Note
Go to the end to download the full example code.
Export with DynamicCache and dynamic shapes¶
Every LLMs implemented in transformers use cache.
One of the most used is transformers.cache_utils.DynamicCache
.
The cache size is dynamic to cope with the growing context.
The example shows a tool which determines the dynamic shapes
for torch.export.export()
based on a set of valid inputs.
DynamicCache¶
torch.export.export()
serializes caches and any custom class
if these serialization functions are provided with is the case for
transformers.cache_utils.DynamicCache
and transformers>=4.50
.
The dynamic shapes must be provided following the serialized form.
import pprint
import torch
from onnx_diagnostic import doc
from onnx_diagnostic.ext_test_case import has_transformers
from onnx_diagnostic.helpers import string_type
from onnx_diagnostic.helpers.cache_helper import (
flatten_unflatten_for_dynamic_shapes,
make_dynamic_cache,
)
from onnx_diagnostic.export import ModelInputs
from onnx_diagnostic.torch_export_patches import bypass_export_some_errors
class Model(torch.nn.Module):
def forward(self, cache, z):
return (
z
+ cache.key_cache[0]
+ cache.key_cache[1]
+ cache.value_cache[0]
+ cache.value_cache[1]
)
model = Model()
n_layers = 2
bsize, nheads, slen, dim = 2, 4, 3, 7
cache = make_dynamic_cache(
[
(torch.randn(bsize, nheads, slen, dim), torch.randn(bsize, nheads, slen, dim))
for i in range(n_layers)
]
)
z = torch.randn((1, 1, 1, 7))
model(cache, z) # to check it works.
tensor([[[[ 1.0879, -4.4025, -0.5639, -1.7052, -2.7697, 1.9283, -1.1653],
[-0.5613, -3.4949, -4.2692, -0.0347, 0.6953, 0.2130, -0.5996],
[ 1.3986, -2.4371, -0.9807, -5.7749, 0.5617, 5.1113, -0.7553]],
[[ 1.2169, -5.3692, -3.4131, 0.3674, -0.1979, -0.4480, -2.8200],
[-1.6197, -1.4710, -0.7341, -1.1980, 1.0244, -3.6100, 0.6107],
[-1.7430, -6.3176, -1.1941, -0.1924, 0.7366, 3.7406, -3.7864]],
[[-2.5551, -6.3271, -4.0353, -5.4786, 1.8927, -2.2760, 2.0058],
[ 1.3311, -3.5763, -7.4183, -1.5429, 3.2541, -3.2067, -0.1113],
[-3.1554, -3.2774, -5.4761, 0.8289, 1.7326, 1.2602, -3.9402]],
[[-1.8448, -0.1268, -2.3021, 1.6851, -0.5552, 1.0116, -0.2312],
[-1.2887, -6.4735, -3.8228, 0.4302, 5.1972, 2.2793, 1.4141],
[ 2.0251, -0.9554, 1.6148, 0.9268, -1.8032, 0.5013, -0.2910]]],
[[[-0.2265, -5.7365, -0.5601, 5.4271, 2.5116, 4.5368, -0.4415],
[-1.5191, -1.3846, -4.2902, 2.0980, 1.3932, 1.8236, -3.2986],
[-5.7523, -2.2481, 0.5032, -1.1002, 1.1223, 4.0369, -2.2837]],
[[-2.1696, -3.4414, 0.4060, 0.1454, -0.5541, 1.6783, -1.5603],
[-1.9539, -4.5904, -0.9212, -2.8754, -1.9597, 1.0716, 2.0292],
[-0.2276, -2.4370, 1.0865, -0.6559, 4.5598, -1.2136, -2.5132]],
[[-0.2207, -0.9122, 0.3639, -0.7208, 0.9747, 1.1281, 0.4297],
[-1.4834, 1.0119, -0.8298, -1.8405, 1.6603, 1.2144, 2.2213],
[-2.4973, -5.3292, -3.5423, 2.0696, 3.5919, 0.1355, -0.2142]],
[[-5.2598, -1.8040, -0.9179, -0.0652, 3.9455, -1.1817, 1.9416],
[-1.9333, -6.8462, -4.7423, 0.9886, 3.0598, 2.1358, -2.2073],
[-2.5837, -4.1323, 1.9288, -2.2071, 1.4710, 2.3045, -0.3423]]]])
The cache looks like this:
print(string_type(cache, with_shape=True))
DynamicCache(key_cache=#2[T1s2x4x3x7,T1s2x4x3x7], value_cache=#2[T1s2x4x3x7,T1s2x4x3x7])
cache2 = make_dynamic_cache(
[
(
torch.randn(bsize + 1, nheads, slen + 1, dim + 1),
torch.randn(bsize + 1, nheads, slen + 1, dim + 1),
)
for i in range(n_layers)
]
)
inputs = [
(cache, z),
(cache2, torch.randn((1, 1, 1, 8))),
]
And the second set of inputs looks like:
print(string_type(inputs[1], with_shape=True))
(DynamicCache(key_cache=#2[T1s3x4x4x8,T1s3x4x4x8], value_cache=#2[T1s3x4x4x8,T1s3x4x4x8]),T1s1x1x1x8)
Guess the dynamic shapes¶
The following tool can be used to guess the dynamic shapes
the way torch.export.export()
expects them.
mi = ModelInputs(Model(), inputs)
ds = mi.guess_dynamic_shapes()
pprint.pprint(ds)
(([[{0: _DimHint(type=<_DimHintType.DYNAMIC: 3>,
min=None,
max=None,
_factory=True),
2: _DimHint(type=<_DimHintType.DYNAMIC: 3>,
min=None,
max=None,
_factory=True),
3: _DimHint(type=<_DimHintType.DYNAMIC: 3>,
min=None,
max=None,
_factory=True)},
{0: _DimHint(type=<_DimHintType.DYNAMIC: 3>,
min=None,
max=None,
_factory=True),
2: _DimHint(type=<_DimHintType.DYNAMIC: 3>,
min=None,
max=None,
_factory=True),
3: _DimHint(type=<_DimHintType.DYNAMIC: 3>,
min=None,
max=None,
_factory=True)}],
[{0: _DimHint(type=<_DimHintType.DYNAMIC: 3>,
min=None,
max=None,
_factory=True),
2: _DimHint(type=<_DimHintType.DYNAMIC: 3>,
min=None,
max=None,
_factory=True),
3: _DimHint(type=<_DimHintType.DYNAMIC: 3>,
min=None,
max=None,
_factory=True)},
{0: _DimHint(type=<_DimHintType.DYNAMIC: 3>,
min=None,
max=None,
_factory=True),
2: _DimHint(type=<_DimHintType.DYNAMIC: 3>,
min=None,
max=None,
_factory=True),
3: _DimHint(type=<_DimHintType.DYNAMIC: 3>,
min=None,
max=None,
_factory=True)}]],
{3: _DimHint(type=<_DimHintType.DYNAMIC: 3>,
min=None,
max=None,
_factory=True)}),
{})
And finally the export.
The export is simple if transformers>=4.50
, otherwise,
transformers needs to be patched.
onnx_diagnostic.torch_export_patches.bypass_export_some_errors()
registers functions to serialize DynamicCache
. This one is modified to make
the shape inference implemented in torch happy.
if has_transformers("4.50"):
ep = torch.export.export(model, inputs[0], dynamic_shapes=ds[0], strict=False)
else:
with bypass_export_some_errors(patch_transformers=True) as modificator:
ep = torch.export.export(
model, modificator(inputs[0]), dynamic_shapes=ds[0], strict=False
)
print(ep)
# Do we need to guess?
# ++++++++++++++++++++
#
# Function :func:`onnx_diagnostic.helpers.string_type` is using
# the serialization functions to print out the DynamicCache the was
# :func:`torch.export.export` expects them.
print(string_type(cache, with_shape=True))
ExportedProgram:
class GraphModule(torch.nn.Module):
def forward(self, cache_key_cache_0: "f32[s26, 4, s28, s1]", cache_key_cache_1: "f32[s26, 4, s28, s1]", cache_value_cache_0: "f32[s26, 4, s28, s1]", cache_value_cache_1: "f32[s26, 4, s28, s1]", z: "f32[1, 1, 1, s1]"):
# File: ~/github/onnx-diagnostic/_doc/examples/plot_export_with_dynamic_cache.py:39 in forward, code: z
add: "f32[s26, 4, s28, s1]" = torch.ops.aten.add.Tensor(z, cache_key_cache_0); z = cache_key_cache_0 = None
add_1: "f32[s26, 4, s28, s1]" = torch.ops.aten.add.Tensor(add, cache_key_cache_1); add = cache_key_cache_1 = None
add_2: "f32[s26, 4, s28, s1]" = torch.ops.aten.add.Tensor(add_1, cache_value_cache_0); add_1 = cache_value_cache_0 = None
add_3: "f32[s26, 4, s28, s1]" = torch.ops.aten.add.Tensor(add_2, cache_value_cache_1); add_2 = cache_value_cache_1 = None
return (add_3,)
Graph signature:
# inputs
cache_key_cache_0: USER_INPUT
cache_key_cache_1: USER_INPUT
cache_value_cache_0: USER_INPUT
cache_value_cache_1: USER_INPUT
z: USER_INPUT
# outputs
add_3: USER_OUTPUT
Range constraints: {s26: VR[2, int_oo], s28: VR[2, int_oo], s1: VR[2, int_oo]}
DynamicCache(key_cache=#2[T1s2x4x3x7,T1s2x4x3x7], value_cache=#2[T1s2x4x3x7,T1s2x4x3x7])
You can also use function
onnx_diagnostic.helpers.cache_helper.flatten_unflatten_for_dynamic_shapes()
to show a DynamicCache restructured the way torch.export.export()
expects
it to be without the custom class.
print(string_type(flatten_unflatten_for_dynamic_shapes(cache), with_shape=True))
#2[#2[T1s2x4x3x7,T1s2x4x3x7],#2[T1s2x4x3x7,T1s2x4x3x7]]
This code works for any custom class if it was registered
with torch.utils._pytree.register_pytree_node()
.
doc.plot_legend("dynamic shapes\nfor DynamicCache", "torch.export.export", "tomato")
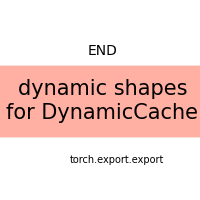
Total running time of the script: (0 minutes 0.210 seconds)
Related examples
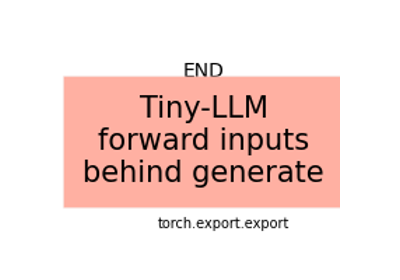
Steel method forward to guess the dynamic shapes (with Tiny-LLM)