 18%|█▊ | 8/45 [00:42<03:05, 5.01s/it]
J=20 E=400 D=10 predict1: 18%|█▊ | 8/45 [00:43<03:05, 5.01s/it]
J=20 E=400 D=10 predictB: 18%|█▊ | 8/45 [00:44<03:05, 5.01s/it]
J=20 E=400 D=10 predictO: 18%|█▊ | 8/45 [00:48<03:05, 5.01s/it]
J=20 E=400 D=10 predictO: 20%|██ | 9/45 [00:52<04:00, 6.69s/it]~/vv/this312/lib/python3.12/site-packages/sklearn/base.py:380: InconsistentVersionWarning: Trying to unpickle estimator DecisionTreeRegressor from version 1.6.dev0 when using version 1.6.1. This might lead to breaking code or invalid results. Use at your own risk. For more info please refer to:
https://scikit-learn.org/stable/model_persistence.html#security-maintainability-limitations
warnings.warn(
~/vv/this312/lib/python3.12/site-packages/sklearn/base.py:380: InconsistentVersionWarning: Trying to unpickle estimator RandomForestRegressor from version 1.6.dev0 when using version 1.6.1. This might lead to breaking code or invalid results. Use at your own risk. For more info please refer to:
https://scikit-learn.org/stable/model_persistence.html#security-maintainability-limitations
warnings.warn(
J=20 E=100 D=12 ISession: 20%|██ | 9/45 [00:52<04:00, 6.69s/it]
J=20 E=100 D=12 predict1: 20%|██ | 9/45 [00:53<04:00, 6.69s/it]
J=20 E=100 D=12 predictB: 20%|██ | 9/45 [00:53<04:00, 6.69s/it]
J=20 E=100 D=12 predictO: 20%|██ | 9/45 [00:54<04:00, 6.69s/it]
J=20 E=100 D=12 predictO: 22%|██▏ | 10/45 [00:57<03:33, 6.09s/it]
J=20 E=200 D=12 ISession: 22%|██▏ | 10/45 [00:57<03:33, 6.09s/it]
J=20 E=200 D=12 predict1: 22%|██▏ | 10/45 [00:59<03:33, 6.09s/it]
J=20 E=200 D=12 predictB: 22%|██▏ | 10/45 [01:00<03:33, 6.09s/it]
J=20 E=200 D=12 predictO: 22%|██▏ | 10/45 [01:03<03:33, 6.09s/it]
J=20 E=200 D=12 predictO: 24%|██▍ | 11/45 [01:08<04:20, 7.67s/it]
J=20 E=400 D=12 ISession: 24%|██▍ | 11/45 [01:09<04:20, 7.67s/it]
J=20 E=400 D=12 predict1: 24%|██▍ | 11/45 [01:11<04:20, 7.67s/it]
J=20 E=400 D=12 predictB: 24%|██▍ | 11/45 [01:14<04:20, 7.67s/it]
J=20 E=400 D=12 predictO: 24%|██▍ | 11/45 [01:18<04:20, 7.67s/it]
J=20 E=400 D=12 predictO: 27%|██▋ | 12/45 [01:24<05:28, 9.95s/it]
J=20 E=100 D=14 ISession: 27%|██▋ | 12/45 [01:24<05:28, 9.95s/it]
J=20 E=100 D=14 predict1: 27%|██▋ | 12/45 [01:25<05:28, 9.95s/it]
J=20 E=100 D=14 predictB: 27%|██▋ | 12/45 [01:26<05:28, 9.95s/it]
J=20 E=100 D=14 predictO: 27%|██▋ | 12/45 [01:27<05:28, 9.95s/it]
J=20 E=100 D=14 predictO: 29%|██▉ | 13/45 [01:31<04:53, 9.18s/it]
J=20 E=200 D=14 ISession: 29%|██▉ | 13/45 [01:31<04:53, 9.18s/it]
J=20 E=200 D=14 predict1: 29%|██▉ | 13/45 [01:33<04:53, 9.18s/it]
J=20 E=200 D=14 predictB: 29%|██▉ | 13/45 [01:35<04:53, 9.18s/it]
J=20 E=200 D=14 predictO: 29%|██▉ | 13/45 [01:38<04:53, 9.18s/it]
J=20 E=200 D=14 predictO: 31%|███ | 14/45 [01:43<05:09, 9.97s/it]
J=20 E=400 D=14 ISession: 31%|███ | 14/45 [01:43<05:09, 9.97s/it]
J=20 E=400 D=14 predict1: 31%|███ | 14/45 [01:48<05:09, 9.97s/it]
J=20 E=400 D=14 predictB: 31%|███ | 14/45 [01:51<05:09, 9.97s/it]
J=20 E=400 D=14 predictO: 31%|███ | 14/45 [01:56<05:09, 9.97s/it]
J=20 E=400 D=14 predictO: 33%|███▎ | 15/45 [02:04<06:38, 13.28s/it]
J=10 E=100 D=6 ISession: 33%|███▎ | 15/45 [02:04<06:38, 13.28s/it]
J=10 E=100 D=6 predict1: 33%|███▎ | 15/45 [02:04<06:38, 13.28s/it]
J=10 E=100 D=6 predictB: 33%|███▎ | 15/45 [02:04<06:38, 13.28s/it]
J=10 E=100 D=6 predictO: 33%|███▎ | 15/45 [02:05<06:38, 13.28s/it]
J=10 E=100 D=6 predictO: 36%|███▌ | 16/45 [02:06<04:45, 9.85s/it]
J=10 E=200 D=6 ISession: 36%|███▌ | 16/45 [02:06<04:45, 9.85s/it]
J=10 E=200 D=6 predict1: 36%|███▌ | 16/45 [02:06<04:45, 9.85s/it]
J=10 E=200 D=6 predictB: 36%|███▌ | 16/45 [02:06<04:45, 9.85s/it]
J=10 E=200 D=6 predictO: 36%|███▌ | 16/45 [02:08<04:45, 9.85s/it]
J=10 E=200 D=6 predictO: 38%|███▊ | 17/45 [02:09<03:41, 7.91s/it]
J=10 E=400 D=6 train rf: 38%|███▊ | 17/45 [02:09<03:41, 7.91s/it]
J=10 E=400 D=6 ISession: 38%|███▊ | 17/45 [03:06<03:41, 7.91s/it]
J=10 E=400 D=6 cvt onnx: 38%|███▊ | 17/45 [03:06<03:41, 7.91s/it]
J=10 E=400 D=6 predict1: 38%|███▊ | 17/45 [03:06<03:41, 7.91s/it]
J=10 E=400 D=6 predictB: 38%|███▊ | 17/45 [03:07<03:41, 7.91s/it]
J=10 E=400 D=6 predictO: 38%|███▊ | 17/45 [03:10<03:41, 7.91s/it]
J=10 E=400 D=6 predictO: 40%|████ | 18/45 [03:13<11:04, 24.61s/it]
J=10 E=100 D=8 train rf: 40%|████ | 18/45 [03:13<11:04, 24.61s/it]
J=10 E=100 D=8 ISession: 40%|████ | 18/45 [03:31<11:04, 24.61s/it]
J=10 E=100 D=8 cvt onnx: 40%|████ | 18/45 [03:31<11:04, 24.61s/it]
J=10 E=100 D=8 predict1: 40%|████ | 18/45 [03:31<11:04, 24.61s/it]
J=10 E=100 D=8 predictB: 40%|████ | 18/45 [03:32<11:04, 24.61s/it]
J=10 E=100 D=8 predictO: 40%|████ | 18/45 [03:32<11:04, 24.61s/it]
J=10 E=100 D=8 predictO: 42%|████▏ | 19/45 [03:33<10:08, 23.40s/it]
J=10 E=200 D=8 train rf: 42%|████▏ | 19/45 [03:33<10:08, 23.40s/it]
J=10 E=200 D=8 ISession: 42%|████▏ | 19/45 [04:15<10:08, 23.40s/it]
J=10 E=200 D=8 cvt onnx: 42%|████▏ | 19/45 [04:15<10:08, 23.40s/it]
J=10 E=200 D=8 predict1: 42%|████▏ | 19/45 [04:16<10:08, 23.40s/it]
J=10 E=200 D=8 predictB: 42%|████▏ | 19/45 [04:16<10:08, 23.40s/it]
J=10 E=200 D=8 predictO: 42%|████▏ | 19/45 [04:18<10:08, 23.40s/it]
J=10 E=200 D=8 predictO: 44%|████▍ | 20/45 [04:19<12:35, 30.20s/it]
J=10 E=400 D=8 train rf: 44%|████▍ | 20/45 [04:19<12:35, 30.20s/it]
J=10 E=400 D=8 ISession: 44%|████▍ | 20/45 [05:32<12:35, 30.20s/it]
J=10 E=400 D=8 cvt onnx: 44%|████▍ | 20/45 [05:32<12:35, 30.20s/it]
J=10 E=400 D=8 predict1: 44%|████▍ | 20/45 [05:33<12:35, 30.20s/it]
J=10 E=400 D=8 predictB: 44%|████▍ | 20/45 [05:35<12:35, 30.20s/it]
J=10 E=400 D=8 predictO: 44%|████▍ | 20/45 [05:38<12:35, 30.20s/it]
J=10 E=400 D=8 predictO: 47%|████▋ | 21/45 [05:41<18:18, 45.76s/it]
J=10 E=100 D=10 train rf: 47%|████▋ | 21/45 [05:41<18:18, 45.76s/it]
J=10 E=100 D=10 ISession: 47%|████▋ | 21/45 [06:04<18:18, 45.76s/it]
J=10 E=100 D=10 cvt onnx: 47%|████▋ | 21/45 [06:04<18:18, 45.76s/it]
J=10 E=100 D=10 predict1: 47%|████▋ | 21/45 [06:05<18:18, 45.76s/it]
J=10 E=100 D=10 predictB: 47%|████▋ | 21/45 [06:05<18:18, 45.76s/it]
J=10 E=100 D=10 predictO: 47%|████▋ | 21/45 [06:06<18:18, 45.76s/it]
J=10 E=100 D=10 predictO: 49%|████▉ | 22/45 [06:07<15:14, 39.78s/it]
J=10 E=200 D=10 train rf: 49%|████▉ | 22/45 [06:07<15:14, 39.78s/it]
J=10 E=200 D=10 ISession: 49%|████▉ | 22/45 [06:50<15:14, 39.78s/it]
J=10 E=200 D=10 cvt onnx: 49%|████▉ | 22/45 [06:50<15:14, 39.78s/it]
J=10 E=200 D=10 predict1: 49%|████▉ | 22/45 [06:52<15:14, 39.78s/it]
J=10 E=200 D=10 predictB: 49%|████▉ | 22/45 [06:53<15:14, 39.78s/it]
J=10 E=200 D=10 predictO: 49%|████▉ | 22/45 [06:55<15:14, 39.78s/it]
J=10 E=200 D=10 predictO: 51%|█████ | 23/45 [06:57<15:40, 42.75s/it]
J=10 E=400 D=10 train rf: 51%|█████ | 23/45 [06:57<15:40, 42.75s/it]
J=10 E=400 D=10 ISession: 51%|█████ | 23/45 [08:25<15:40, 42.75s/it]
J=10 E=400 D=10 cvt onnx: 51%|█████ | 23/45 [08:25<15:40, 42.75s/it]
J=10 E=400 D=10 predict1: 51%|█████ | 23/45 [08:31<15:40, 42.75s/it]
J=10 E=400 D=10 predictB: 51%|█████ | 23/45 [08:32<15:40, 42.75s/it]
J=10 E=400 D=10 predictO: 51%|█████ | 23/45 [08:35<15:40, 42.75s/it]
J=10 E=400 D=10 predictO: 53%|█████▎ | 24/45 [08:40<21:17, 60.83s/it]
J=10 E=100 D=12 train rf: 53%|█████▎ | 24/45 [08:40<21:17, 60.83s/it]
J=10 E=100 D=12 ISession: 53%|█████▎ | 24/45 [09:06<21:17, 60.83s/it]
J=10 E=100 D=12 cvt onnx: 53%|█████▎ | 24/45 [09:06<21:17, 60.83s/it]
J=10 E=100 D=12 predict1: 53%|█████▎ | 24/45 [09:11<21:17, 60.83s/it]
J=10 E=100 D=12 predictB: 53%|█████▎ | 24/45 [09:11<21:17, 60.83s/it]
J=10 E=100 D=12 predictO: 53%|█████▎ | 24/45 [09:13<21:17, 60.83s/it]
J=10 E=100 D=12 predictO: 56%|█████▌ | 25/45 [09:14<17:37, 52.90s/it]
J=10 E=200 D=12 train rf: 56%|█████▌ | 25/45 [09:14<17:37, 52.90s/it]
J=10 E=200 D=12 ISession: 56%|█████▌ | 25/45 [10:07<17:37, 52.90s/it]
J=10 E=200 D=12 cvt onnx: 56%|█████▌ | 25/45 [10:07<17:37, 52.90s/it]
J=10 E=200 D=12 predict1: 56%|█████▌ | 25/45 [10:16<17:37, 52.90s/it]
J=10 E=200 D=12 predictB: 56%|█████▌ | 25/45 [10:17<17:37, 52.90s/it]
J=10 E=200 D=12 predictO: 56%|█████▌ | 25/45 [10:19<17:37, 52.90s/it]
J=10 E=200 D=12 predictO: 58%|█████▊ | 26/45 [10:23<18:14, 57.63s/it]
J=10 E=400 D=12 train rf: 58%|█████▊ | 26/45 [10:23<18:14, 57.63s/it]
J=10 E=400 D=12 ISession: 58%|█████▊ | 26/45 [12:02<18:14, 57.63s/it]
J=10 E=400 D=12 cvt onnx: 58%|█████▊ | 26/45 [12:02<18:14, 57.63s/it]
J=10 E=400 D=12 predict1: 58%|█████▊ | 26/45 [12:19<18:14, 57.63s/it]
J=10 E=400 D=12 predictB: 58%|█████▊ | 26/45 [12:21<18:14, 57.63s/it]
J=10 E=400 D=12 predictO: 58%|█████▊ | 26/45 [12:26<18:14, 57.63s/it]
J=10 E=400 D=12 predictO: 60%|██████ | 27/45 [12:31<23:37, 78.77s/it]
J=10 E=100 D=14 train rf: 60%|██████ | 27/45 [12:31<23:37, 78.77s/it]
J=10 E=100 D=14 ISession: 60%|██████ | 27/45 [12:58<23:37, 78.77s/it]
J=10 E=100 D=14 cvt onnx: 60%|██████ | 27/45 [12:58<23:37, 78.77s/it]
J=10 E=100 D=14 predict1: 60%|██████ | 27/45 [13:08<23:37, 78.77s/it]
J=10 E=100 D=14 predictB: 60%|██████ | 27/45 [13:09<23:37, 78.77s/it]
J=10 E=100 D=14 predictO: 60%|██████ | 27/45 [13:11<23:37, 78.77s/it]
J=10 E=100 D=14 predictO: 62%|██████▏ | 28/45 [13:13<19:11, 67.75s/it]
J=10 E=200 D=14 train rf: 62%|██████▏ | 28/45 [13:13<19:11, 67.75s/it]
J=10 E=200 D=14 ISession: 62%|██████▏ | 28/45 [14:08<19:11, 67.75s/it]
J=10 E=200 D=14 cvt onnx: 62%|██████▏ | 28/45 [14:08<19:11, 67.75s/it]
J=10 E=200 D=14 predict1: 62%|██████▏ | 28/45 [14:28<19:11, 67.75s/it]
J=10 E=200 D=14 predictB: 62%|██████▏ | 28/45 [14:29<19:11, 67.75s/it]
J=10 E=200 D=14 predictO: 62%|██████▏ | 28/45 [14:33<19:11, 67.75s/it]
J=10 E=200 D=14 predictO: 64%|██████▍ | 29/45 [14:38<19:27, 72.97s/it]
J=10 E=400 D=14 train rf: 64%|██████▍ | 29/45 [14:38<19:27, 72.97s/it]
J=10 E=400 D=14 ISession: 64%|██████▍ | 29/45 [16:27<19:27, 72.97s/it]
J=10 E=400 D=14 cvt onnx: 64%|██████▍ | 29/45 [16:27<19:27, 72.97s/it]
J=10 E=400 D=14 predict1: 64%|██████▍ | 29/45 [17:07<19:27, 72.97s/it]
J=10 E=400 D=14 predictB: 64%|██████▍ | 29/45 [17:10<19:27, 72.97s/it]
J=10 E=400 D=14 predictO: 64%|██████▍ | 29/45 [17:16<19:27, 72.97s/it]
J=10 E=400 D=14 predictO: 67%|██████▋ | 30/45 [17:22<25:04, 100.31s/it]
J=1 E=100 D=6 train rf: 67%|██████▋ | 30/45 [17:22<25:04, 100.31s/it]
J=1 E=100 D=6 ISession: 67%|██████▋ | 30/45 [17:32<25:04, 100.31s/it]
J=1 E=100 D=6 cvt onnx: 67%|██████▋ | 30/45 [17:32<25:04, 100.31s/it]
J=1 E=100 D=6 predict1: 67%|██████▋ | 30/45 [17:32<25:04, 100.31s/it]
J=1 E=100 D=6 predictB: 67%|██████▋ | 30/45 [17:33<25:04, 100.31s/it]
J=1 E=100 D=6 predictO: 67%|██████▋ | 30/45 [17:39<25:04, 100.31s/it]
J=1 E=100 D=6 predictO: 69%|██████▉ | 31/45 [17:42<17:44, 76.04s/it]
J=1 E=100 D=8 train rf: 69%|██████▉ | 31/45 [17:42<17:44, 76.04s/it]
J=1 E=100 D=8 ISession: 69%|██████▉ | 31/45 [17:55<17:44, 76.04s/it]
J=1 E=100 D=8 cvt onnx: 69%|██████▉ | 31/45 [17:55<17:44, 76.04s/it]
J=1 E=100 D=8 predict1: 69%|██████▉ | 31/45 [17:56<17:44, 76.04s/it]
J=1 E=100 D=8 predictB: 69%|██████▉ | 31/45 [17:58<17:44, 76.04s/it]
J=1 E=100 D=8 predictO: 69%|██████▉ | 31/45 [18:03<17:44, 76.04s/it]
J=1 E=100 D=8 predictO: 76%|███████▌ | 34/45 [18:07<06:59, 38.13s/it]
J=1 E=100 D=10 train rf: 76%|███████▌ | 34/45 [18:07<06:59, 38.13s/it]
J=1 E=100 D=10 ISession: 76%|███████▌ | 34/45 [18:22<06:59, 38.13s/it]
J=1 E=100 D=10 cvt onnx: 76%|███████▌ | 34/45 [18:22<06:59, 38.13s/it]
J=1 E=100 D=10 predict1: 76%|███████▌ | 34/45 [18:24<06:59, 38.13s/it]
J=1 E=100 D=10 predictB: 76%|███████▌ | 34/45 [18:26<06:59, 38.13s/it]
J=1 E=100 D=10 predictO: 76%|███████▌ | 34/45 [18:31<06:59, 38.13s/it]
J=1 E=100 D=10 predictO: 82%|████████▏ | 37/45 [18:37<03:24, 25.53s/it]
J=1 E=100 D=12 train rf: 82%|████████▏ | 37/45 [18:37<03:24, 25.53s/it]
J=1 E=100 D=12 ISession: 82%|████████▏ | 37/45 [18:54<03:24, 25.53s/it]
J=1 E=100 D=12 cvt onnx: 82%|████████▏ | 37/45 [18:54<03:24, 25.53s/it]
J=1 E=100 D=12 predict1: 82%|████████▏ | 37/45 [18:58<03:24, 25.53s/it]
J=1 E=100 D=12 predictB: 82%|████████▏ | 37/45 [19:02<03:24, 25.53s/it]
J=1 E=100 D=12 predictO: 82%|████████▏ | 37/45 [19:07<03:24, 25.53s/it]
J=1 E=100 D=12 predictO: 89%|████████▉ | 40/45 [19:12<01:40, 20.15s/it]
J=1 E=100 D=14 train rf: 89%|████████▉ | 40/45 [19:12<01:40, 20.15s/it]
J=1 E=100 D=14 ISession: 89%|████████▉ | 40/45 [19:31<01:40, 20.15s/it]
J=1 E=100 D=14 cvt onnx: 89%|████████▉ | 40/45 [19:31<01:40, 20.15s/it]
J=1 E=100 D=14 predict1: 89%|████████▉ | 40/45 [19:40<01:40, 20.15s/it]
J=1 E=100 D=14 predictB: 89%|████████▉ | 40/45 [19:46<01:40, 20.15s/it]
J=1 E=100 D=14 predictO: 89%|████████▉ | 40/45 [19:53<01:40, 20.15s/it]
J=1 E=100 D=14 predictO: 96%|█████████▌| 43/45 [20:04<00:38, 19.18s/it]
J=1 E=100 D=14 predictO: 100%|██████████| 45/45 [20:04<00:00, 26.78s/it]
Saving data¶
name = os.path.join(cache_dir, "plot_beanchmark_rf")
print(f"Saving data into {name!r}")
df = pandas.DataFrame(data)
df2 = df.copy()
df2["legend"] = legend
df2.to_csv(f"{name}-{legend}.csv", index=False)
Saving data into '_cache/plot_beanchmark_rf'
Printing the data
Plot¶
n_rows = len(n_jobs)
n_cols = len(n_ests)
fig, axes = plt.subplots(n_rows, n_cols, figsize=(4 * n_cols, 4 * n_rows))
fig.suptitle(f"{rf.__class__.__name__}\nX.shape={X.shape}")
for n_j, n_estimators in tqdm(product(n_jobs, n_ests)):
i = n_jobs.index(n_j)
j = n_ests.index(n_estimators)
ax = axes[i, j]
subdf = df[(df.n_estimators == n_estimators) & (df.n_jobs == n_j)]
if subdf.shape[0] == 0:
continue
piv = subdf.pivot(index="max_depth", columns="name", values=["avg", "med"])
piv.plot(ax=ax, title=f"jobs={n_j}, trees={n_estimators}")
ax.set_ylabel(f"n_jobs={n_j}", fontsize="small")
ax.set_xlabel("max_depth", fontsize="small")
# ratio
ax2 = ax.twinx()
piv1 = subdf.pivot(index="max_depth", columns="name", values="avg")
piv1["speedup"] = piv1.base / piv1.ort_
ax2.plot(piv1.index, piv1.speedup, "b--", label="speedup avg")
piv1 = subdf.pivot(index="max_depth", columns="name", values="med")
piv1["speedup"] = piv1.base / piv1.ort_
ax2.plot(piv1.index, piv1.speedup, "y--", label="speedup med")
ax2.legend(fontsize="x-small")
# 1
ax2.plot(piv1.index, [1 for _ in piv1.index], "k--", label="no speedup")
for i in range(axes.shape[0]):
for j in range(axes.shape[1]):
axes[i, j].legend(fontsize="small")
fig.tight_layout()
fig.savefig(f"{name}-{legend}.png")
# plt.show()
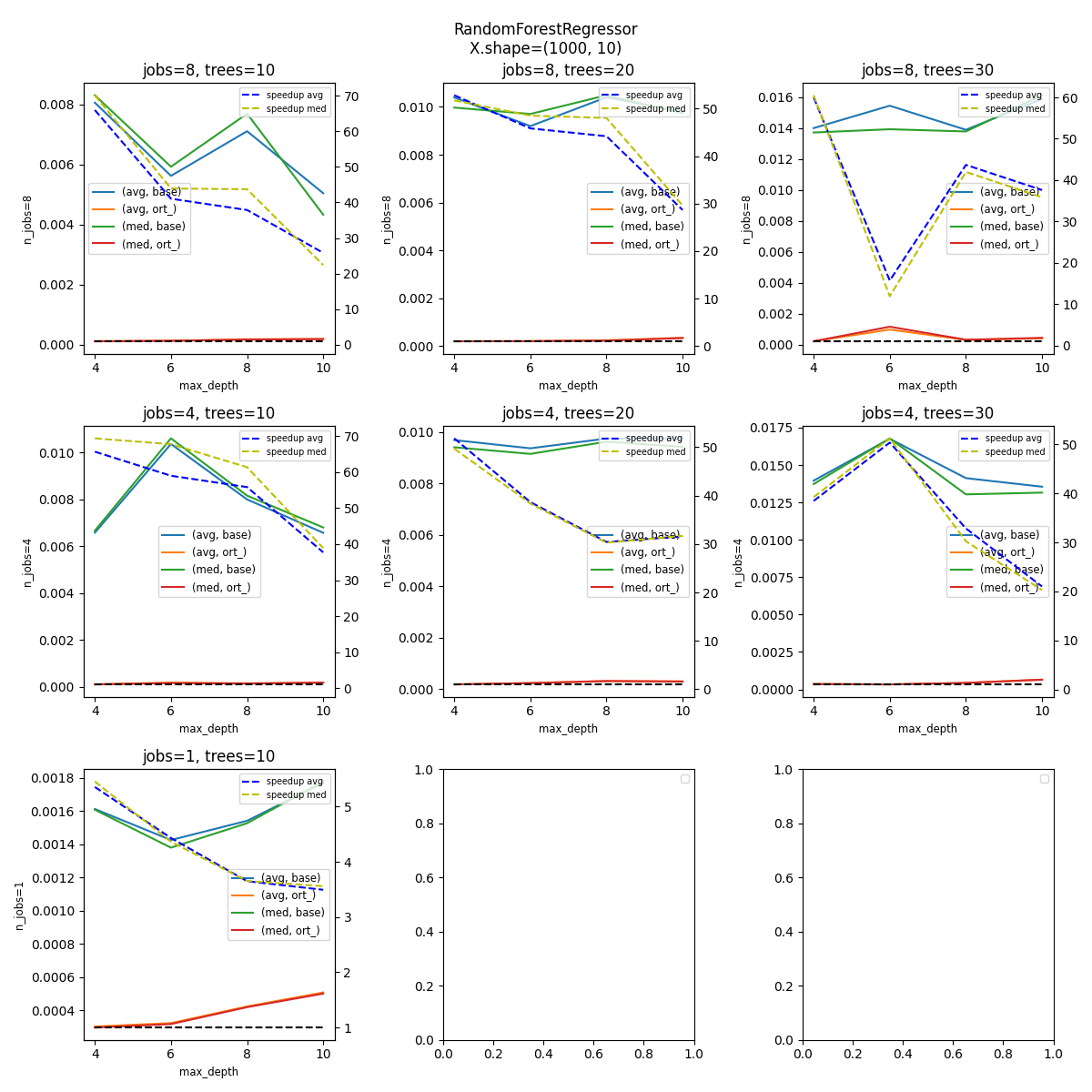
0it [00:00, ?it/s]
3it [00:00, 25.48it/s]
9it [00:00, 43.67it/s]
~/github/onnx-array-api/_doc/examples/plot_benchmark_rf.py:307: UserWarning: No artists with labels found to put in legend. Note that artists whose label start with an underscore are ignored when legend() is called with no argument.
axes[i, j].legend(fontsize="small")
Total running time of the script: (20 minutes 19.615 seconds)